Training of computational algorithms to predict NAFLD activity score and fibrosis stage from liver histopathology slides
Computer Methods and Programs in Biomedicine(2021)
摘要
Background: The incidence of non-alcoholic fatty liver disease (NAFLD) and its progressive form, nonalcoholic steatohepatitis (NASH), has been increasing for decades. Since the mainstay is lifestyle modification in this mainly asymptomatic condition, there is a need for accurate diagnostic methods. Objectives: This study proposes a method with a computer-aided diagnosis (CAD) system to predict NAFLD Activity score (NAS scores-steatosis, lobular inflammation, and ballooning) and fibrosis stage from histopathology slides. Methods: A total of 87 pathology slides pairs (H&E and Trichrome-stained) were used for the study. Ground-truth NAS scores and fibrosis stages were previously identified by a pathologist. Each slide was split into 224 x 224 patches and fed into a feature extraction network to generate local features. These local features were processed and aggregated to obtain a global feature to predict the slide's scores. The effects of different training strategies, as well as training data with different staining and magnifications were explored. Four-fold cross validation was performed due to the small data size. Area Under the Receiver Operating Curve (AUROC) was utilized to evaluate the prediction performance of the machine learning algorithm. Results: Predictive accuracy for all subscores was high in comparison with pathologist assessment. There was no difference among the 3 magnifications (5x, 10x, 20x) for NAS-steatosis and fibrosis stage tasks. A larger magnification (20x) achieved better performance for NAS-lobular scores. Middle-level magnification was best for NAS-ballooning task. Trichrome slides are better for fibrosis stage prediction and NAS-ballooning score prediction task. NAS-steatosis prediction had the best performance (AUC 90.48%) in the model. A good performance was observed with fibrosis stage prediction (AUC 83.85%) as well as NAS-ballooning prediction (AUC 81.06%). Conclusions: These results were robust. The method proposed proved to be effective in predicting NAFLD Activity score and fibrosis stage from histopathology slides. The algorithms are an aid in having an accurate and systematic diagnosis in a condition that affects hundreds of millions of patients globally. (c) 2021 Published by Elsevier B.V.
更多查看译文
关键词
Machine learning,Computer-aided diagnosis systems,NAFLD,NASH,NAS score,Fibrosis stage
AI 理解论文
溯源树
样例
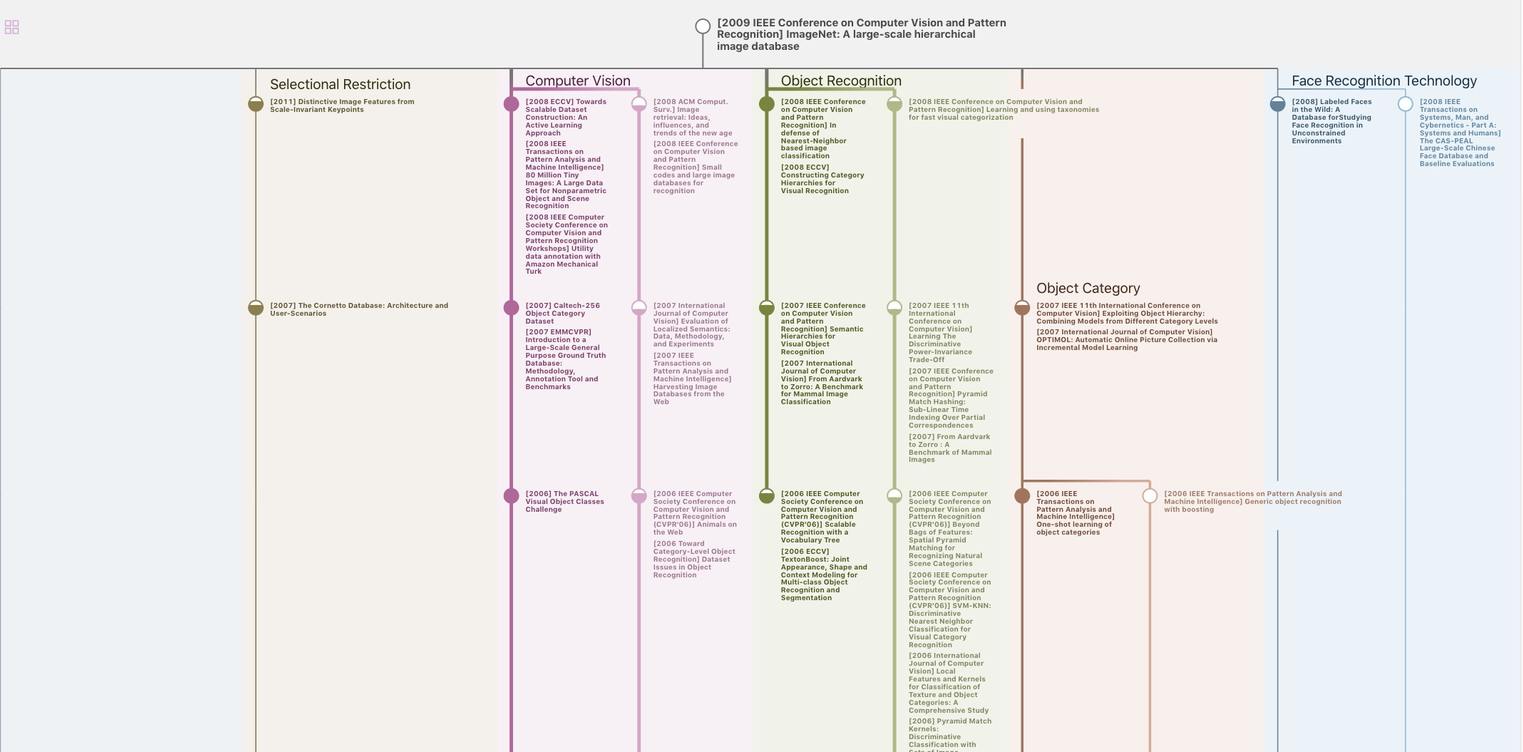
生成溯源树,研究论文发展脉络
Chat Paper
正在生成论文摘要