Kaizen: Continuously Improving Teacher Using Exponential Moving Average for Semi-Supervised Speech Recognition
2021 IEEE Automatic Speech Recognition and Understanding Workshop (ASRU)(2021)
摘要
In this paper, we introduce the Kaizen framework that uses a continuously improving teacher to generate pseudo-labels for semi-supervised speech recognition (ASR). The proposed approach uses a teacher model which is updated as the exponential moving average (EMA) of the student model parameters. We demonstrate that it is critical for EMA to be accumulated with full-precision floating point. The Kaizen framework can be seen as a continuous version of the iterative pseudo-labeling approach for semi-supervised training. It is applicable for different training criteria, and in this paper we demonstrate its effectiveness for frame-level hybrid hidden Markov model-deep neural network (HMM-DNN) systems as well as sequence-level Connectionist Temporal Classification (CTC) based models. For large scale real-world unsupervised public videos in UK English and Italian languages the proposed approach i) shows more than 10% relative word error rate (WER) reduction over standard teacher-student training; ii) using just 10 hours of supervised data and a large amount of unsupervised data closes the gap to the upper-bound supervised ASR system that uses 650h or 2700h respectively.
更多查看译文
关键词
speech recognition,semi-supervised training,pseudo-labeling,low-resource,teacher-student
AI 理解论文
溯源树
样例
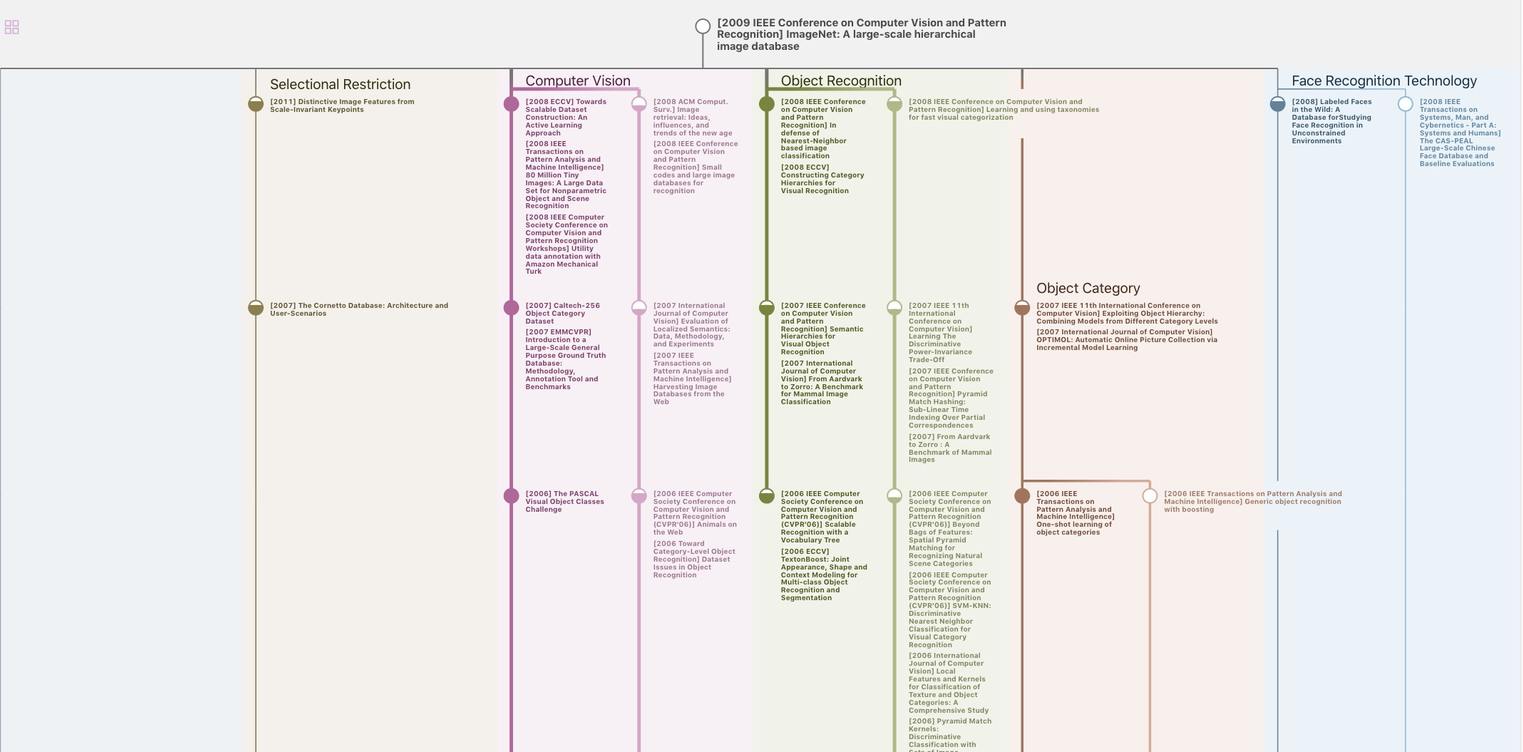
生成溯源树,研究论文发展脉络
Chat Paper
正在生成论文摘要