Fairness-Aware PageRank
WWW 2021
摘要
Algorithmic fairness has attracted significant attention in the past years. In this paper, we consider fairness for link analysis and in particular for the celebrated Pagerank algorithm. Given that the nodes in a network belong to groups (for example, based on demographic or other characteristics), we provide a parity-based definition of fairness that imposes constraints on the proportion of Pagerank allocated to the members of each group. We propose two families of fair Pagerank algorithms: the first (Fairness-Sensitive Pagerank) modifies the jump vector of the Pagerank algorithm to enforce fairness; the second (Locally Fair Pagerank) imposes a fair behavior per node. We then define a stronger fairness requirement, termed universal personalized fairness, that asks that the derived personalized pageranks of all nodes are fair. We prove that the locally fair algorithms achieve also universal personalized fairness, and furthermore, we prove that this is the only family of algorithms with this property, establishing an equivalence between universal personalized fairness and local fairness. We also consider the problem of achieving fairness while minimizing the utility loss with respect to the original Pagerank algorithm. We present experiments with real and synthetic networks that examine the fairness of the original Pagerank and demonstrate qualitatively and quantitatively the properties of our algorithms.
更多查看译文
关键词
networks, pagerank, link analysis, fairness, homophily
AI 理解论文
溯源树
样例
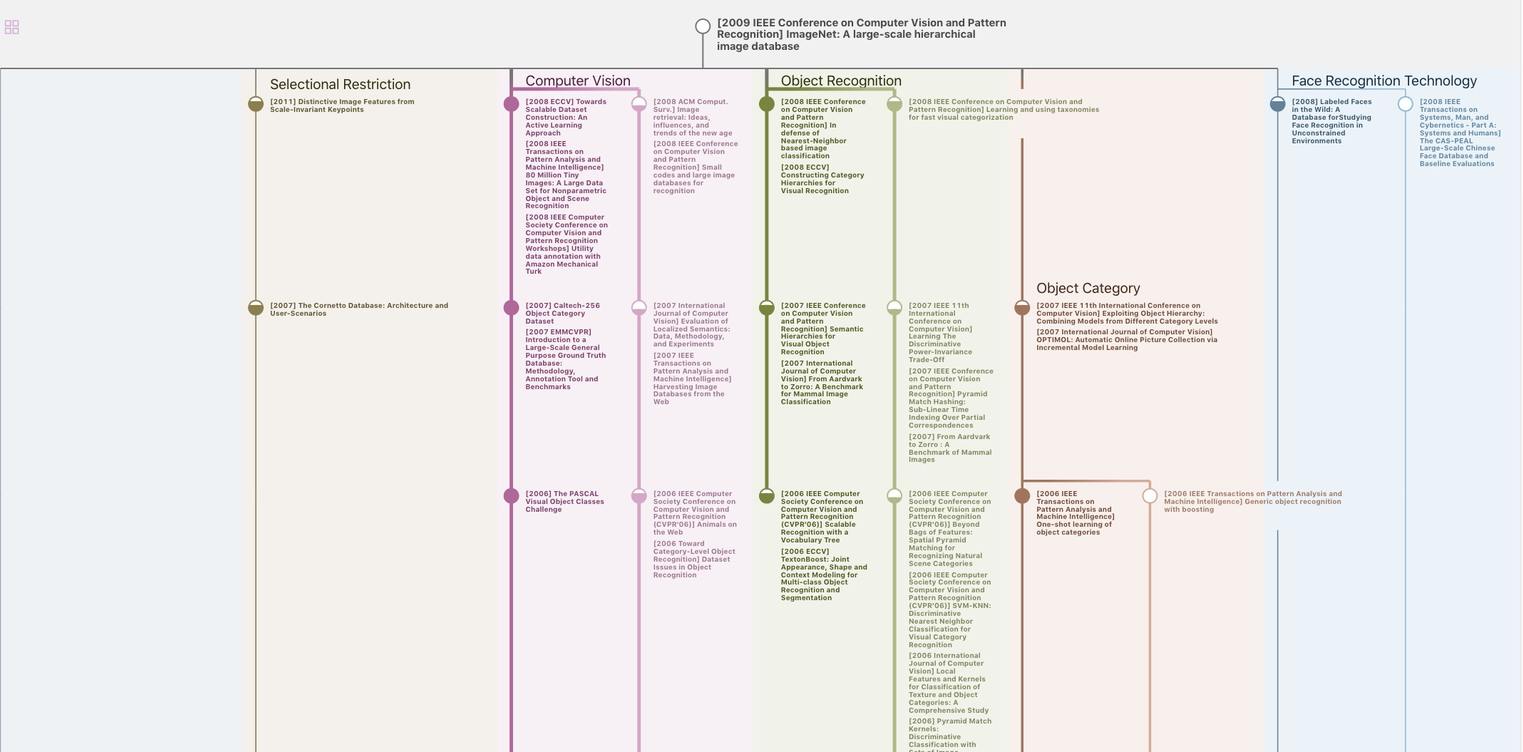
生成溯源树,研究论文发展脉络
Chat Paper
正在生成论文摘要