Data-Fusion-Based Two-Stage Cascade Framework for Multimodality Face Anti-Spoofing
IEEE Transactions on Cognitive and Developmental Systems(2022)
摘要
Existing face anti-spoofing models using deep learning for multimodality data suffer from low generalization in the case of using variety of presentation attacks, such as 2-D printing and high-precision 3-D face masks. One of the main reasons is that the nonlinearity of multispectral information used to preserve the intrinsic attributes between a real and a fake face is not well extracted. To address this issue, we propose a multimodility data-based two-stage cascade framework for face anti-spoofing. The proposed framework has two advantages. First, we design a two-stage cascade architecture that can selectively fuse low-level and high-level features from different modalities to improve feature representation. Second, we use multimodality data to construct a distance-free spectral on RGB and infrared to augment the nonlinearity of data. The presented data fusion strategy is different from popular fusion approaches, since it can strengthen discrimination ability of network models on physical attribute features than identity structure features under certain constraints. In addition, a multiscale patch-based weighted fine-tuning strategy is designed to learn each specific local face region. The experimental results show that the proposed framework achieves better performance than other state-of-the-art methods on both benchmark data sets and self-established data sets, especially on multimaterial masks spoofing.
更多查看译文
关键词
Convolutional neural network (CNN),deep learning,face anti-spoofing,multimodality fusion
AI 理解论文
溯源树
样例
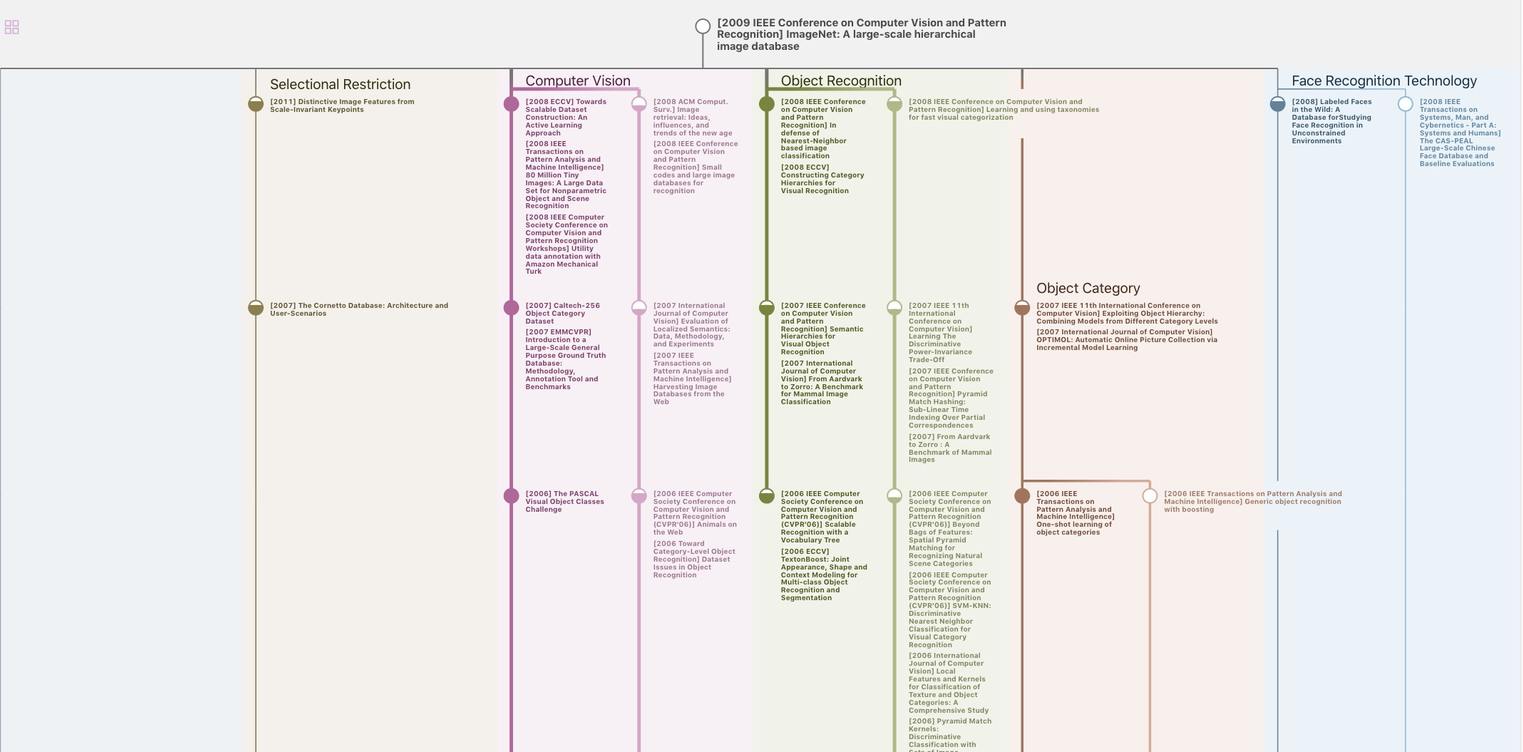
生成溯源树,研究论文发展脉络
Chat Paper
正在生成论文摘要