DFNet: Deep Fusion Network for 3D Shape Classification and Retrieval
chinese automation congress(2020)
摘要
3D shape classification and retrieval are the primary tasks in computer vision with great application value. Recent researches have benefited a lot from deep learning methods, mainly around point-based and view-based methods. The former lacks robustness under different perspectives, while the latter lacks the ability to capture global features of 3D objects. Inspired by the way humans perceive a 3D object, first to receive its 3D global characteristics and then learn more details from multiple perspectives, we design a Deep Fusion Network (DFNet) that combines a point-based network (PointNet) which reflects intrinsic properties of 3D shape from the point cloud and a view-based network (ViewNet) which captures spatio-temporal features from shape\u0027s sequential projections through the combination of CNNs and LSTM. A high-level 3D shape feature descriptor, combining the advantages of the two features merits, is finally obtained by FusionNet. Experimental results show that our proposed DFNet which fuses point-based and view-based features achieves better performance than only one feature based network. And comparison with other excellent methods shows that DFNet outperforms under two large-scale 3D shape benchmarks.
更多查看译文
关键词
DFNet,deep fusion network,3D shape classification,deep learning methods,global features,point-based network,point cloud,view-based network,spatio-temporal features,high-level 3D shape feature descriptor,feature based network,CNNs,LSTM,shape sequential projections,PointNet
AI 理解论文
溯源树
样例
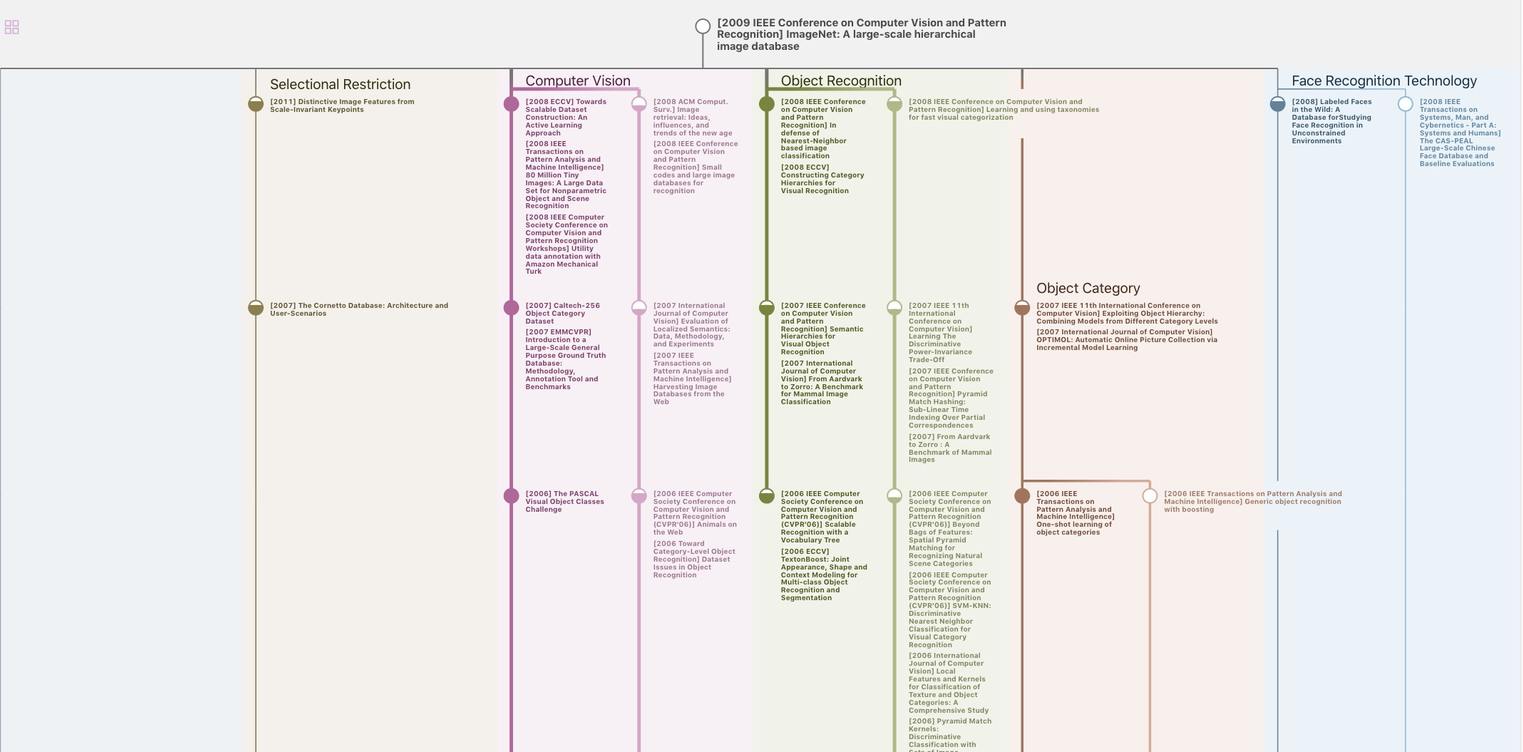
生成溯源树,研究论文发展脉络
Chat Paper
正在生成论文摘要