Solving Sokoban with backward reinforcement learning
arxiv(2021)
摘要
In some puzzles, the strategy we need to use near the goal can be quite different from the strategy that is effective earlier on, e.g. due to a smaller branching factor near the exit state in a maze. A common approach in these cases is to apply both a forward and a backward search, and to try and align the two. In this work we propose an approach that takes this idea a step forward, within a reinforcement learning (RL) framework. Training a traditional forward-looking agent using RL can be difficult because rewards are often sparse, e.g. given only at the goal. Instead, we first train a backward-looking agent with a simple relaxed goal. We then augment the state representation of the puzzle with straightforward hint features that are extracted from the behavior of that agent. Finally, we train a forward looking agent with this informed augmented state. We demonstrate that this simple "access" to partial backward plans leads to a substantial performance boost. On the challenging domain of the Sokoban puzzle, our RL approach substantially surpasses the best learned solvers that generalize over levels, and is competitive with SOTA performance of the best highly-crafted solution. Impressively, we achieve these results while learning from only a small number of practice levels and using simple RL techniques.
更多查看译文
关键词
sokoban,reinforcement,learning
AI 理解论文
溯源树
样例
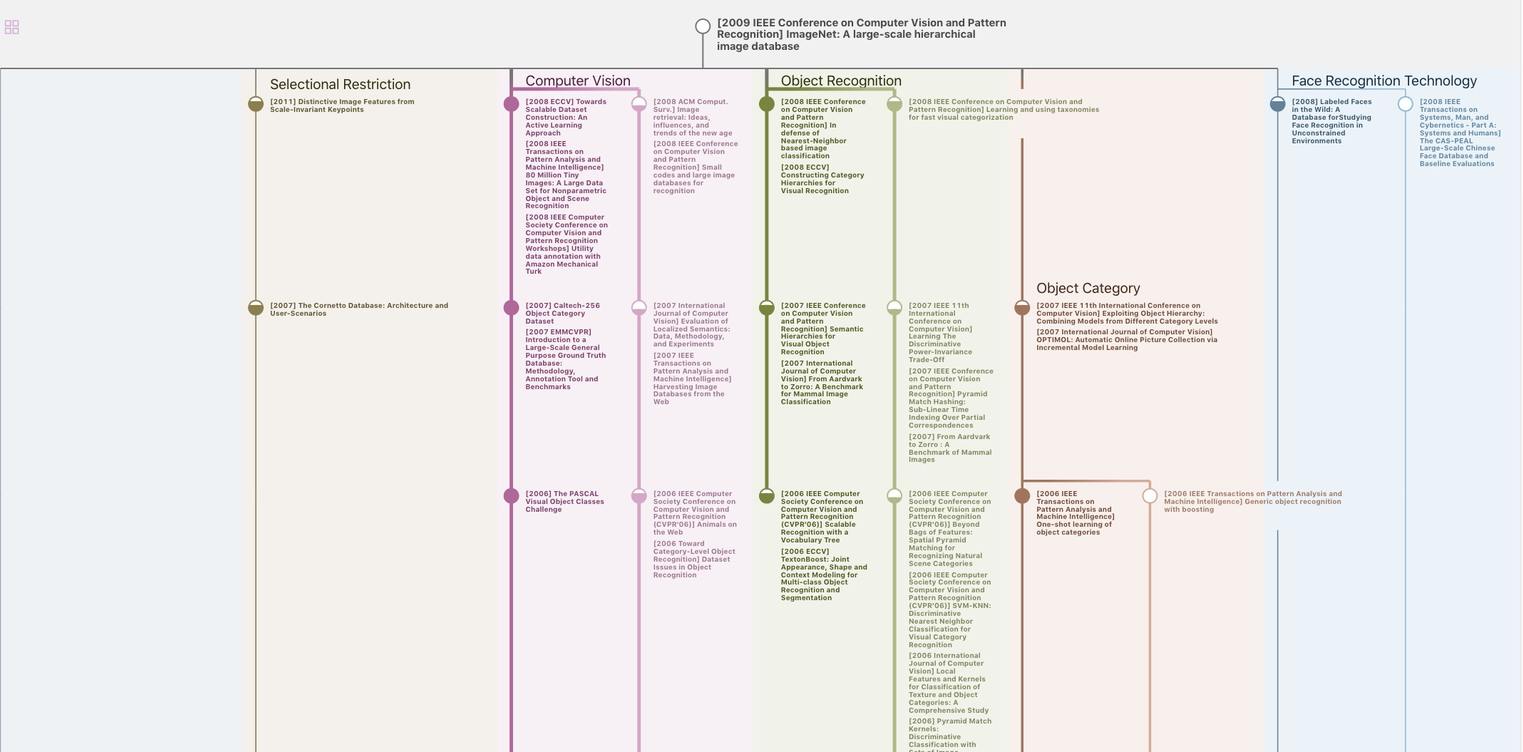
生成溯源树,研究论文发展脉络
Chat Paper
正在生成论文摘要