Neural Network Based Sleep Phases Classification for Resource Constraint Environments
SIU(2021)
摘要
Sleep is restoration process of the body. The efficiency of this restoration process is directly correlated to the amount of time spent at each sleep phase. Hence, automatic tracking of sleep via wearable devices has attracted both the researchers and industry. Current state-of-the-art sleep tracking solutions are memory and processing greedy and they require cloud or mobile phone connectivity. We propose a memory efficient sleep tracking architecture which can work in the embedded environment without needing any cloud or mobile phone connection. In this study, a novel architecture is proposed that consists of a feature extraction and Artificial Neural Networks based stacking classifier. Besides, we discussed how to tackle with sequential nature of the sleep staging for the memory constraint environments through the proposed framework. To verify the system, a dataset is collected from 24 different subjects for 31 nights with a wrist worn device having 3-axis accelerometer (ACC) and photoplethysmogram (PPG) sensors. Over the collected dataset, the proposed classification architecture achieves 20\% and 14\% better F1 scores than its competitors. Apart from the superior performance, proposed architecture is a promising solution for resource constraint embedded systems by allocating only 4.2 kilobytes of memory (RAM).
更多查看译文
关键词
Heart Rate,Heart Rate Variability,Sleep Phase Classification,Artificial Neural Networks,Wearable Devices
AI 理解论文
溯源树
样例
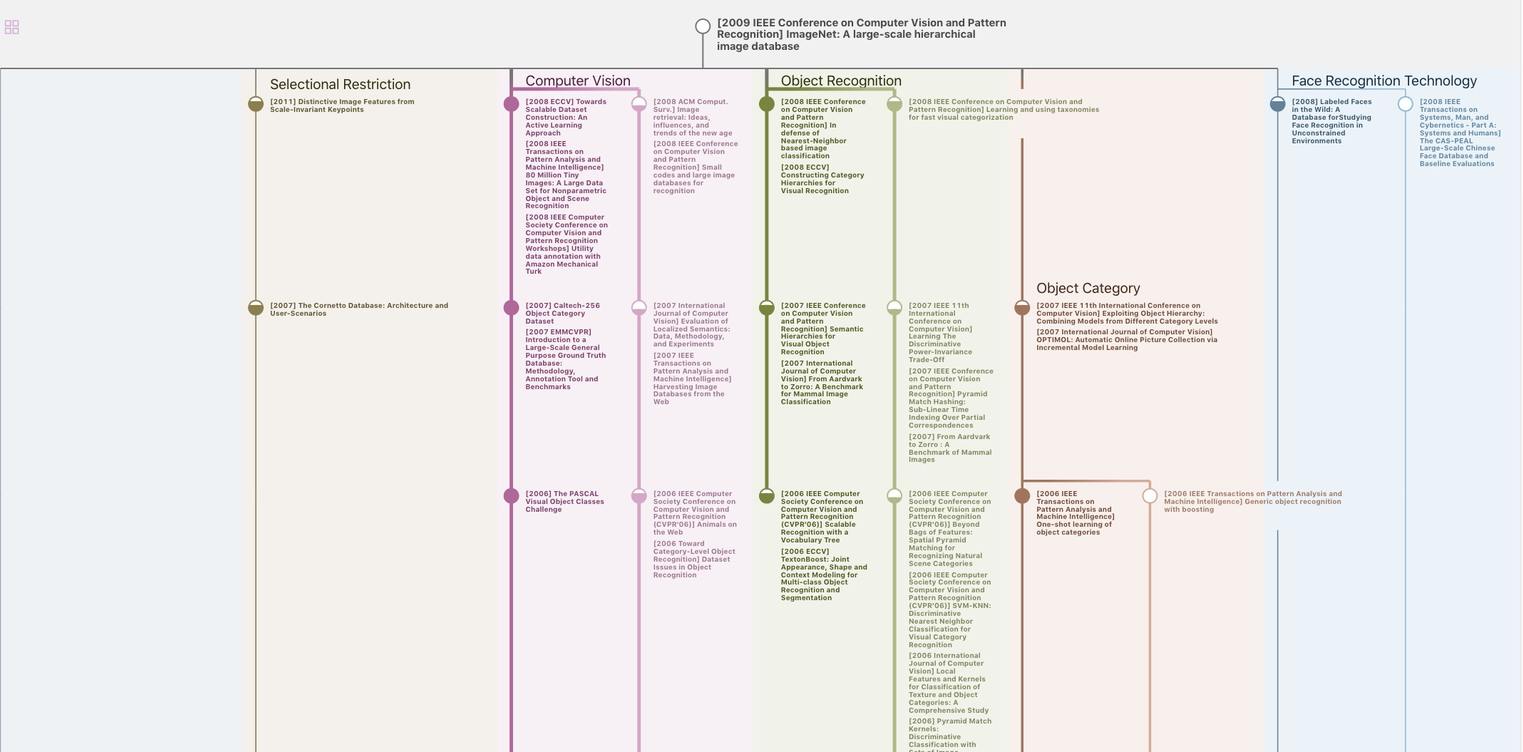
生成溯源树,研究论文发展脉络
Chat Paper
正在生成论文摘要