Continual 3D Convolutional Neural Networks for Real-time Processing of Videos.
European Conference on Computer Vision(2022)
摘要
We introduce Continual 3D Convolutional Neural Networks (Co3D CNNs), a new computational formulation of spatio-temporal 3D CNNs, in which videos are processed frame-by-frame rather than by clip. In online tasks demanding frame-wise predictions, Co3D CNNs dispense with the computational redundancies of regular 3D CNNs, namely the repeated convolutions over frames, which appear in overlapping clips. We show that Continual 3D CNNs can reuse preexisting 3D-CNN weights to reduce the per-prediction floating point operations (FLOPs) in proportion to the temporal receptive field while retaining similar memory requirements and accuracy. This is validated with multiple models on Kinetics-400 and Charades with remarkable results: CoX3D models attain state-of-the-art complexity/accuracy trade-offs on Kinetics-400 with 12.1−15.3\(\times \) reductions of FLOPs and 2.3−3.8% improvements in accuracy compared to regular X3D models while reducing peak memory consumption by up to 48%. Moreover, we investigate the transient response of Co3D CNNs at start-up and perform extensive benchmarks of on-hardware processing characteristics for publicly available 3D CNNs.
更多查看译文
关键词
3D CNN,Human activity recognition,Efficient,Stream processing,Online inference,Continual inference network
AI 理解论文
溯源树
样例
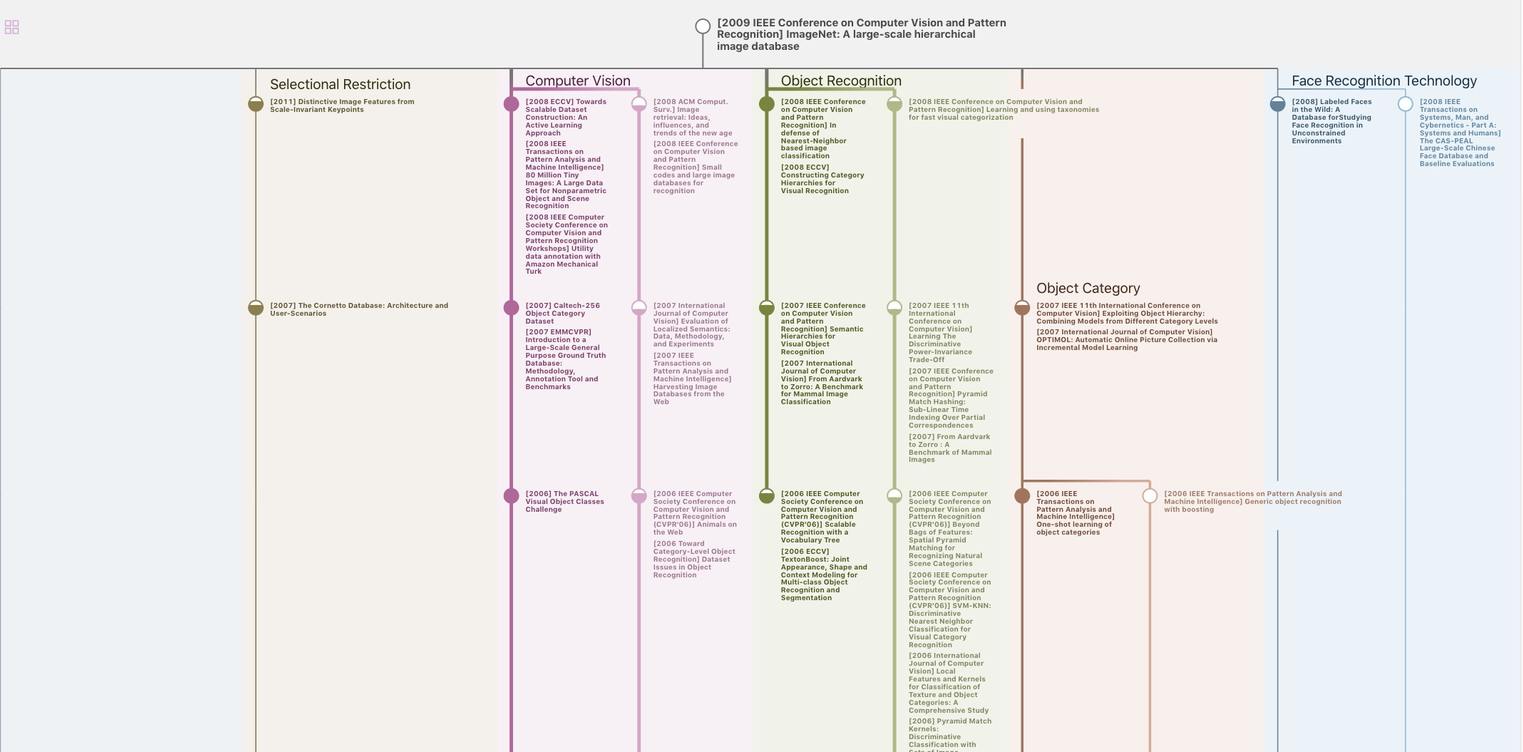
生成溯源树,研究论文发展脉络
Chat Paper
正在生成论文摘要