EmoDNN: understanding emotions from short texts through a deep neural network ensemble
Neural Computing and Applications(2023)
摘要
The knowledge obtained from emotions via online communities is substantially valuable in various domains, including social management, resource planning, politics, and market predictions. Affective computing, as a multi-aspect realm, aims to exploit emotion-pertinent details from various contents via connecting artificial intelligence to cognitive science. The hidden personality cues in daily brief contents can reveal the cognitive aspect of authors and uncover both similarities and contrasts between them. However, the main challenge lies in devising a cognition-aware algorithm to trace emotional cues in brief contents. To solve the challenge, we develop a novel framework that infers the cognitive aspect of individuals. We propose a deep ensemble method, supplied with a novel dropout algorithm, that aggregates outcomes from various classifiers to extract emotions from short texts. We employ a new embedding approach to enrich emotion-relevant features, collectively assembled via lexicons and attention actuates, resulting in a preferable set of vectors. The experimental results show that our proposed framework can achieve better accuracy in recognizing emotions versus other trending competitors. We empirically observe that detecting emotion latent cues via relying on personality features can effectively distinguish short text authors. Furthermore, the deep learning models overcome conventional methods, including the SVM, categorization, and heuristic rules.
更多查看译文
关键词
Neural network architecture,Cognitive factors,Emotion recognition,Ensemble learning
AI 理解论文
溯源树
样例
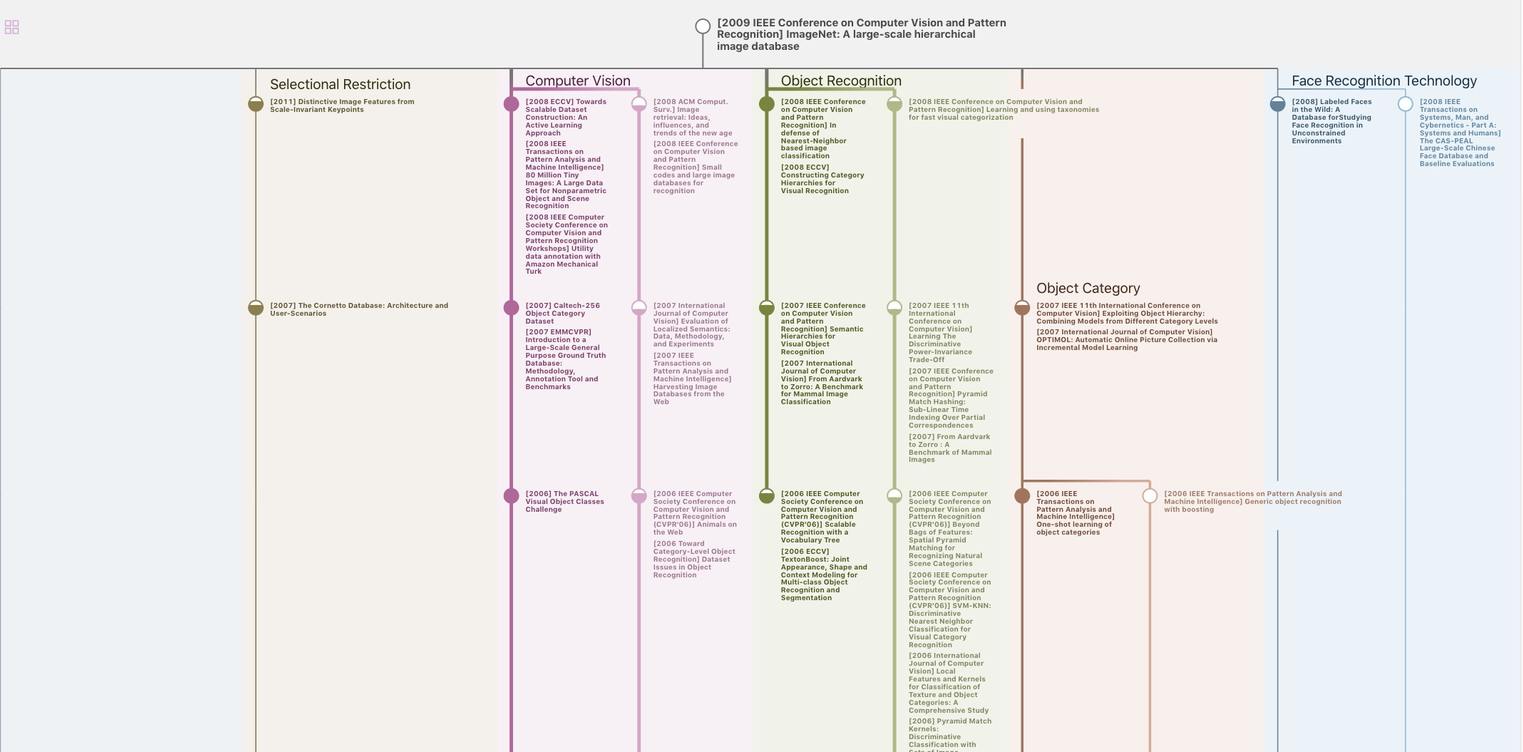
生成溯源树,研究论文发展脉络
Chat Paper
正在生成论文摘要