A Deep Motion Deblurring Network Using Channel Adaptive Residual Module
IEEE ACCESS(2021)
摘要
In this paper, we solve the problem of dynamic scenes deblurring with motion blur. Restoration of images in the presence of motion blur necessitates a network design that the receptive field can completely cover all areas that need to be deblurred, while the existing network increases the receptive field by continuously stacking the ordinary convolutional layer or increasing the size of the convolution kernel. However, these methods inevitably increase the computational burden of the network. We propose a novel architecture consisting of a channel adaptive residual module. Different features of the blurred image are extracted and distributed on each feature channel. Our network can calculate the weight of each channel through learning, and extract the image features adaptively according to different degrees of blurring and importance of information. We embed the module in a modified encoder-decoder design with skip connections to achieve multi-scale feature fusion for further performance improvement. The extensive comparison with the existing techniques in the baseline dynamic scene deblurring dataset shows that the proposed network can effectively realize image deblurring, and the accuracy and speed are comparable with the existing techniques.
更多查看译文
关键词
Generators, Generative adversarial networks, Kernel, Feature extraction, Convolution, Automobiles, Image restoration, Channel feature, generative adversarial network, motion deblurring
AI 理解论文
溯源树
样例
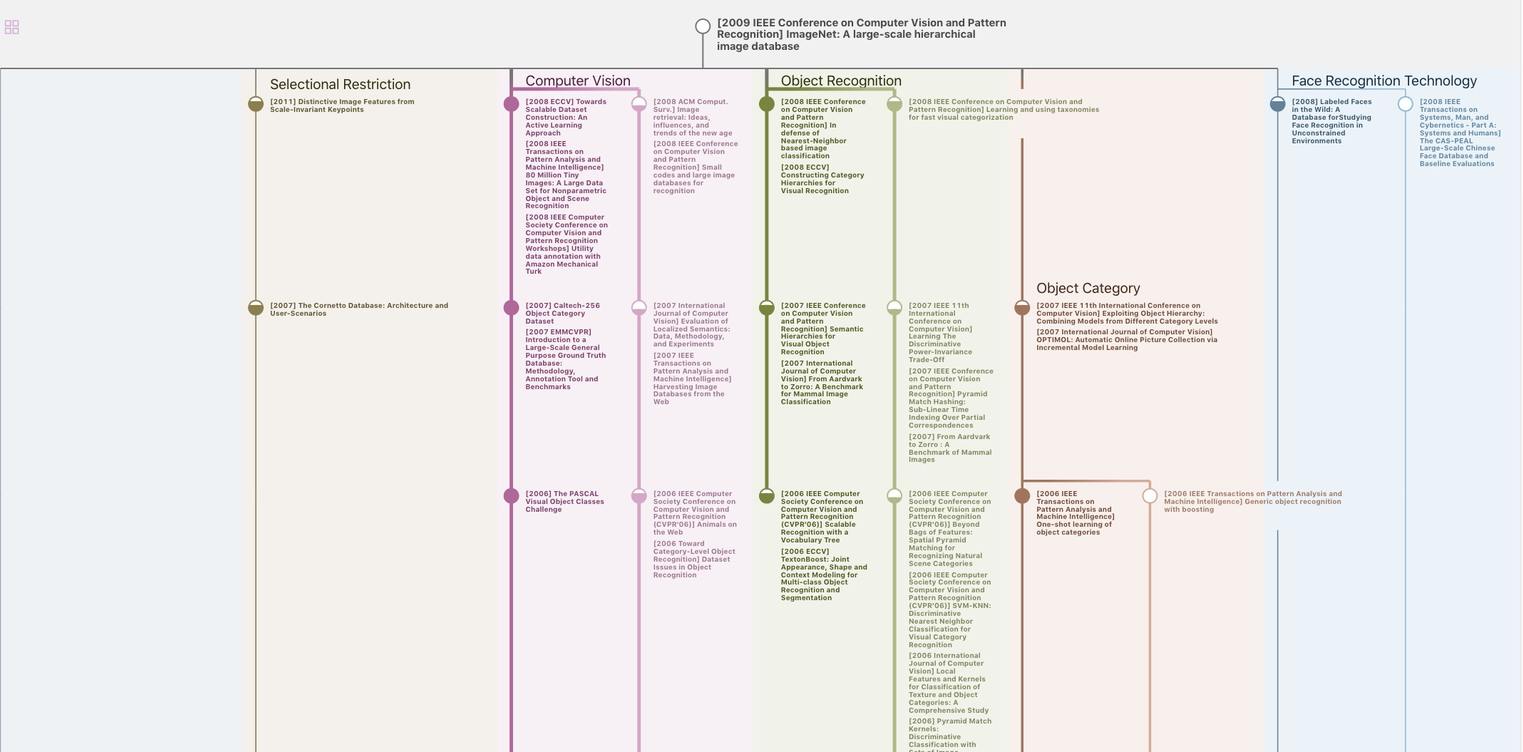
生成溯源树,研究论文发展脉络
Chat Paper
正在生成论文摘要