Micro-environment Recognition in the context of Environmental Crowdsensing.
EDBT/ICDT Workshops(2021)
摘要
With the rapid advancements of sensor technologies and mobile computing, Mobile Crowd-Sensing (MCS) has emerged as a new paradigm to collect massive-scale rich trajectory data. Nomadic sensors empower people and objects with the capability of reporting and sharing observations on their state, their behavior and/or their surrounding environments. Processing and mining multi-source sensor data in MCS raise several challenges due to their multi-dimensional nature where the measured parameters (i.e., dimensions) may differ in terms of quality, variabilty, and time scale. We consider the context of air quality MCS, and focus on the task of mining the context from the MCS data. Relating the measures to their context is crucial to interpret them and analyse the participant\u0027s exposure. This paper investigates the feasibility of recognizing the human\u0027s context (called herein micro-environment) in an environmental MCS scenario. We put forward a multi-view learning approach, that we adapt to our context, and implement it along with other time series classification approaches. The experimental results, applied to real MCS data, not only confirm the power of MCS data in characterizing the micro-environment, but also show a moderate impact of the integration of mobility data in this recognition. Furthermore, multi-view learning shows similar performance as the reference deep learning algorithm, without requiring specific hardware.
更多查看译文
AI 理解论文
溯源树
样例
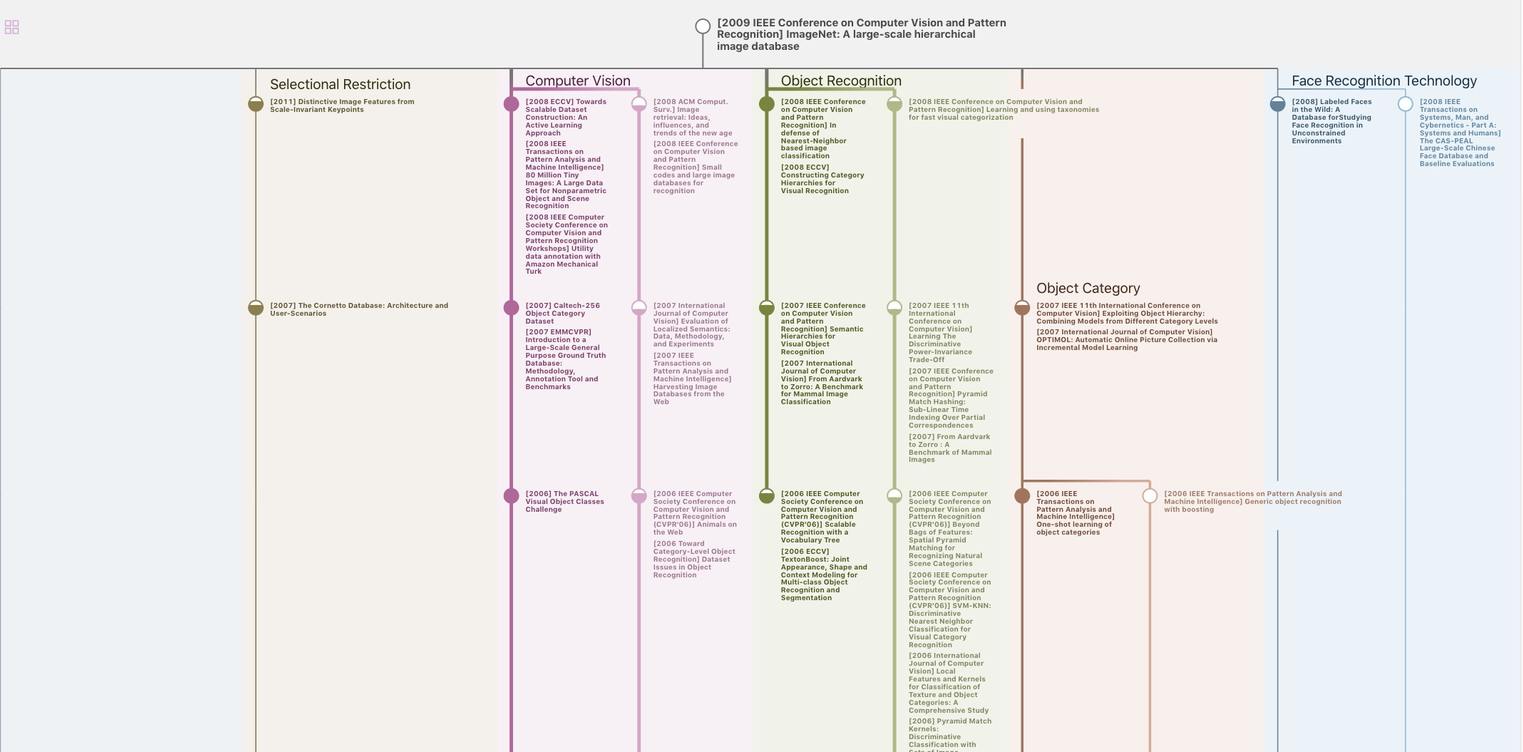
生成溯源树,研究论文发展脉络
Chat Paper
正在生成论文摘要