A Hybrid Model for Named Entity Recognition on Chinese Electronic Medical Records
ACM Transactions on Asian and Low-Resource Language Information Processing(2021)
摘要
AbstractElectronic medical records (EMRs) contain valuable information about the patients, such as clinical symptoms, diagnostic results, and medications. Named entity recognition (NER) aims to recognize entities from unstructured text, which is the initial step toward the semantic understanding of the EMRs. Extracting medical information from Chinese EMRs could be a more complicated task because of the difference between English and Chinese. Some researchers have noticed the importance of Chinese NER and used the recurrent neural network or convolutional neural network (CNN) to deal with this task. However, it is interesting to know whether the performance could be improved if the advantages of the RNN and CNN can be both utilized. Moreover, RoBERTa-WWM, as a pre-training model, can generate the embeddings with word-level features, which is more suitable for Chinese NER compared with Word2Vec. In this article, we propose a hybrid model. This model first obtains the entities identified by bidirectional long short-term memory and CNN, respectively, and then uses two hybrid strategies to output the final results relying on these entities. We also conduct experiments on raw medical records from real hospitals. This dataset is provided by the China Conference on Knowledge Graph and Semantic Computing in 2019 (CCKS 2019). Results demonstrate that the hybrid model can improve performance significantly.
更多查看译文
关键词
Named entity recognition, Chinese electronic medical records, neural networks, hybrid models
AI 理解论文
溯源树
样例
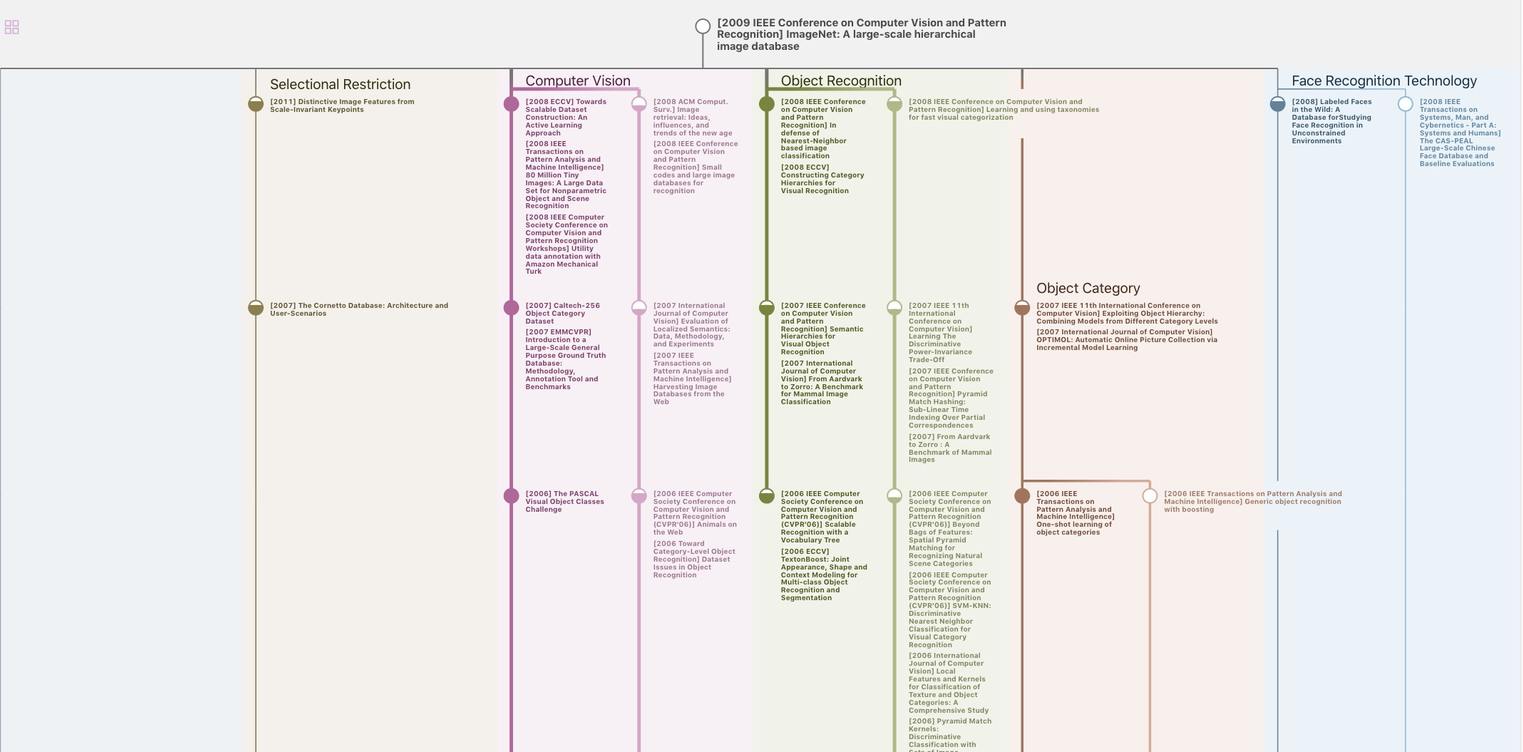
生成溯源树,研究论文发展脉络
Chat Paper
正在生成论文摘要