Structural Generalizability: The Case of Similarity Search
International Conference on Management of Data(2021)
摘要
ABSTRACTSupervised and Unsupervised ML algorithms are widely used over graphs. They use the structural properties of the data to deliver effective results. It is known that the same information can be represented under various graph structures. Thus, these algorithms may be effective on some structural variations of the data and ineffective on others. One would like to have an algorithm that is effective and generalizes to all structural variations of a data graph. We define the concept of structural generalizability for algorithms over graphs. We focus on the problem of similarity search, which is a popular task and the building block of many ML algorithms on graphs, and propose a structurally generalizable similarity search algorithm. As this algorithm may require users to specify features in a rather complex language, we modify this algorithm so that it requires only simple guidance from the user. Our extensive empirical study show that our algorithms are structurally generalizable while being efficient and more effective than current algorithms.
更多查看译文
关键词
Structural Variations, Structural Generalizability, Similarity Search
AI 理解论文
溯源树
样例
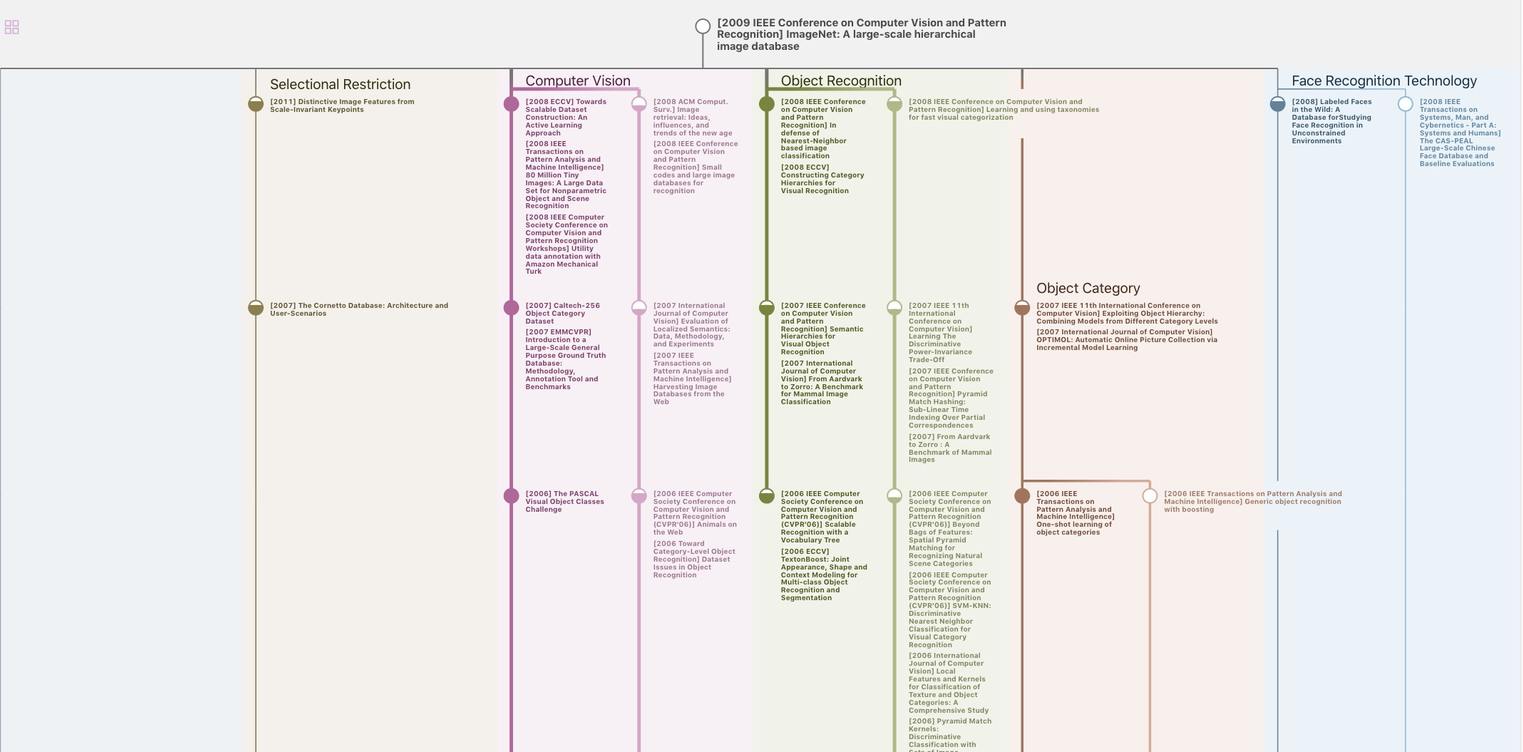
生成溯源树,研究论文发展脉络
Chat Paper
正在生成论文摘要