Physics-Guided Discovery of Highly Nonlinear Parametric Partial Differential Equations
KDD '23 Proceedings of the 29th ACM SIGKDD Conference on Knowledge Discovery and Data Mining(2023)
Abstract
Partial differential equations (PDEs) fitting scientific data can represent physical laws with explainable mechanisms for various mathematically-oriented subjects. The data-driven discovery of PDEs from scientific data thrives as a new attempt to model complex phenomena in nature, but the effectiveness of current practice is typically limited by the scarcity of data and the complexity of phenomena. Especially, the discovery of PDEs with highly nonlinear coefficients from low-quality data remains largely under-addressed. To deal with this challenge, we propose a novel physics-guided learning method, which can not only encode observation knowledge such as initial and boundary conditions but also incorporate the basic physical principles and laws to guide the model optimization. We empirically demonstrate that the proposed method is more robust against data noise and sparsity, and can reduce the estimation error by a large margin; moreover, for the first time we are able to discover PDEs with highly nonlinear coefficients. With the promising performance, the proposed method pushes forward the boundary of the PDEs that can be found by machine learning models for scientific discovery.
MoreTranslated text
Key words
highly nonlinear parametric partial
AI Read Science
Must-Reading Tree
Example
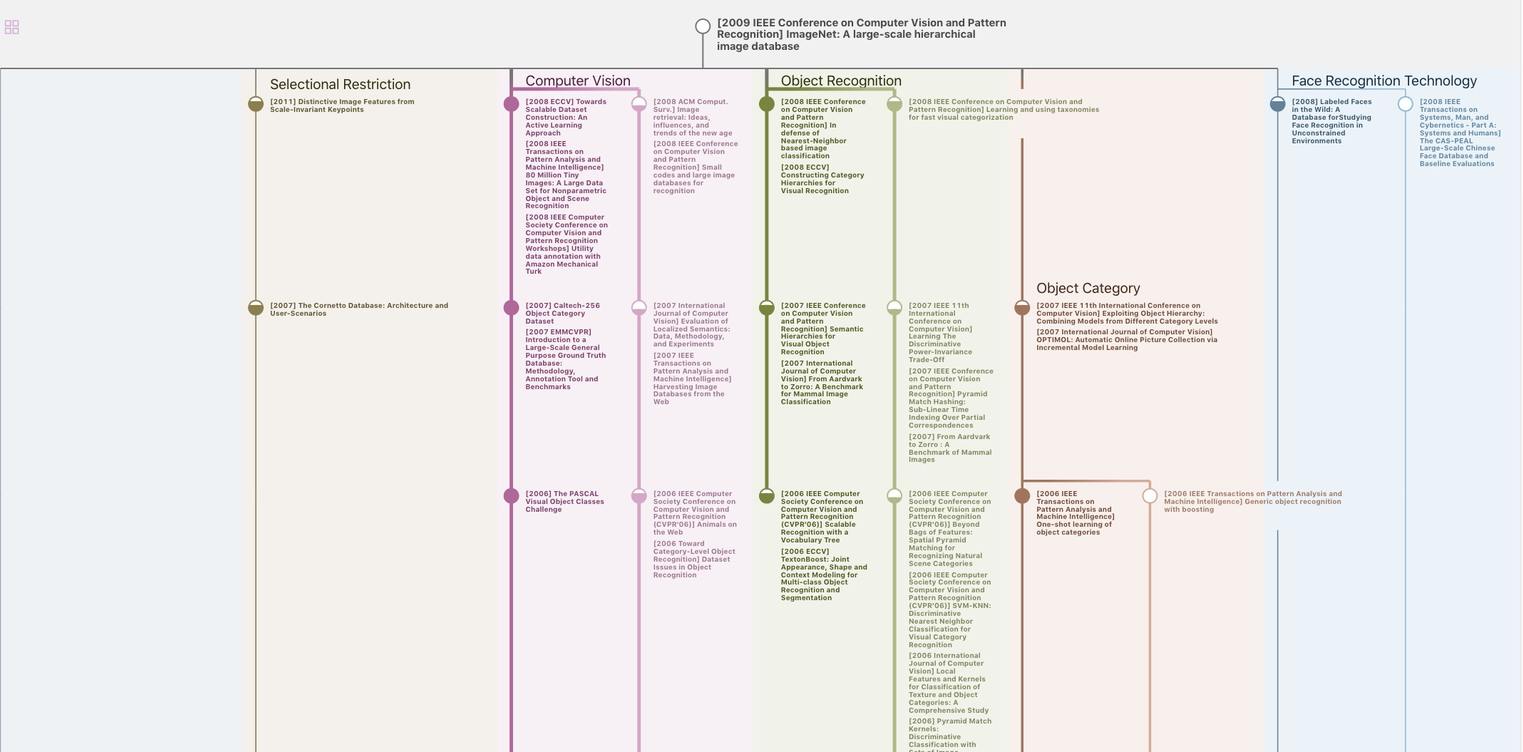
Generate MRT to find the research sequence of this paper
Chat Paper
Summary is being generated by the instructions you defined