Individualized Degradation Modeling and Prognostics in a Heterogeneous Group via Incorporating Intrinsic Covariate Information
IEEE Transactions on Automation Science and Engineering(2022)
摘要
This article focuses on individualized degradation modeling and prognostics for a heterogeneous group, where each individual unit shows a distinct degradation process. Existing degradation models usually treat each unit separately and do not fully utilize the distinct characteristics of each individual. In this study, we propose a generic framework to handle the heterogeneity across units by effectively leveraging the intrinsic covariate information, which is closely related to the unit’s degradation process. Specifically, we employ a multivariate Gaussian process (MGP) to nonparametrically establish the relation between the covariate information and degradation process. Through modeling the unit similarities based on the covariates, efficient information transfer among units is enabled for better degradation modeling and prognostics, as the collected degradation signals from one unit can be shared with the entire heterogeneous group. A theoretical justification for the proposed model is also investigated. Simulation studies are presented to evaluate the parameter estimation accuracy and the sensitivity of the proposed method. A case study on the Alzheimer’s disease (AD) neuroimaging initiative data set is further conducted, which demonstrates the advantage of the proposed method over existing benchmark approaches.
Note to Practitioners
—This article is motivated by the practical issue of degradation modeling and prognostics for a heterogeneous group, where all units in a group share some similarities and each unit has its own distinct individual-level characteristics (covariates). The covariates in this study refer to static intrinsic characteristics instead of dynamic external environmental conditions. Several practical examples are explained in
Section I
with more details. There are two fundamental questions involved: 1) how to quantify the distinct individual characteristics of each unit while representing the group-level commonalities among all units and 2) how to perform degradation modeling and prognostics of a newly launched unit with few degradation signals available. The novelty of this article lies in encoding the available knowledge about individual covariates and group-level commonalities into the degradation modeling and prognostics. There are three main steps involved when implementing the proposed method: 1) collecting degradation signals, failure time, and covariate information of heterogeneous units; 2) constructing an MGP-based degradation model; and 3) predicting the degradation status and remaining useful life of the in-service units based on their covariates and signals. The proposed method is particularly useful when we collect various intrinsic covariates which can effectively represent the individual-level characteristics and when only sparse or no data are available for the units of interest.
更多查看译文
关键词
Multivariate Gaussian process (MGP),predictive analytics,remaining useful lifetime (RUL) distribution,transfer learning
AI 理解论文
溯源树
样例
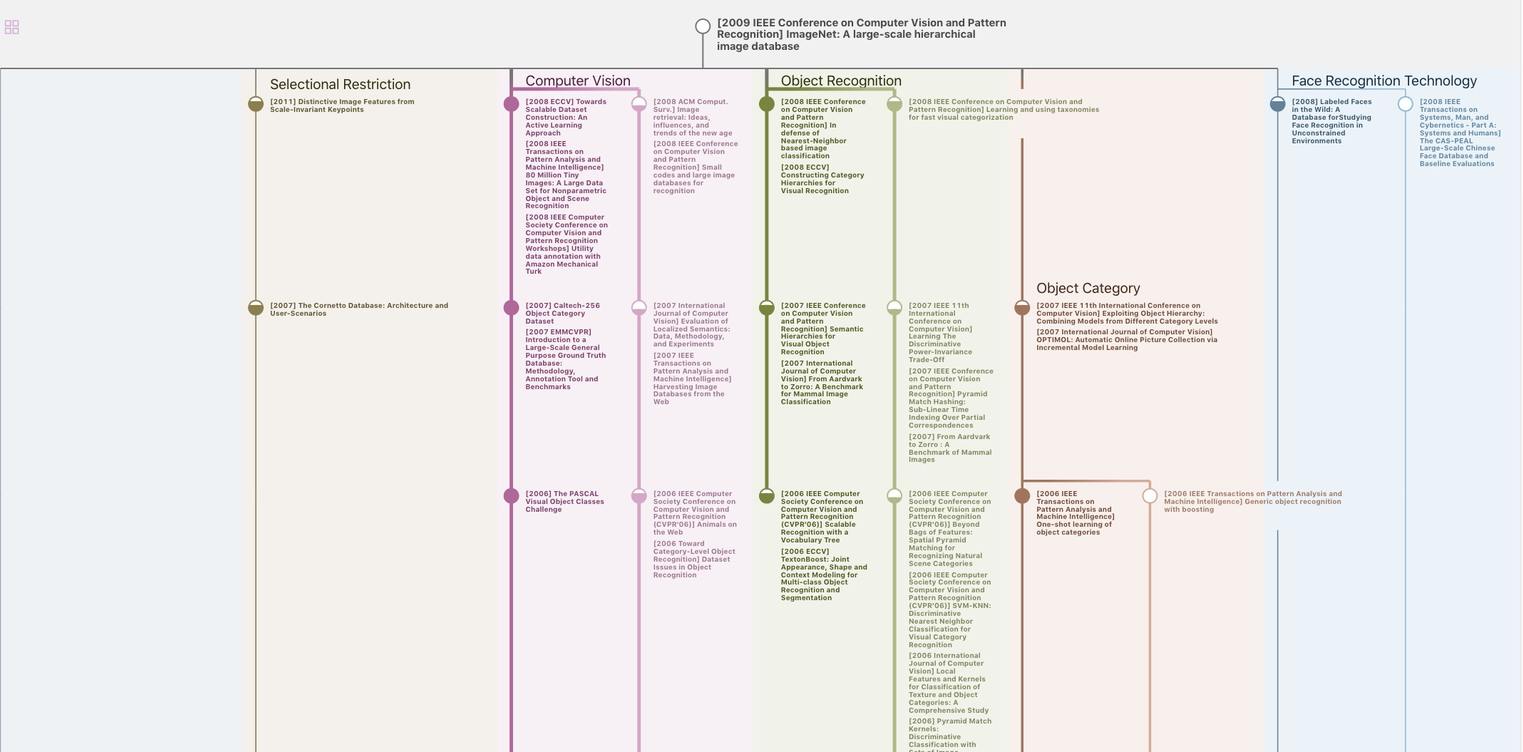
生成溯源树,研究论文发展脉络
Chat Paper
正在生成论文摘要