Meta-Learning Bidirectional Update Rules
INTERNATIONAL CONFERENCE ON MACHINE LEARNING, VOL 139(2021)
摘要
In this paper, we introduce a new type of generalized neural network where neurons and synapses maintain multiple states. We show that classical gradient-based backpropagation in neural networks can be seen as a special case of a twostate network where one state is used for activations and another for gradients, with update rules derived from the chain rule. In our generalized framework, networks have neither explicit notion of nor ever receive gradients. The synapses and neurons are updated using a bidirectional Hebb-style update rule parameterized by a shared low-dimensional "genome". We show that such genomes can be meta-learned from scratch, using either conventional optimization techniques, or evolutionary strategies, such as CMA-ES. Resulting update rules generalize to unseen tasks and train faster than gradient descent based optimizers for several standard computer vision and synthetic tasks.
更多查看译文
关键词
update,rules,meta-learning
AI 理解论文
溯源树
样例
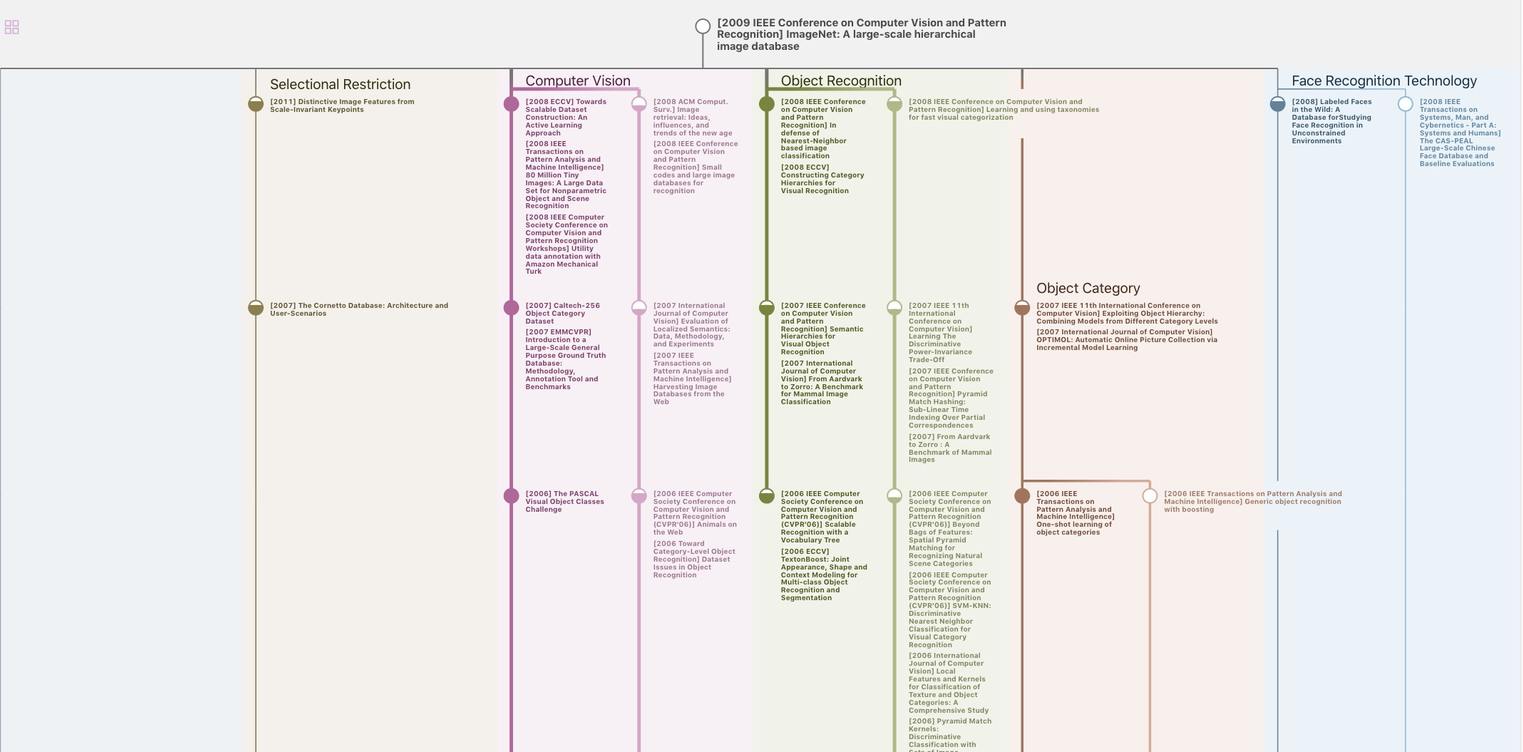
生成溯源树,研究论文发展脉络
Chat Paper
正在生成论文摘要