Identification of Autism Spectrum Disorder via an Eye-Tracking Based Representation Learning Model.
ICBRA(2020)
摘要
Autism spectrum disorder (ASD) is a lifelong developmental disorder characterized by repetitive, restricted behavior and deficits in communication and social interactions. Early diagnosis and intervention can significantly reduce the hazards of the disease. However, the lack of effective clinical resources for early diagnosis has been a long-standing problem. In response to this problem, we apply the recent advances in deep neural networks on eye-tracking data in this study to classify children with and without ASD. First, we record the eye movement data of 31 children with ASD and 43 typically developing children on four categories of stimuli to construct an eye-tracking data set for ASD identification. Based on the collected eye movement data, we extract the dynamic saccadic scanpath on each image for all subjects. Then, we utilize the hierarchical features learned from a convolutional neural network and multidimensional visual salient features to encode the scanpaths. Next, we adopt the support vector machine to learn the relationship between encoded pieces of scanpaths and the labels from the two classes via supervised learning. Finally, we derive the scores of each scanpath and make the final judgment for each subject according to the scores on all scanpaths. The experimental results have shown that the proposed model has a maximum classification accuracy of 94.28% in the diagnostic tests. Based on existing research and calculation models, dynamic saccadic scanpaths can provide promising findings and implications for ASD early detection. Furthermore, integrating more information of the scanpaths into the model and developing a more in-depth description of scanpaths can improve the recognition accuracy. We hope our work can contribute to the development of multimodal approaches in the early detection and diagnosis of ASD.
更多查看译文
AI 理解论文
溯源树
样例
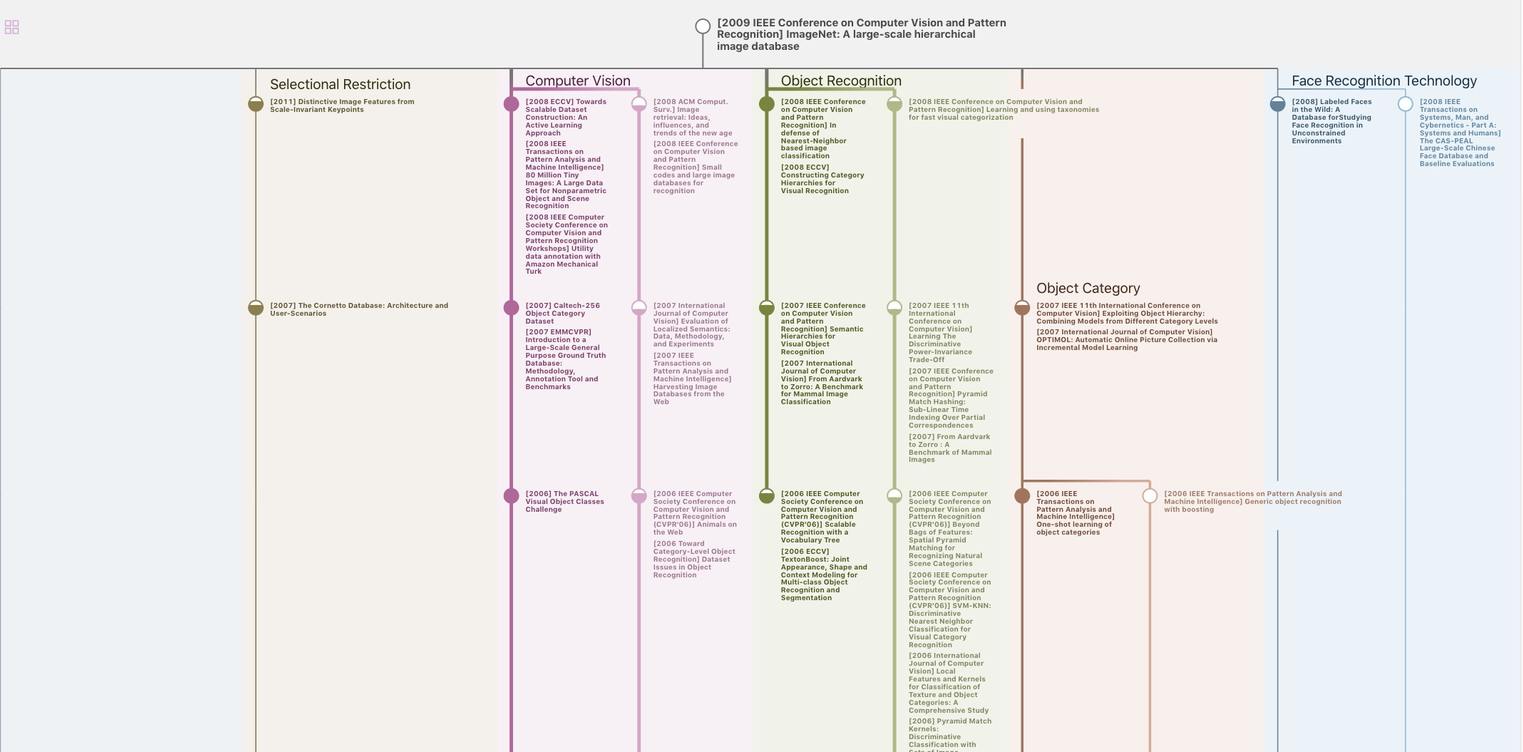
生成溯源树,研究论文发展脉络
Chat Paper
正在生成论文摘要