Dynamically changing sequencing rules with reinforcement learning in a job shop system with stochastic influences
Winter Simulation Conference(2020)
摘要
ABSTRACTSequencing operations can be difficult, especially under uncertain conditions. Applying decentral sequencing rules has been a viable option; however, no rule exists that can outperform all other rules under varying system performance. For this reason, reinforcement learning (RL) is used as a hyper heuristic to select a sequencing rule based on the system status. Based on multiple training scenarios considering stochastic influences, such as varying inter arrival time or customers changing the product mix, the advantages of RL are presented. For evaluation, the trained agents are exploited in a generic manufacturing system. The best agent trained is able to dynamically adjust sequencing rules based on system performance, thereby matching and outperforming the presumed best static sequencing rules by ≈ 3%. Using the trained policy in an unknown scenario, the RL heuristic is still able to change the sequencing rule according to the system status, thereby providing robust performance.
更多查看译文
关键词
dynamically changing sequencing rules,reinforcement learning,job shop system,stochastic influences,sequencing operations,uncertain conditions,decentral sequencing rules,system performance,sequencing rule,multiple training scenarios,trained agents,generic manufacturing system,presumed best static sequencing rules,RL heuristic
AI 理解论文
溯源树
样例
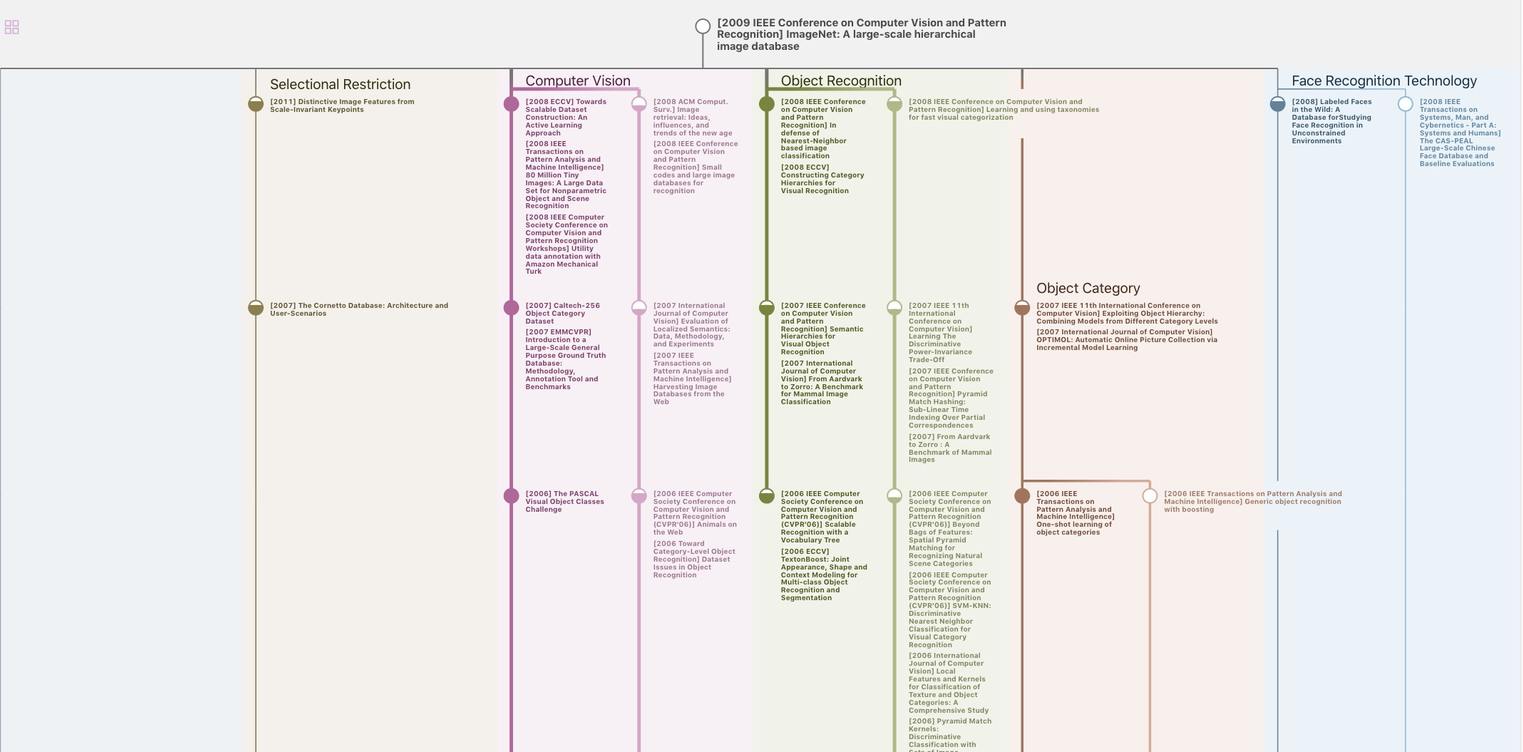
生成溯源树,研究论文发展脉络
Chat Paper
正在生成论文摘要