Modelling Spatio-Temporal Ageing Phenomena With Deep Generative Adversarial Networks
SIGNAL PROCESSING-IMAGE COMMUNICATION(2021)
摘要
Deterioration modelling of ageing phenomena on materials is an actively researched topic in computer graphics and vision, with a wide range of applications in domains such as cultural heritage, game programming, material science and virtual reality. As a result significant progress has been accomplished and existing methods are able to produce visually pleasing results that appear realistic. However, there is a very limited connection to comprehensive measurements that actually capture the ageing process of a material. This paper focuses on this gap, aiming to provide a link between physical measurements and deterioration modelling. Based on extensive measurements of texture and surface geometry of artificially aged reference materials, a Deep Learning (DL) framework is proposed that models spatio-temporal variations on the 3D surface geometry and the 2D colour? image appearance. Concretely, the problem of material degradation over time is formulated as an 2D/3D material-to-material translation problem, where the goal is, given an input material and a target degradation time, to output the degraded material at that time. At the core of the method lies a modified conditional Generative Adversarial Network (cGAN), which maps input materials to degraded materials over time. In order to train and deploy the proposed cGAN model, proper data parameterization and augmentation steps are introduced. As shown through extensive experimentation on real data coming from materials commonly found in artwork and from actual artworks, the proposed approach produces high quality results.
更多查看译文
关键词
Ageing simulation, Adversarial learning, Conditional GANs
AI 理解论文
溯源树
样例
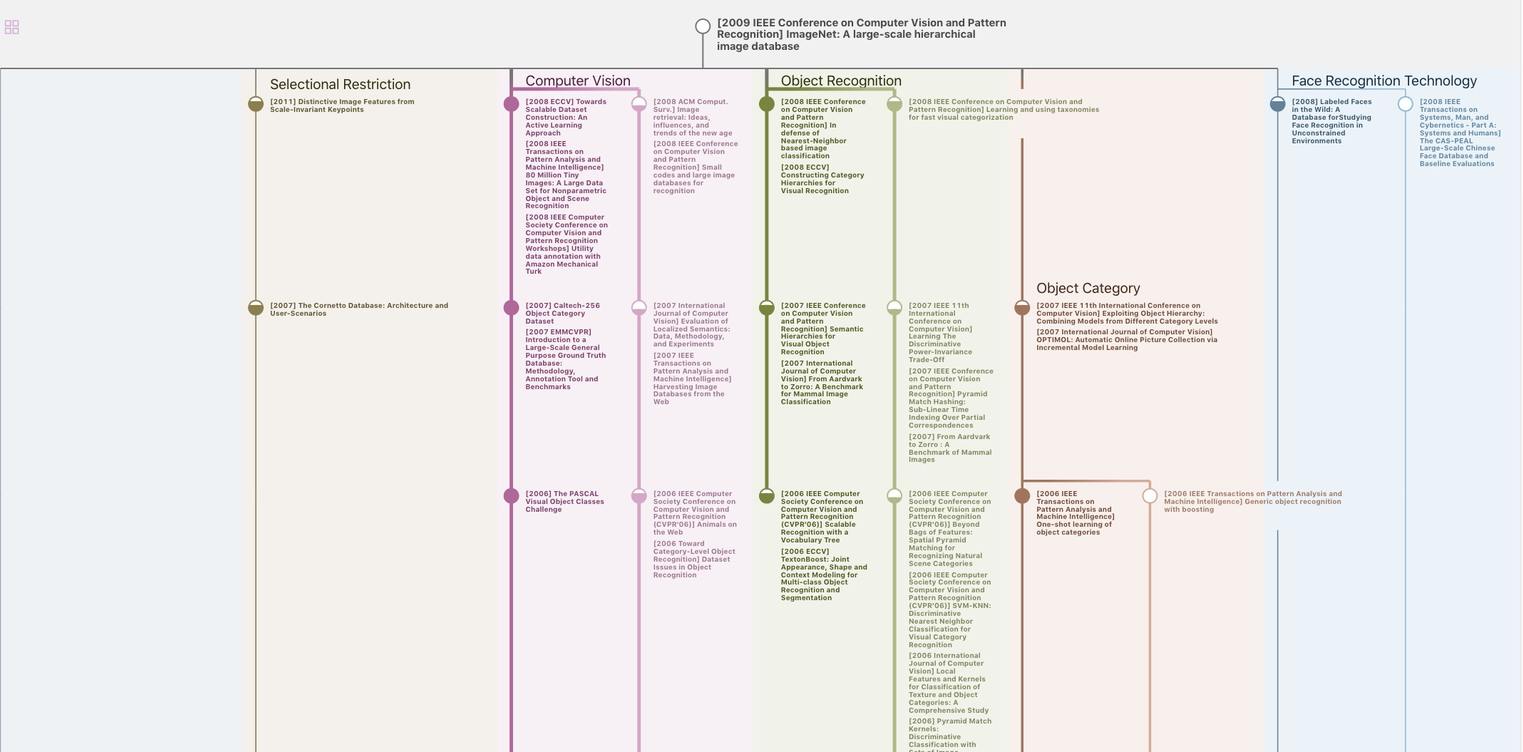
生成溯源树,研究论文发展脉络
Chat Paper
正在生成论文摘要