Two-Level K-Nearest Neighbors Approach For Invasive Plants Detection And Classification
APPLIED SOFT COMPUTING(2021)
摘要
Invasive plants (IPs) are considered major threat to biodiversity and ecosystems due to their potential to compete for resources with other plants or crops. Timely and effective detection is important to prevent and control their growth. Recent advancements in unmanned aerial systems (UAS) have brought an effective, inexpensive, and non-invasive approach to monitoring plants. The use of UAS is promising in the accurate and rapid identification of IPs in the field. However, challenges exist when using UAS for IP detection as they generate a huge number of images that must be interpreted by researchers manually. This time-consuming and error-prone process significantly limits the applicability of UAS in a variety of environmental and agriculture monitoring applications. To reduce processing time and increase detection accuracy, we develop an automatic and intelligent detection approach to identify IPs with high levels of efficiency and accuracy from images of the monitoring areas captured by UAS. First, the original field images are segmented into patch images using the simple linear iterative clustering (SLIC) superpixels algorithm. Then a novel two-level K-nearest neighbors (TLKNN) algorithm is proposed to classify the patch images into different IP categories. In it, a deep convolutional neural network (CNN) is used to extract the features from the patch image and a kernel map function is utilized to obtain the kernel features. Two feature sets are fed to train two K-nearest neighbor (KNN) models. Finally, the final classification is determined by the selection results by KNN models. In the experiments, two public UAV image sets are employed to train and verify the proposed method. Several metrics such as accuracy, recall, precision, and F1-score, are used to evaluate the performance. The proposed TLKNN is compared with two newly proposed convolutional neural network (CNN) methods on the same datasets. Experimental results indicate the proposed TLKNN algorithm achieves better classification performance and reaches the highest accuracy, recall rate, precision rate, and F1-score. The improved performance not only means that IPs can be detected more accurately and automatically, but also that there are environmental benefits in developing an intelligent strategy for IP prevention and control. (C) 2021 Elsevier B.V. All rights reserved.
更多查看译文
关键词
Deep learning, K-nearest neighbors, Image classification, Unmanned aerial system, Invasive plant detection
AI 理解论文
溯源树
样例
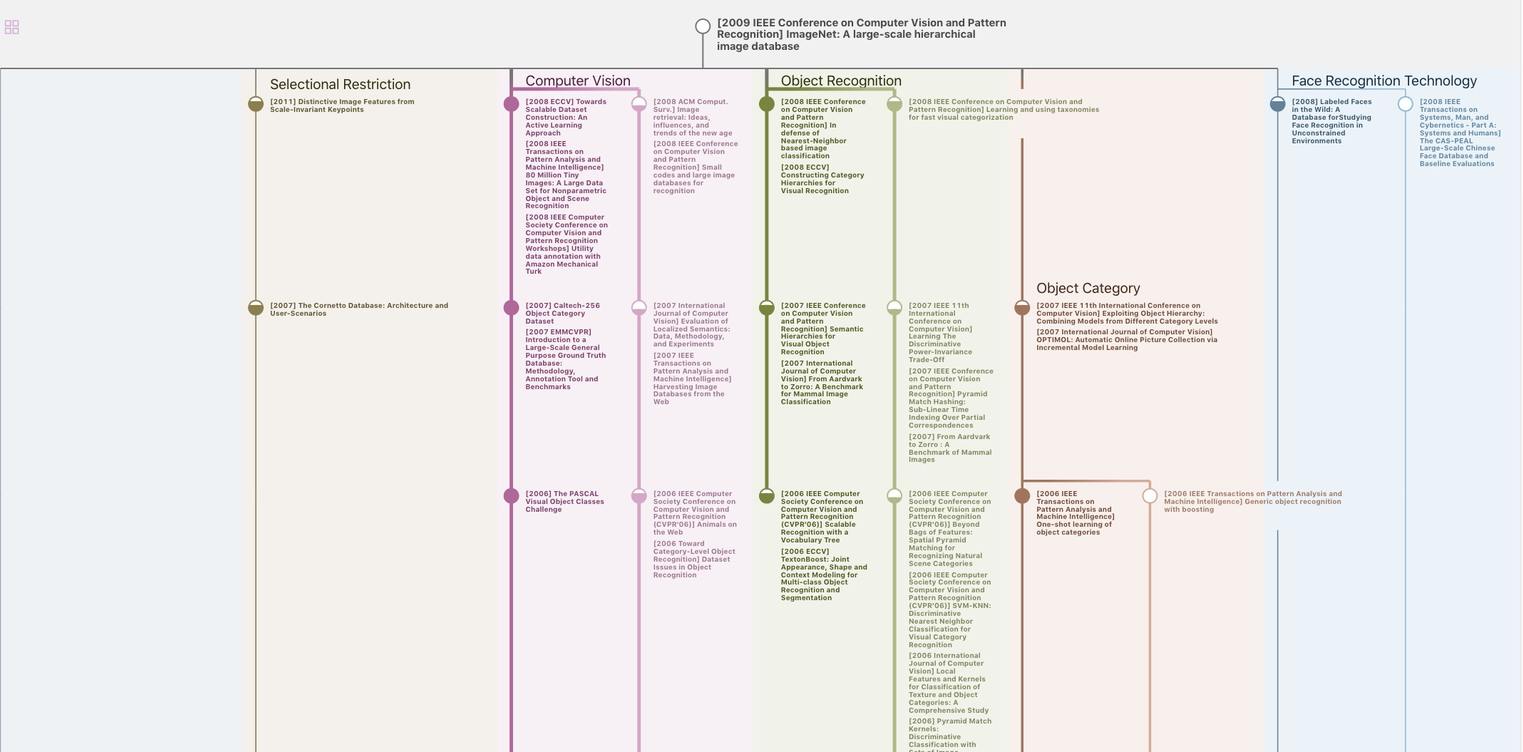
生成溯源树,研究论文发展脉络
Chat Paper
正在生成论文摘要