A Truss-Based Approach For Densest Homogeneous Subgraph Mining In Node-Attributed Graphs
COMPUTATIONAL INTELLIGENCE(2021)
摘要
In a wide range of graph analysis tasks such as community detection and event detection, densest subgraph mining is important and primitive. With the development of social network, densest subgraph mining not only need to consider the structural data but also the attributes information, which descripts the features of nodes or edges. However, there are few researches on densest subgraph mining with attribute description. In this article, we only focus on the node-attributed graph. According to the properties of structure and attribute in node-attributed graphs, we define a novel dense subgraph pattern, called hybridized k-truss in attribute-augmented graph. A hybridized k-truss is a subgraph that consists of structural nodes and attribute nodes, of which there are at least (k - 2) common neighbors between any two connected nodes. We introduce the densest hybridized truss problem, and the densest hybridized truss mapping to a densely connected subgraph with homogenous attributes in the original graph. We propose a densest hybridized truss extraction (DHTE) algorithm for node-attributed graphs, to automatically find the densest subgraph with high density and homogenous attributes at the same time. Extensive experimental results of 21 real world datasets demonstrate the effectiveness and efficiency of DHTE over state-of-the-art methods, through comparison about structural cohesiveness and attributive homogeneity.
更多查看译文
关键词
attribute description, densest subgraph, hybridized k‐, truss, Jaccard support, node‐, attributed graph
AI 理解论文
溯源树
样例
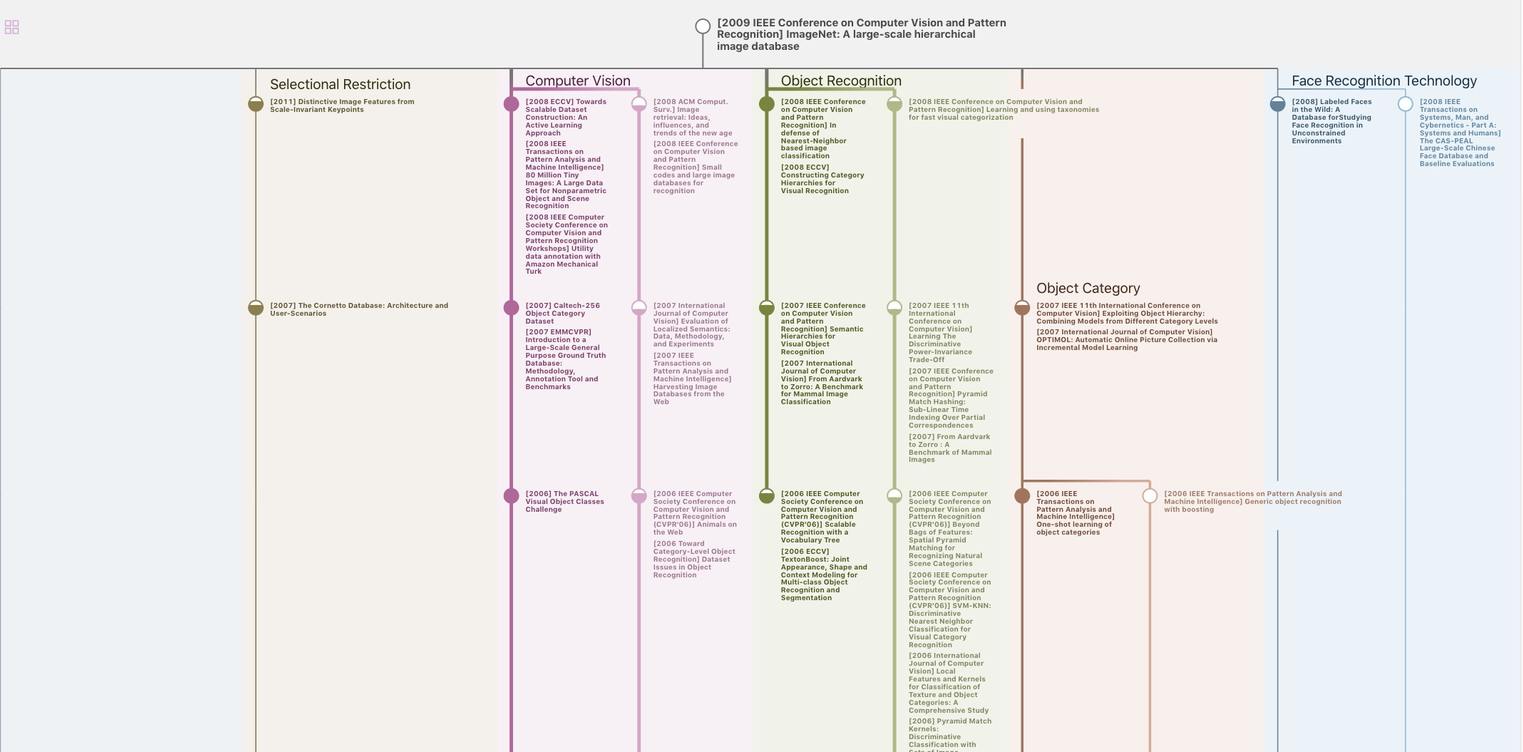
生成溯源树,研究论文发展脉络
Chat Paper
正在生成论文摘要