Genome-Scale Metabolic Modelling When Changes In Environmental Conditions Affect Biomass Composition
PLOS COMPUTATIONAL BIOLOGY(2021)
摘要
Genome-scale metabolic modeling is an important tool in the study of metabolism by enhancing the collation of knowledge, interpretation of data, and prediction of metabolic capabilities. A frequent assumption in the use of genome-scale models is that the in vivo organism is evolved for optimal growth, where growth is represented by flux through a biomass objective function (BOF). While the specific composition of the BOF is crucial, its formulation is often inherited from similar organisms due to the experimental challenges associated with its proper determination. A cell's macro-molecular composition is not fixed and it responds to changes in environmental conditions. As a consequence, initiatives for the high-fidelity determination of cellular biomass composition have been launched. Thus, there is a need for a mathematical and computational framework capable of using multiple measurements of cellular biomass composition in different environments. Here, we propose two different computational approaches for directly addressing this challenge: Biomass Trade-off Weighting (BTW) and Higher-dimensional-plane InterPolation (HIP). In lieu of experimental data on biomass composition-variation in response to changing nutrient environment, we assess the properties of BTW and HIP using three hypothetical, yet biologically plausible, BOFs for the Escherichia coli genome-scale metabolic model iML1515. We find that the BTW and HIP formulations have a significant impact on model performance and phenotypes. Furthermore, the BTW method generates larger growth rates in all environments when compared to HIP. Using acetate secretion and the respiratory quotient as proxies for phenotypic changes, we find marked differences between the methods as HIP generates BOFs more similar to a reference BOF than BTW. We conclude that the presented methods constitute a conceptual step in developing genome-scale metabolic modelling approaches capable of addressing the inherent dependence of cellular biomass composition on nutrient environments.Author summary Changes in the environment promote changes in an organism's metabolism. To achieve balanced growth states for near-optimal function, cells respond through metabolic rearrangements, which may influence the biosynthesis of metabolic precursors for building a cell's molecular constituents. Therefore, it is necessary to take the dependence of biomass composition on environmental conditions into consideration. While measuring the biomass composition for some environments is possible, and should be done, it cannot be completed for all possible environments. In this work, we propose two main approaches, BTW and HIP, for addressing the challenge of estimating biomass composition in response to environmental changes. We evaluate the phenotypic consequences of BTW and HIP by characterizing their effect on growth, secretion potential, respiratory efficiency, and gene essentiality of a cell. Our work constitutes a first conceptual step in accounting for the influence of growth conditions on biomass composition, and in turn the biomass composition's effect on metabolic phenotypic traits, within constraint-based modelling. As such, we believe it will improve the relevance of constraint-based methods in metabolic engineering and drug discovery, since the biosynthetic potential of microbes for generating industrially relevant products or drugs often is closely linked to their biomass composition.
更多查看译文
关键词
biomass composition,genome-scale
AI 理解论文
溯源树
样例
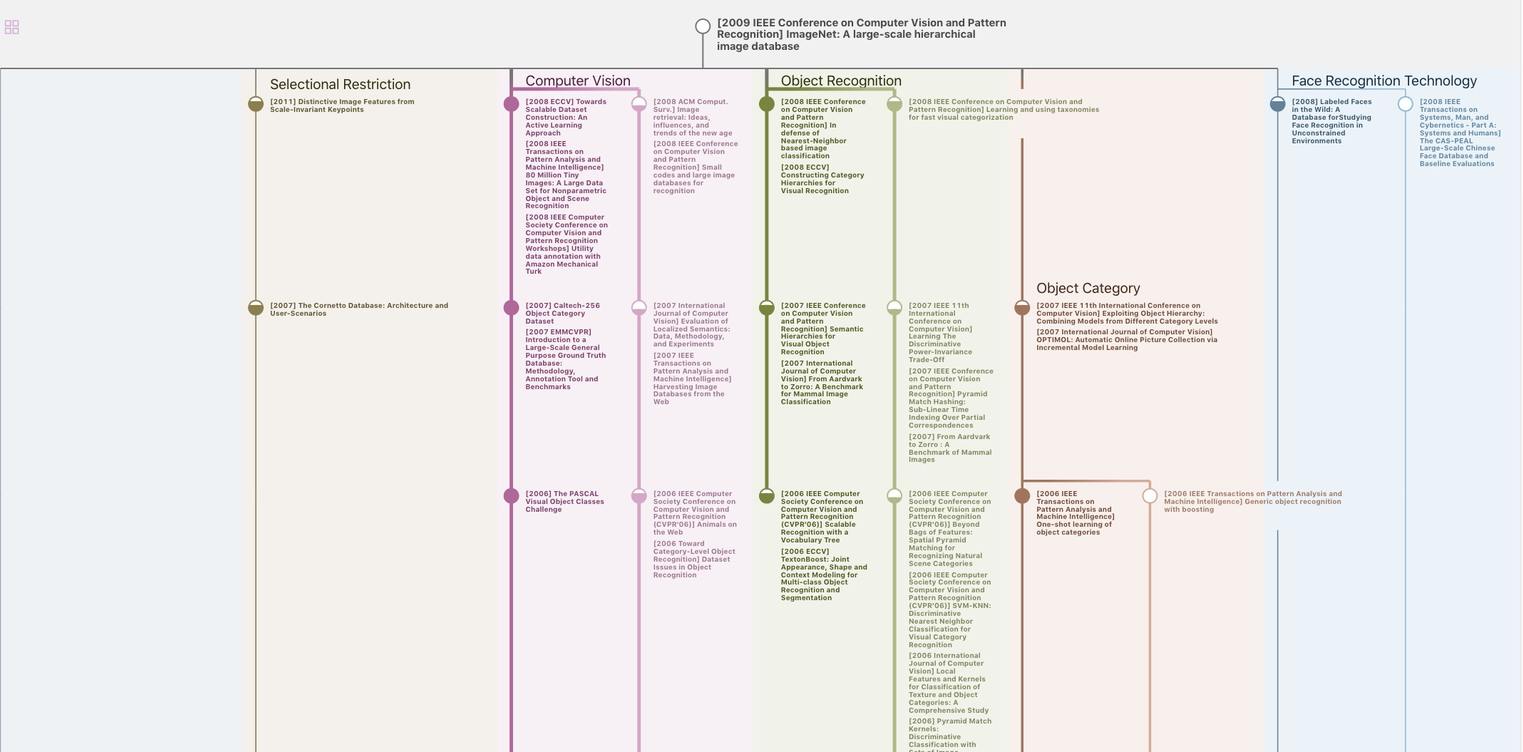
生成溯源树,研究论文发展脉络
Chat Paper
正在生成论文摘要