Effective Nonparametric Distribution Modeling for Distribution Approximation Applications
2020 SoutheastCon(2020)
摘要
Many fields of science rely on the collection of samples and estimation of true population distributions from those samples. There are several effective nonparametric methods for approximating a true distribution from empirical data, however it is unclear which methods produce the best approximations in practice. This work presents a case study on the effectiveness of various distribution approximations. Results show that piecewise linear approximations produce the smallest maximum absolute error, while the classic empirical distribution function (EDF) produces the smallest median absolute error as well as the smallest first quartile and minimum absolute error when approximating a distribution from a sample. When building distribution prediction models, the piecewise quintic and cubic approximations produce the lowest absolute error at most error percentiles. This case study encourages more research on the best methods of fitting empirical data with smooth functions to generate accurate distribution approximations.
更多查看译文
关键词
distribution approximation,population distributions,piecewise linear approximations,maximum absolute error,median absolute error,minimum absolute error,distribution prediction models,cubic approximations,empirical data fitting,nonparametric distribution modeling,empirical distribution function,sociology
AI 理解论文
溯源树
样例
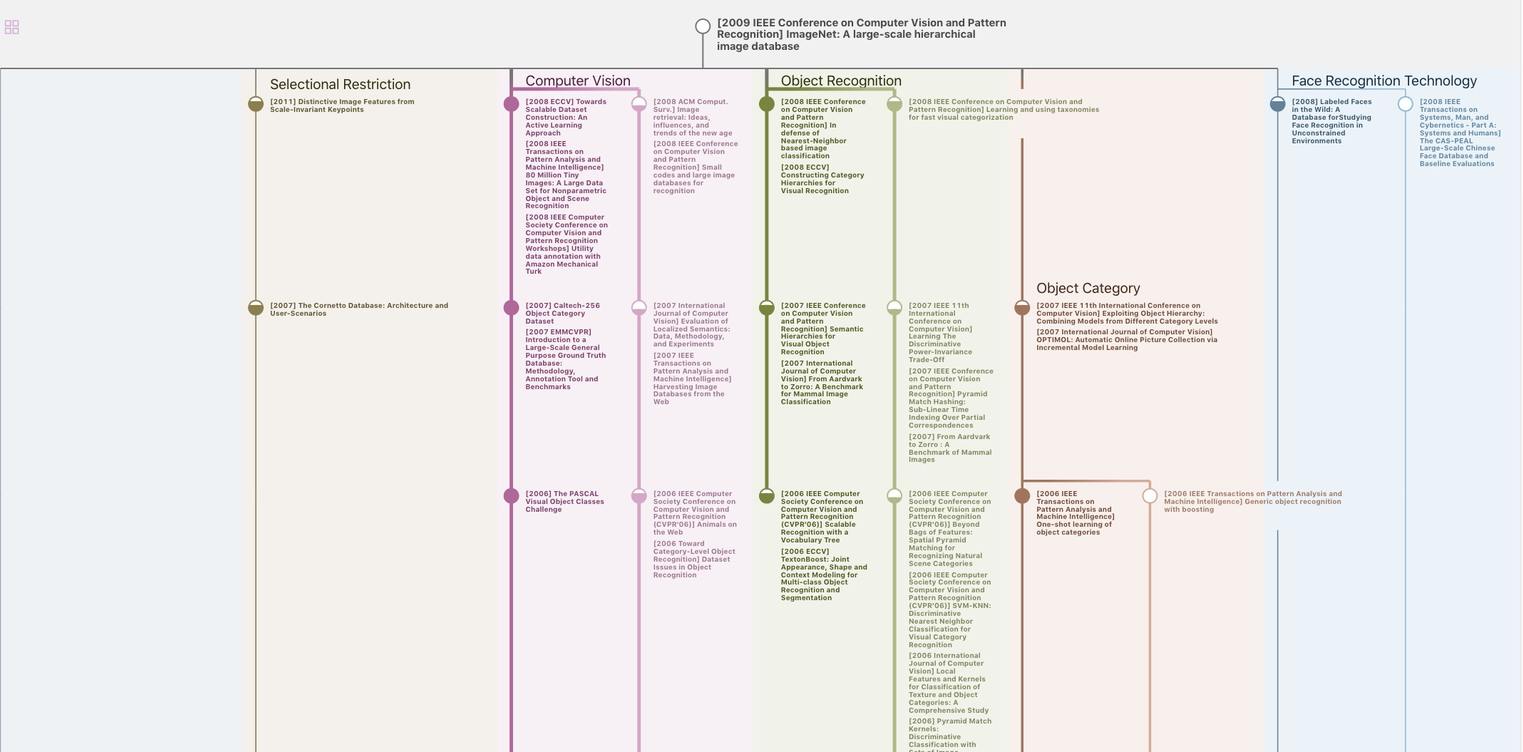
生成溯源树,研究论文发展脉络
Chat Paper
正在生成论文摘要