Non-Volatile In-Ga-Zn-O Transistors for Neuromorphic Computing
APPLIED PHYSICS A-MATERIALS SCIENCE & PROCESSING(2021)
Abstract
Hardware neural networks (HNNs) can be constructed by placing synaptic devices in a crossbar array, and these systems may overcome the inherent inefficiency of conventional computing systems. In this work, non-volatile In-Ga-Zn-O transistors were designed for use in HNNs. The In-Ga-Zn-O transistors consist of a naturally oxidized nanothick Al 2 O 3 /ion gel stacking dielectric, in which the gate electric field induces positive charge trapping effect could pull the subthreshold swing down to the value of 32.9 mV/decade, which is much lower than the theoretical limit of 60 mV/decade at room temperature. A highly repeatable and robust hysteresis behavior was observed in the transfer curve of the devices. These three-terminal In-Ga-Zn-O transistors could implement important memristive synaptic functions, including non-volatile memory, synaptic plasticity, and long-term potentiation/depression. A simulated HNN built from these In-Ga-Zn-O transistors with long-term potentiation/depression and optimized amplification factor exhibits 84% recognition accurate of handwritten data. The work illustrates an important application of energy-efficient HNNs by using high-performance non-volatile neuromorphic devices.
MoreTranslated text
Key words
In-Ga-Zn-O transistors, Charge trapping, Non-volatile memory, Steep subthreshold swing, Hardware neural networks
AI Read Science
Must-Reading Tree
Example
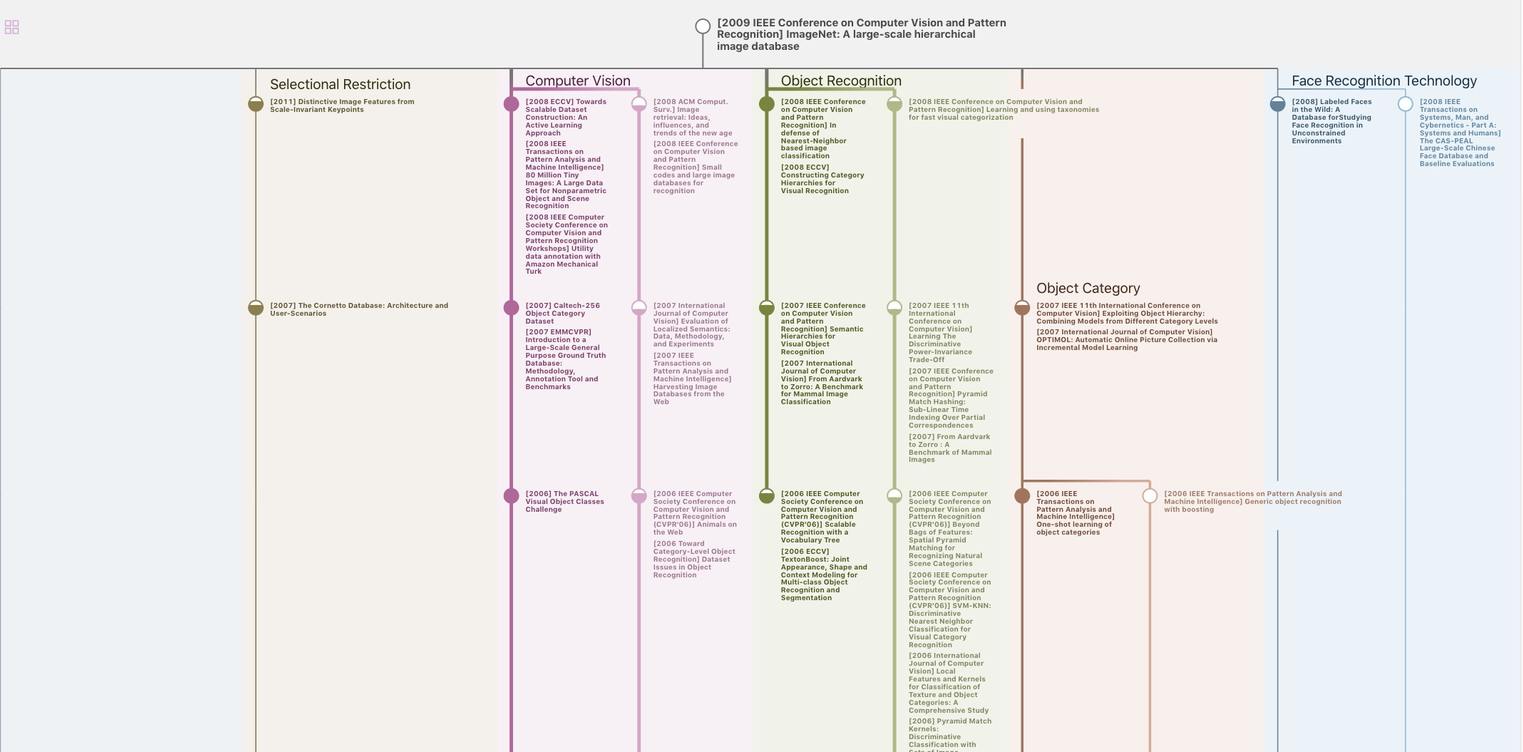
Generate MRT to find the research sequence of this paper
Chat Paper
Summary is being generated by the instructions you defined