Efficient learning with augmented spikes: A case study with image classification
Neural Networks(2021)
摘要
Efficient learning of spikes plays a valuable role in training spiking neural networks (SNNs) to have desired responses to input stimuli. However, current learning rules are limited to a binary form of spikes. The seemingly ubiquitous phenomenon of burst in nervous systems suggests a new way to carry more information with spike bursts in addition to times. Based on this, we introduce an advanced form, the augmented spikes, where spike coefficients are used to carry additional information. How could neurons learn and benefit from augmented spikes remains unclear. In this paper, we propose two new efficient learning rules to process spatiotemporal patterns composed of augmented spikes. Moreover, we examine the learning abilities of our methods with a synthetic recognition task of augmented spike patterns and two practical ones for image classification. Experimental results demonstrate that our rules are capable of extracting information carried by both the timing and coefficient of spikes. Our proposed approaches achieve remarkable performance and good robustness under various noise conditions, as compared to benchmarks. The improved performance indicates the merits of augmented spikes and our learning rules, which could be beneficial and generalized to a broad range of spike-based platforms.
更多查看译文
关键词
Augmented spikes,Multi-spike learning,Image classification,Spiking neural networks,Temporal encoding,Neuromorphic computing
AI 理解论文
溯源树
样例
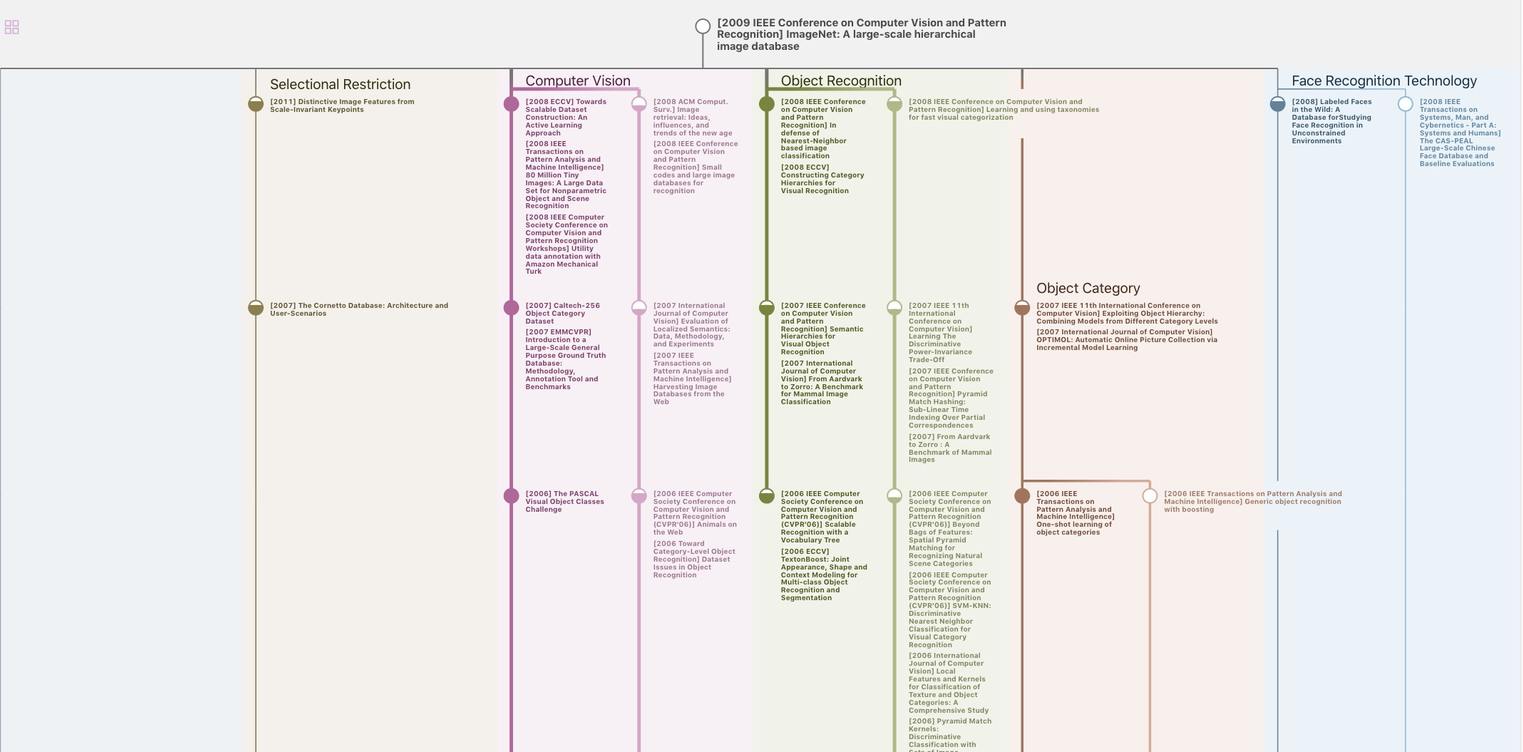
生成溯源树,研究论文发展脉络
Chat Paper
正在生成论文摘要