Deep Learning And Phenology Enhance Large-Scale Tree Species Classification In Aerial Imagery During A Biosecurity Response
REMOTE SENSING(2021)
摘要
The ability of deep convolutional neural networks (deep learning) to learn complex visual characteristics offers a new method to classify tree species using lower-cost data such as regional aerial RGB imagery. In this study, we use 10 cm resolution imagery and 4600 trees to develop a deep learning model to identify Metrosideros excelsa (pohutukawa)-a culturally important New Zealand tree that displays distinctive red flowers during summer and is under threat from the invasive pathogen Austropuccinia psidii (myrtle rust). Our objectives were to compare the accuracy of deep learning models that could learn the distinctive visual characteristics of the canopies with tree-based models (XGBoost) that used spectral and textural metrics. We tested whether the phenology of pohutukawa could be used to enhance classification by using multitemporal aerial imagery that showed the same trees with and without widespread flowering. The XGBoost model achieved an accuracy of 86.7% on the dataset with strong phenology (flowering). Without phenology, the accuracy fell to 79.4% and the model relied on the blueish hue and texture of the canopies. The deep learning model achieved 97.4% accuracy with 96.5% sensitivity and 98.3% specificity when leveraging phenology-even though the intensity of flowering varied substantially. Without strong phenology, the accuracy of the deep learning model remained high at 92.7% with sensitivity of 91.2% and specificity of 94.3% despite significant variation in the appearance of non-flowering pohutukawa. Pooling time-series imagery did not enhance either approach. The accuracy of XGBoost and deep learning models were, respectively, 83.2% and 95.2%, which were of intermediate precision between the separate models.
更多查看译文
关键词
tree species, classification, deep learning, convolutional networks, biosecurity, forest pathology, myrtle rust, urban forestry, machine learning, aerial imagery
AI 理解论文
溯源树
样例
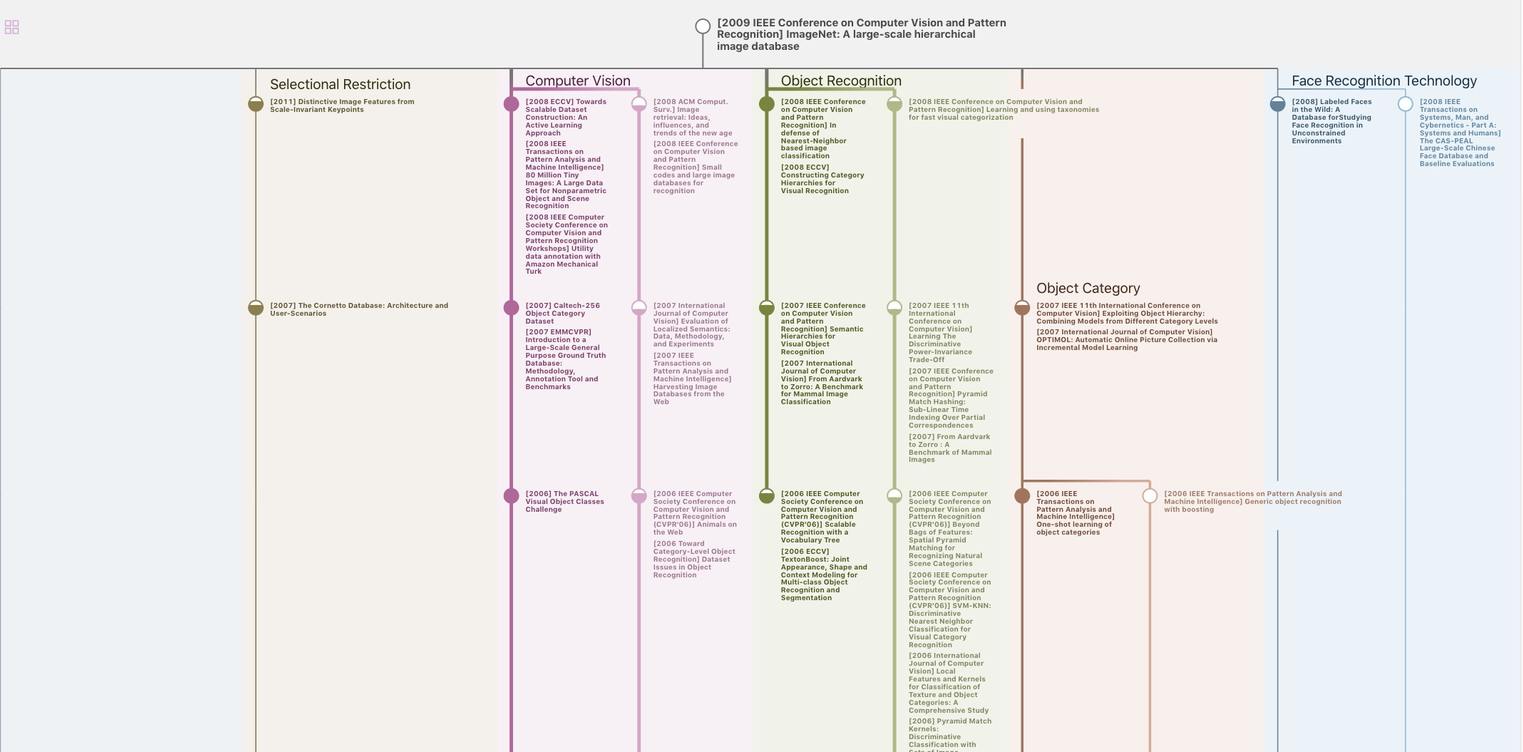
生成溯源树,研究论文发展脉络
Chat Paper
正在生成论文摘要