Combining Images And T-Staging Information To Improve The Automatic Segmentation Of Nasopharyngeal Carcinoma Tumors In Mr Images
IEEE ACCESS(2021)
摘要
The accurate and reproducible delineation of tumors from uninvolved tissue is essential for radiation oncology. However, the tumor margin may be challenging to identify from magnetic resonance (MR) images of nasopharyngeal carcinomas (NPCs). Additionally, clinical diagnoses such as T-staging may already provide some information on tumor invasion. To use this information and improve the performance of tumor segmentation, we propose a novel deep learning neural network architecture that can incorporate both T-staging and image information. Based on U-Net, our model adds a T-channel composed of T-staging information and uses the attention mechanism. Since the T-staging information is defined by the extent of tumor invasion, the T-channel using T-staging information can improve the segmentation accuracy at different stages. Additionally, the addition of an attention mechanism allows our model to retain the most valuable pixels of the image, thus further improving the delineation accuracy. In our experiments, the proposed network was trained and validated based on records from 251 clinical patients using 10-fold cross-validation. The dice similarity coefficient (DSC) and average symmetric surface distance (ASSD) were used to evaluate our network's results. The average DSC and ASSD and their standard deviation (SD) values are 0.841 +/- 0.011 and 0.747 +/- 0.199 mm. The unique T-channel effectively utilizes T-staging information to improve the results. With the combination of the T-channel module and the attention module, we significantly improved NPC tumor delineation performance.
更多查看译文
关键词
Image segmentation, Tumors, Feature extraction, Deep learning, Convolution, Decoding, Task analysis, Deep learning, magnetic resonance images, autosegmentation, nasopharyngeal carcinoma
AI 理解论文
溯源树
样例
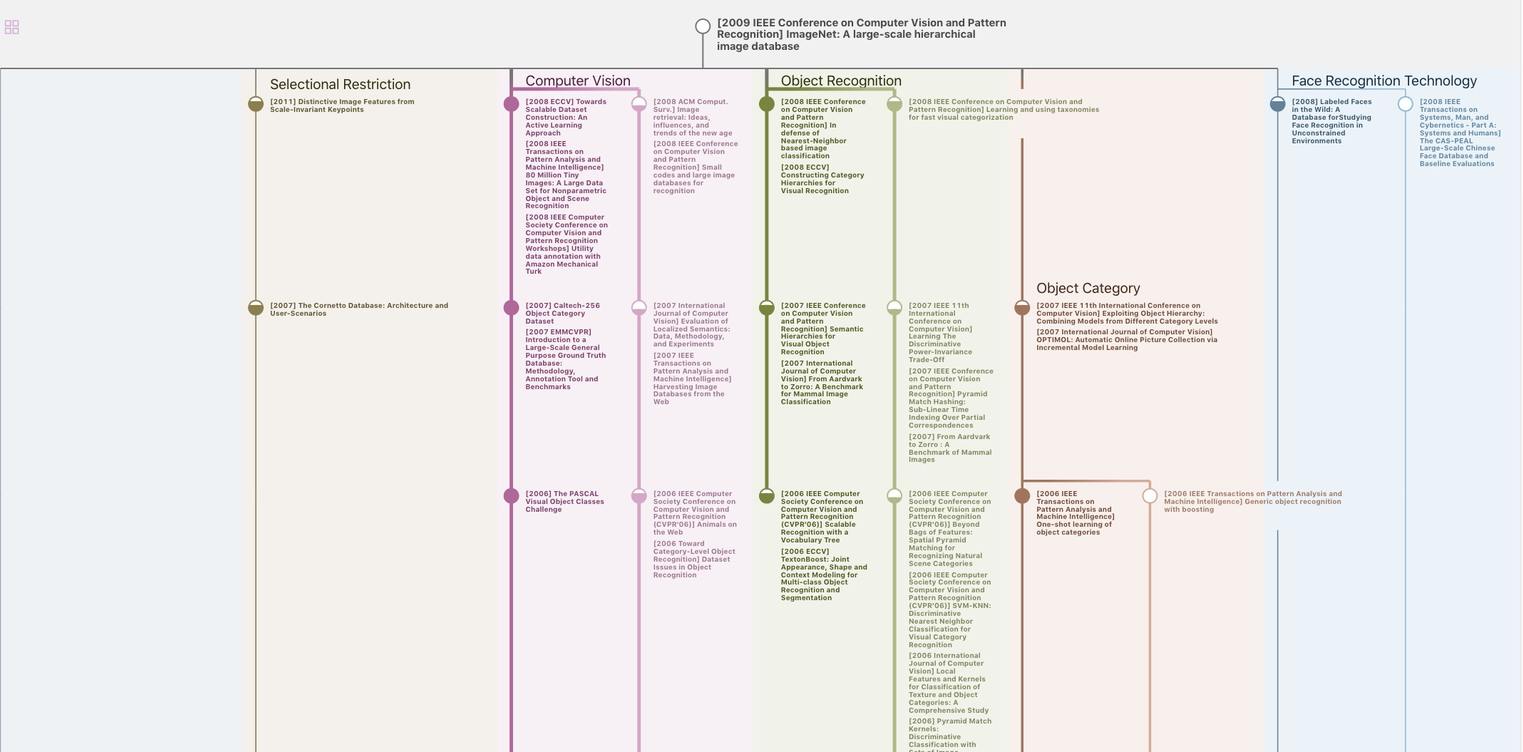
生成溯源树,研究论文发展脉络
Chat Paper
正在生成论文摘要