Signed Graph Metric Learning via Gershgorin Disc Perfect Alignment
IEEE Transactions on Pattern Analysis and Machine Intelligence(2022)
摘要
Given a convex and differentiable objective
$Q({\mathbf M})$
for a real symmetric matrix
${\mathbf M}$
in the positive definite (PD) cone—used to compute Mahalanobis distances—we propose a fast general metric learning framework that is entirely projection-free. We first assume that
${\mathbf M}$
resides in a space
${\mathcal S}$
of generalized graph Laplacian matrices corresponding to balanced signed graphs.
${\mathbf M}\in {\mathcal S}$
that is also PD is called a graph metric matrix. Unlike low-rank metric matrices common in the literature,
${\mathcal S}$
includes the important diagonal-only matrices as a special case. The key theorem to circumvent full eigen-decomposition and enable fast metric matrix optimization is Gershgorin disc perfect alignment (GDPA): given
${\mathbf M}\in {\mathcal S}$
and diagonal matrix
${\mathbf S}$
, where
$S_{ii} = 1/v_i$
and
${\mathbf v}$
is the first eigenvector of
${\mathbf M}$
, we prove that Gershgorin disc left-ends of similarity transform
${\mathbf B}= {\mathbf S}{\mathbf M}{\mathbf S}^{-1}$
are perfectly aligned at the smallest eigenvalue
$\lambda _{\min }$
. Using this theorem, we replace the PD cone constraint in the metric learning problem with tightest possible linear constraints per iteration, so that the alternating optimization of the diagonal / off-diagonal terms in
${\mathbf M}$
can be solved efficiently as linear programs via the Frank-Wolfe method. We update
${\mathbf v}$
using Locally Optimal Block Preconditioned Conjugate Gradient (LOBPCG) with warm start as entries in
${\mathbf M}$
are optimized successively. Experiments show that our graph metric optimization is significantly faster than cone-projection schemes, and produces competitive binary classification performance.
更多查看译文
关键词
Graph signal processing,metric learning,Gershgorin circle theorem,convex optimization
AI 理解论文
溯源树
样例
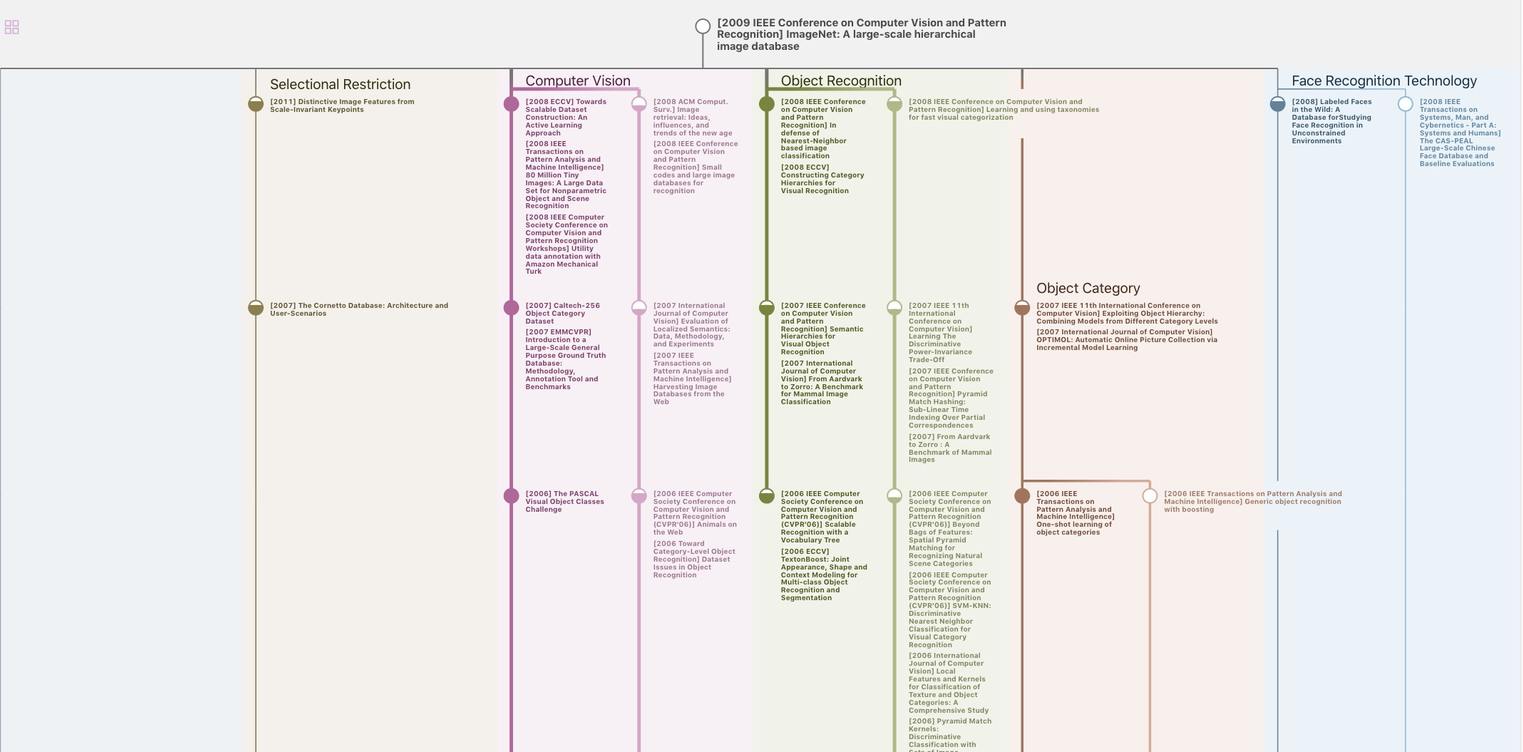
生成溯源树,研究论文发展脉络
Chat Paper
正在生成论文摘要