A Hybrid Model for Time Series Prediction Using Adaptive Variational Mode Decomposition
2020 Chinese Automation Congress (CAC)(2020)
摘要
Due to the non-stationarity and irregularity typically exist in real-world time series, it is generally difficult to achieve satisfied performance for direct prediction as well as avoid of hysteresis. In order to solve these problems, an adaptive hybrid model is proposed in this study. The time series is transformed into a series of sub-sequences using the Variational Mode Decomposition (VMD) method at first. Then, a hybrid model, which is constructed by the Gated Recurrent Unit (GRU) neural networks and Gaussian Process Regression (GPR) model, is used for prediction. In addition, Bayesian optimization technique is deployed to hierarchically optimize the parameters of the VMD method for making the decomposed sub-sequences more suitable for the sub-models along with further improving the prediction accuracy. Finally, comparing with other commonly deployed methods, the proposed model exhibits superior performance on predicting real-world time series in both short-term and long-term.
更多查看译文
关键词
Time series prediction,Variational Mode Decomposition,Bayesian optimization,Gated Recurrent Unit neural networks,Gaussian Process Regression,Hybrid model
AI 理解论文
溯源树
样例
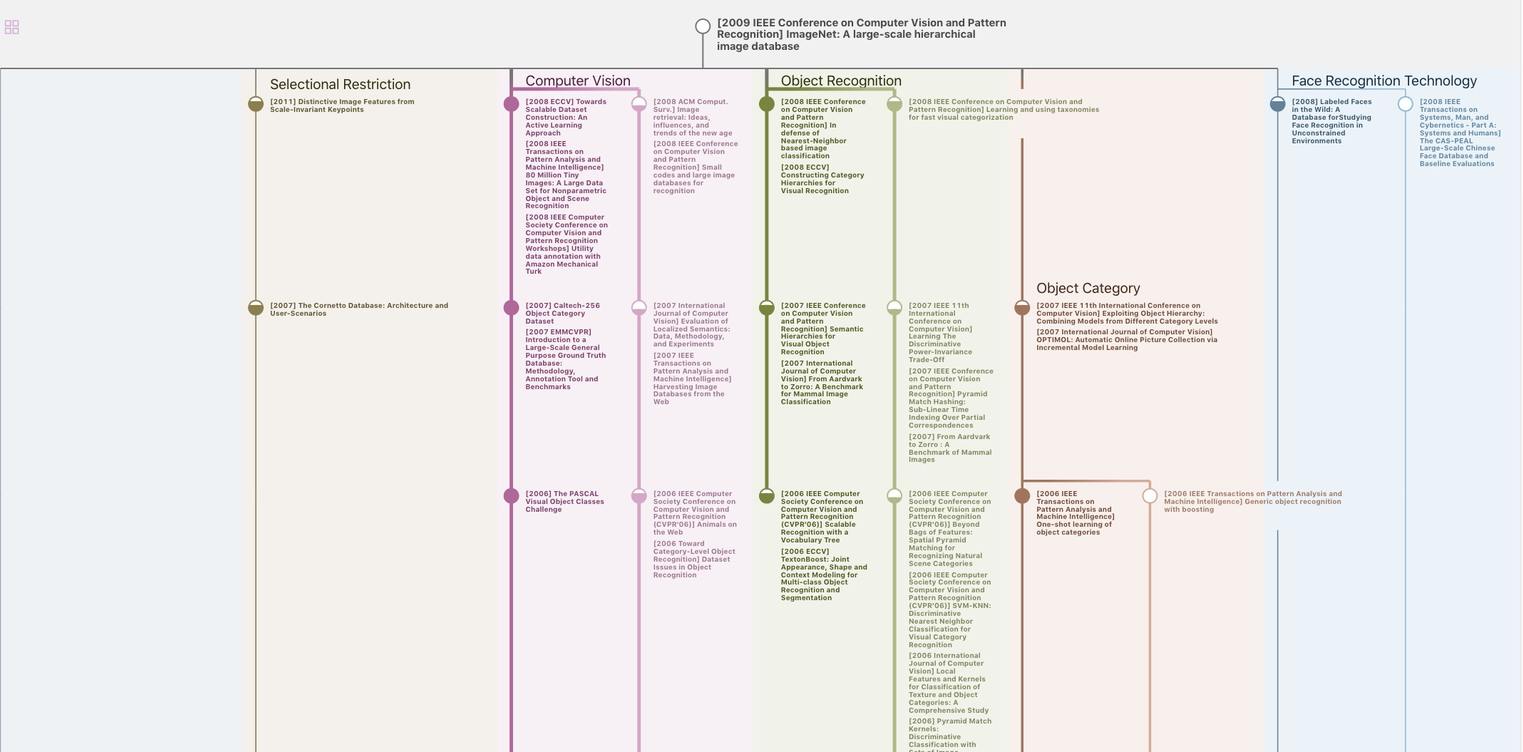
生成溯源树,研究论文发展脉络
Chat Paper
正在生成论文摘要