An Efficient Training Approach for Very Large Scale Face Recognition
arxiv(2022)
摘要
Face recognition has achieved significant progress in deep learning era due to the ultra-large-scale and well- labeled datasets. However, training on the outsize datasets is time-consuming and takes up a lot of hardware resource. Therefore, designing an efficient training approach is in- dispensable. The heavy computational and memory costs mainly result from the million-level dimensionality of the fully connected (FC) layer. To this end, we propose a novel training approach, termed Faster Face Classification (F
2
C), to alleviate time and cost without sacrificing the performance. This method adopts Dynamic Class Pool (DCP) for storing and updating the identities' features dy-namically, which could be regarded as a substitute for the FC layer. DCP is efficiently time-saving and cost-saving, as its smaller size with the independence from the whole face identities together. We further validate the proposed F
2
C method across several face benchmarks and private datasets, and display comparable results, meanwhile the speed is faster than state-of-the-art FC-based methods in terms of recognition accuracy and hardware costs. More-over, our method is further improved by a well-designed dual data loader including indentity-based and instance- based loaders, which makes it more efficient for updating DCP parameters.
更多查看译文
关键词
Face and gestures
AI 理解论文
溯源树
样例
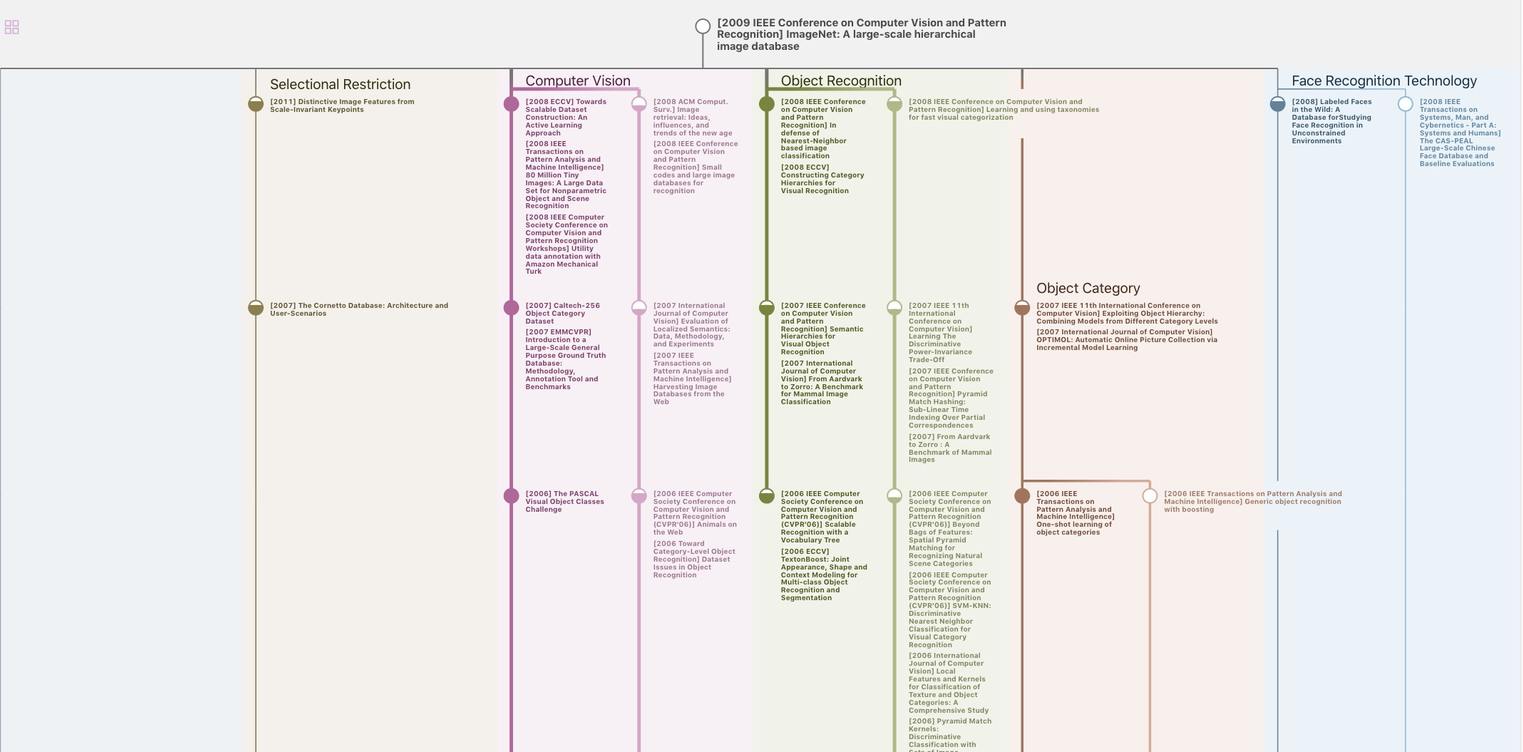
生成溯源树,研究论文发展脉络
Chat Paper
正在生成论文摘要