Using Document Embeddings for Background Linking of News Articles.
NLDB(2021)
摘要
This paper describes our experiments in using document embeddings to provide background links to news articles. This work was done as part of the recent TREC 2020 News Track [26] whose goal is to provide a ranked list of related news articles from a large collection, given a query article. For our participation, we explored a variety of document embedding representations and proximity measures. Experiments with the 2018 and 2019 validation sets showed that GPT2 and XLNet embeddings lead to higher performances. In addition, regardless of the embedding, higher performances were reached when mean pooling, larger models and smaller token chunks are used. However, no embedding configuration alone led to a performance that matched the classic Okapi BM25 method. For our official TREC 2020 News Track submission, we therefore combined the BM25 model with an embedding method. The augmented model led to more diverse sets of related articles with minimal decrease in performance (nDCG@5 of 0.5873 versus 0.5924 with the vanilla BM25). This result is promising as diversity is a key factor used by journalists when providing background links and contextual information to news articles [27].
更多查看译文
关键词
Background linking,Document embedding,Proximity measures
AI 理解论文
溯源树
样例
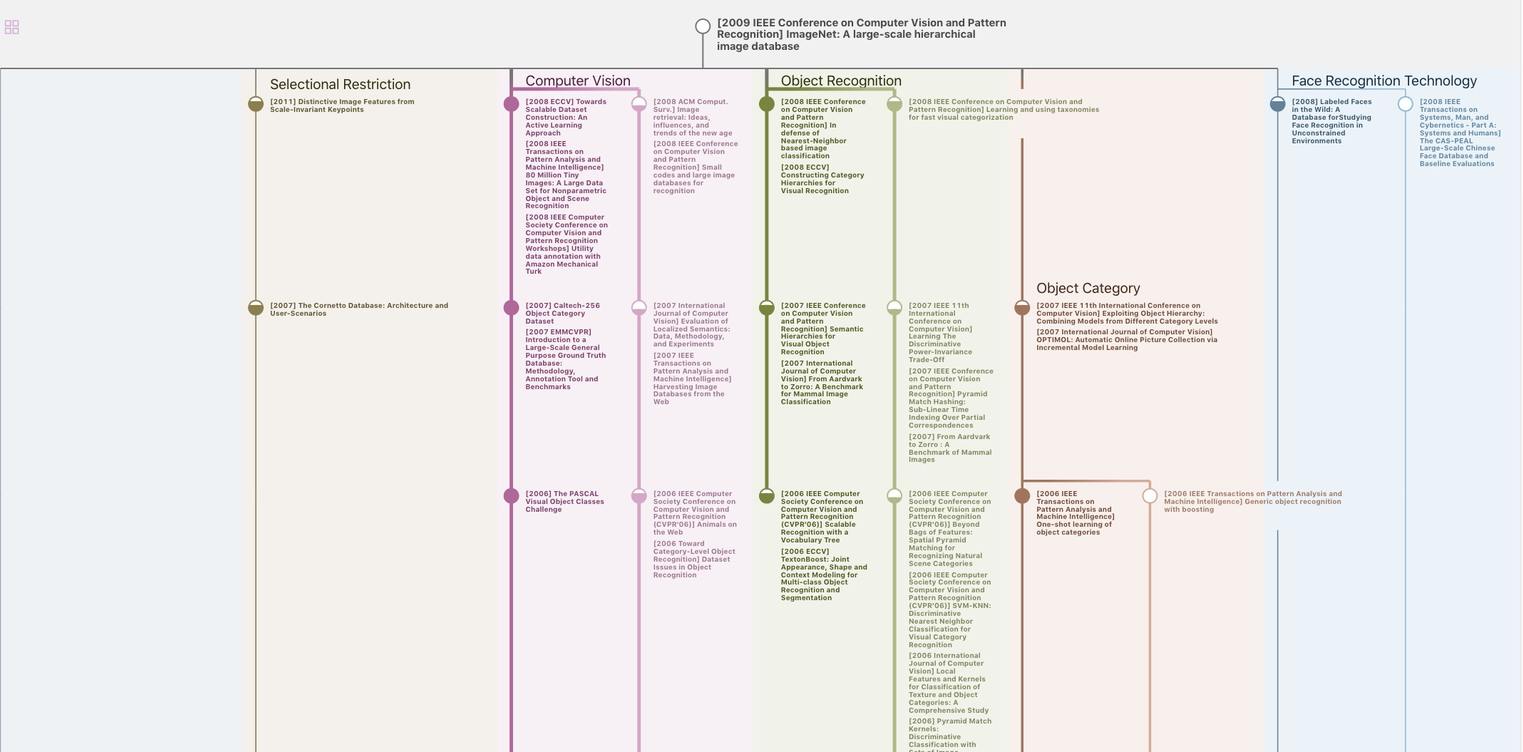
生成溯源树,研究论文发展脉络
Chat Paper
正在生成论文摘要