High-Dimensional Phase Space Reconstruction With A Convolutional Neural Network For Structural Health Monitoring
SENSORS(2021)
摘要
In order to accurately diagnose the health of high-order statically indeterminate structures, most existing structural health monitoring (SHM) methods require multiple sensors to collect enough information. However, comprehensive data collection from multiple sensors for high degree-of-freedom structures is not typically available in practice. We propose a method that reconciles the two seemingly conflicting difficulties. Takens' embedding theorem is used to augment the dimensions of data collected from a single sensor. Taking advantage of the success of machine learning in image classification, high-dimensional reconstructed attractors were converted into images and fed into a convolutional neural network (CNN). Attractor classification was performed for 10 damage cases of a 3-story shear frame structure. Numerical results show that the inherently high dimension of the CNN model allows the handling of higher dimensional data. Information on both the level and the location of damage was successfully embedded. The same methodology will allow the extraction of data with unsupervised CNN classification to be consistent with real use cases.
更多查看译文
关键词
convolutional neural network (CNN), structural health monitoring (SHM), Takens' embedding theorem, attractor reconstruction
AI 理解论文
溯源树
样例
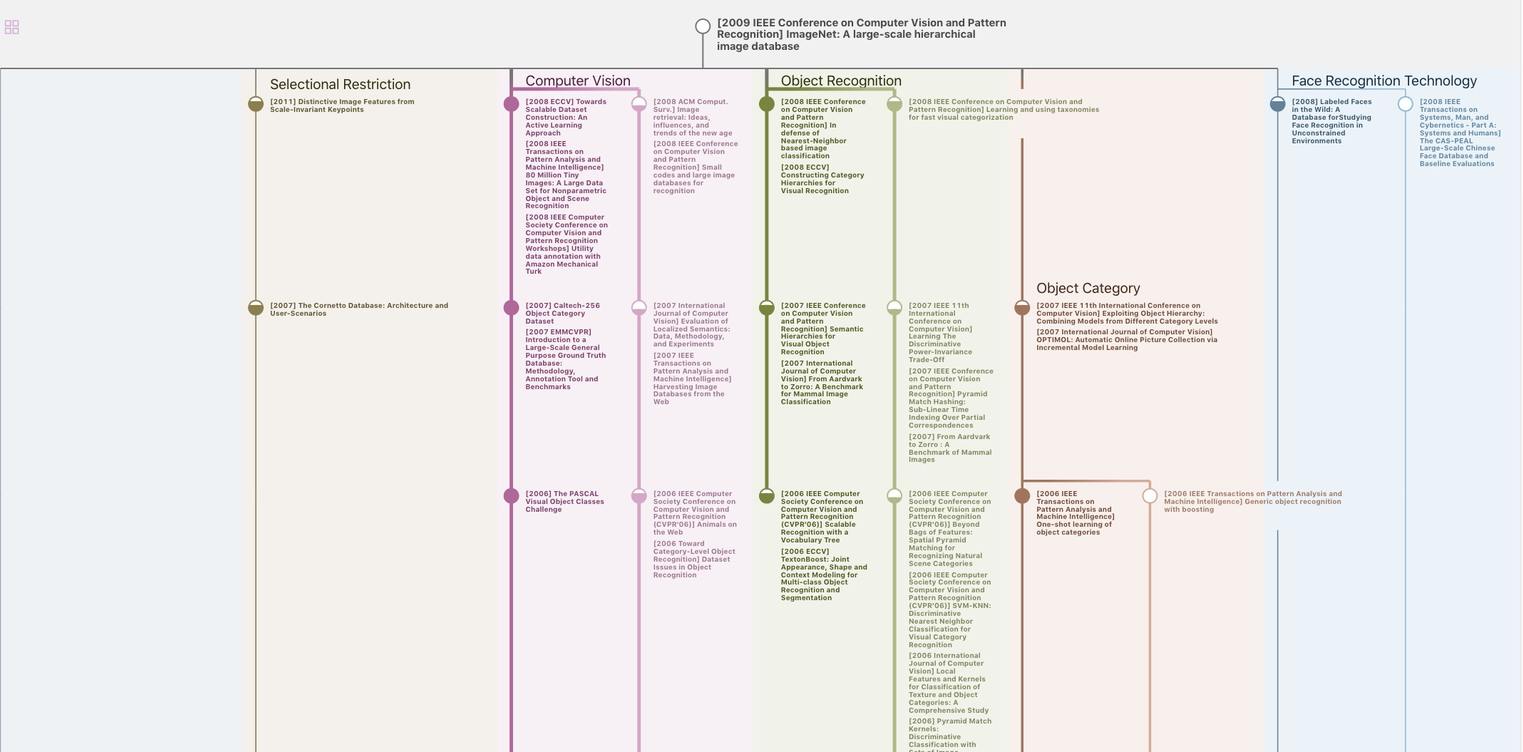
生成溯源树,研究论文发展脉络
Chat Paper
正在生成论文摘要