Coordinated Energy Management For A Cluster Of Buildings Through Deep Reinforcement Learning
ENERGY(2021)
摘要
Advanced control strategies can enable energy flexibility in buildings by enhancing on-site renewable energy exploitation and storage operation, significantly reducing both energy costs and emissions. However, when the energy management is faced shifting from a single building to a cluster of buildings, uncoordinated strategies may have negative effects on the grid reliability, causing undesirable new peaks. To overcome these limitations, the paper explores the opportunity to enhance energy flexibility of a cluster of buildings, taking advantage from the mutual collaboration between single buildings by pursuing a coordinated approach in energy management. This is achieved using Deep Reinforcement Learning (DRL), an adaptive model-free control algorithm, employed to manage the thermal storages of a cluster of four buildings equipped with different energy systems. The controller was designed to flatten the cluster load profile while optimizing energy consumption of each building. The coordinated energy management controller is tested and compared against a manually optimised rule-based one. Results shows a reduction of operational costs of about 4%, together with a decrease of peak demand up to 12%. Furthermore, the control strategy allows to reduce the average daily peak and average peak-to average ratio by 10 and 6% respectively, highlighting the benefits of a coordinated approach. (c) 2021 Elsevier Ltd. All rights reserved.
更多查看译文
关键词
Coordinated energy management, Deep reinforcement learning, Building energy flexibility, Peak demand reduction, Grid interaction
AI 理解论文
溯源树
样例
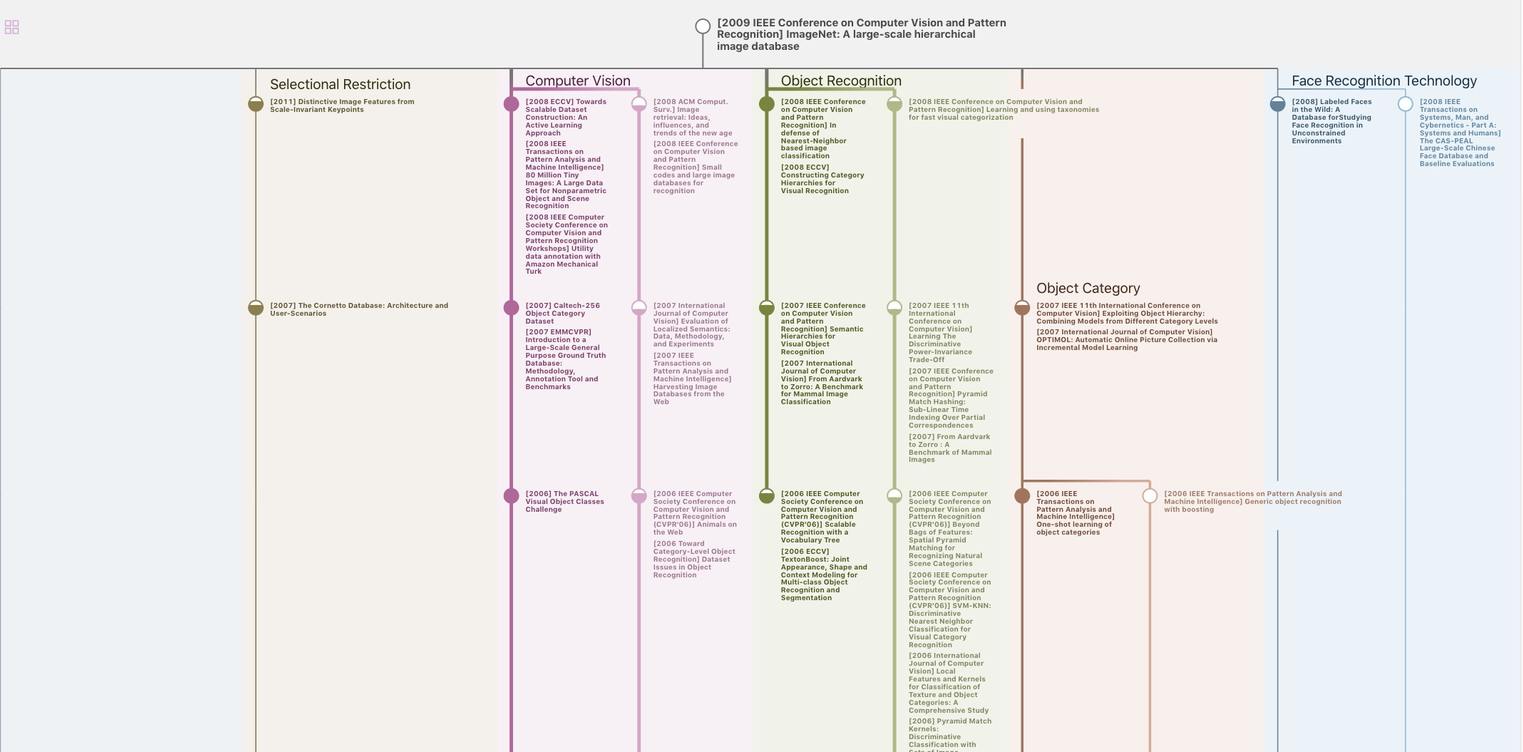
生成溯源树,研究论文发展脉络
Chat Paper
正在生成论文摘要