Move to See Better: Self-Improving Embodied Object Detection.
British Machine Vision Conference(2021)
摘要
Passive methods for object detection and segmentation treat images of the same scene as individual samples and do not exploit object permanence across multiple views. Generalization to novel or difficult viewpoints thus requires additional training with lots of annotations. In contrast, humans often recognize objects by simply moving around, to get more informative viewpoints. In this paper, we propose a method for improving object detection in testing environments, assuming nothing but an embodied agent with a pre-trained 2D object detector. Our agent collects multi-view data, generates 2D and 3D pseudo-labels, and fine-tunes its detector in a self-supervised manner. Experiments on both indoor and outdoor datasets show that (1) our method obtains high-quality 2D and 3D pseudo-labels from multi-view RGB-D data; (2) fine-tuning with these pseudo-labels improves the 2D detector significantly in the test environment; (3) training a 3D detector with our pseudo-labels outperforms a prior self-supervised method by a large margin; (4) given weak supervision, our method can generate better pseudo-labels for novel objects.
更多查看译文
关键词
Object detection,Embodied agent,Object permanence,Detector,Margin (machine learning),Computer vision,Segmentation,Viewpoints,Computer science,Exploit,Artificial intelligence
AI 理解论文
溯源树
样例
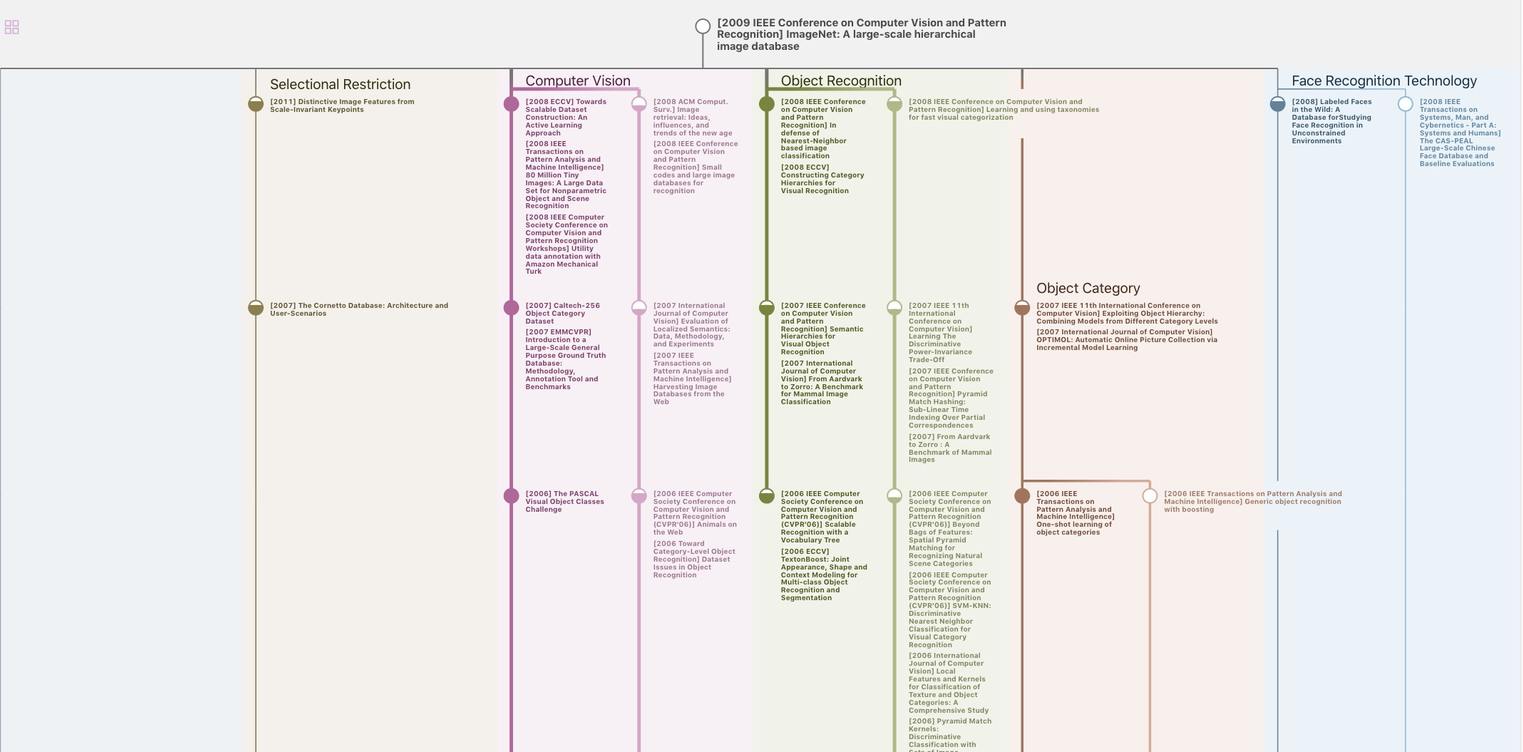
生成溯源树,研究论文发展脉络
Chat Paper
正在生成论文摘要