Improve conditional adversarial domain adaptation using self‐training
Iet Image Processing(2021)
摘要
Abstract Domain adaptation for image classification is one of the most fundamental transfer learning tasks and a promising solution to overcome the annotation burden. Existing deep adversarial domain adaptation approaches imply minimax optimization algorithms, matching the global features across domains. However, the information conveyed in unlabelled target samples is not fully exploited. Here, adversarial learning and self‐training are unified in an objective function, where the neural network parameters and the pseudo‐labels of target samples are jointly optimized. The model's predictions on unlabelled samples are leveraged to pseudo‐label target samples. The training procedure consists of two alternating steps. The first one is to train the network, while the second is to generate pseudo‐labels, and the loop continues. The proposed method achieves mean accuracy improvements of 2% on Office‐31, 0.7% on ImageCLEF‐DA, 1.8% on Office‐Home, and 1.2% on Digits than the baseline, which is superior to most state‐of‐the‐art approaches.
更多查看译文
关键词
Image recognition,Optimisation techniques,Computer vision and image processing techniques,Optimisation techniques,Neural nets
AI 理解论文
溯源树
样例
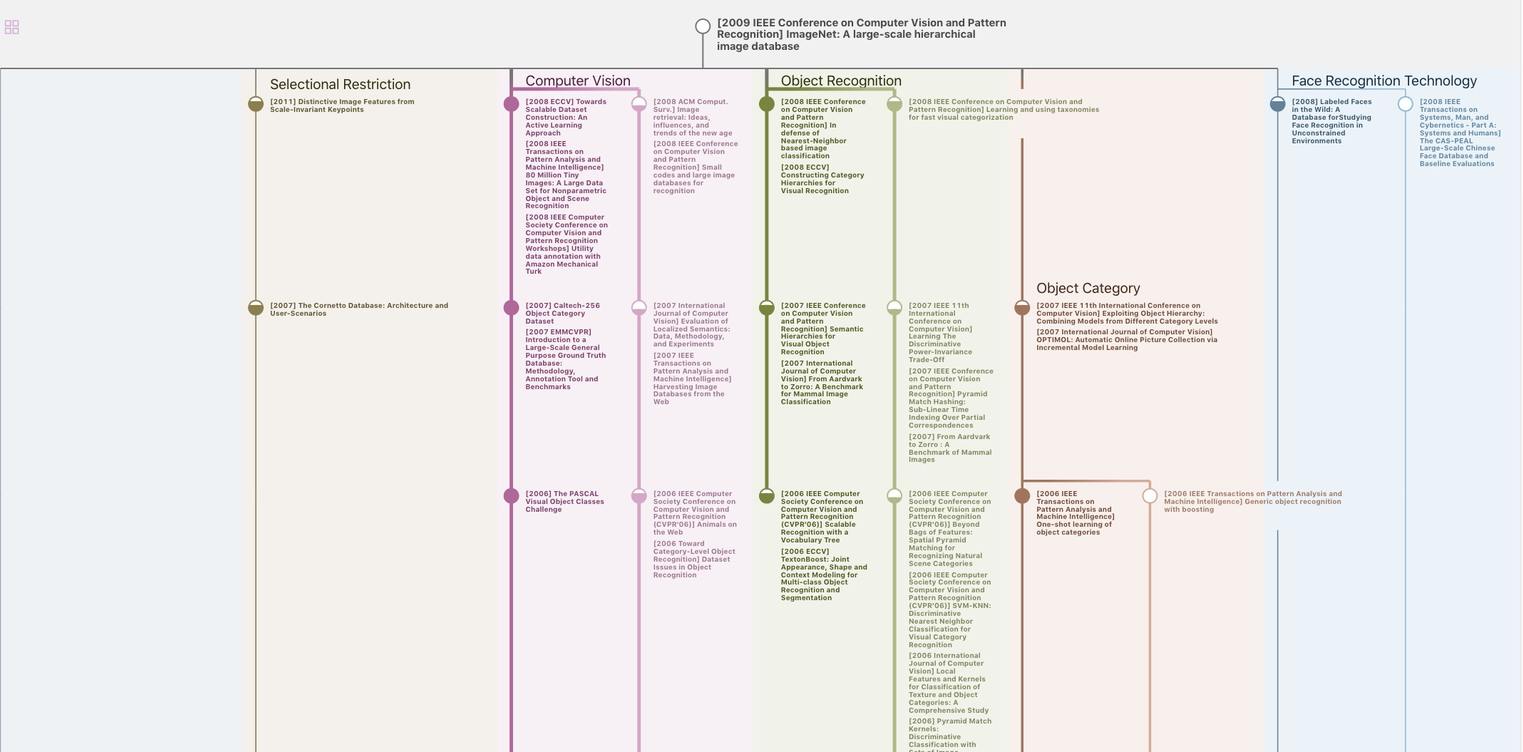
生成溯源树,研究论文发展脉络
Chat Paper
正在生成论文摘要