MI-EEG Temporal Information Learning Based on One-Dimensional Convolutional Neural Network
2020 35th Youth Academic Annual Conference of Chinese Association of Automation (YAC)(2020)
摘要
The Brain-Computer Interface (BCI) is a special communication system that does not rely on the nerve and muscle tissue around the human body. it realizes the direct communication between the brain and the computer. The key to the realization of the BCI system is reasonable extraction and analysis of the characteristics for electroencephalogram (EEG) signals. Although deep learning has made initial progress in this field, only a few works have discussed the characteristics of EEG signals learned by deep learning. In this paper, an end-to-end network model for deep learning is designed to extract the time domain information of MI EEG signals. The model uses a one-dimensional convolution kernel to learn the time-domain information in the MI-EEG signal. Furthermore, we analyzed the frequency-dependent characteristics of the convolution kernel. We use the BCI competition dataset as a standard to measure the performance of this method. The experimental results show that the neural network tends to learn information in a specific frequency band, and each person's specific frequency band is different. The classification result of the motor imagery obtained by the network is better than the competition's winner.
更多查看译文
关键词
brain-computer interface,motor imagery(MI),electro-encephalogram(EEG),convolutional neural network(CNN),time-frequency domain
AI 理解论文
溯源树
样例
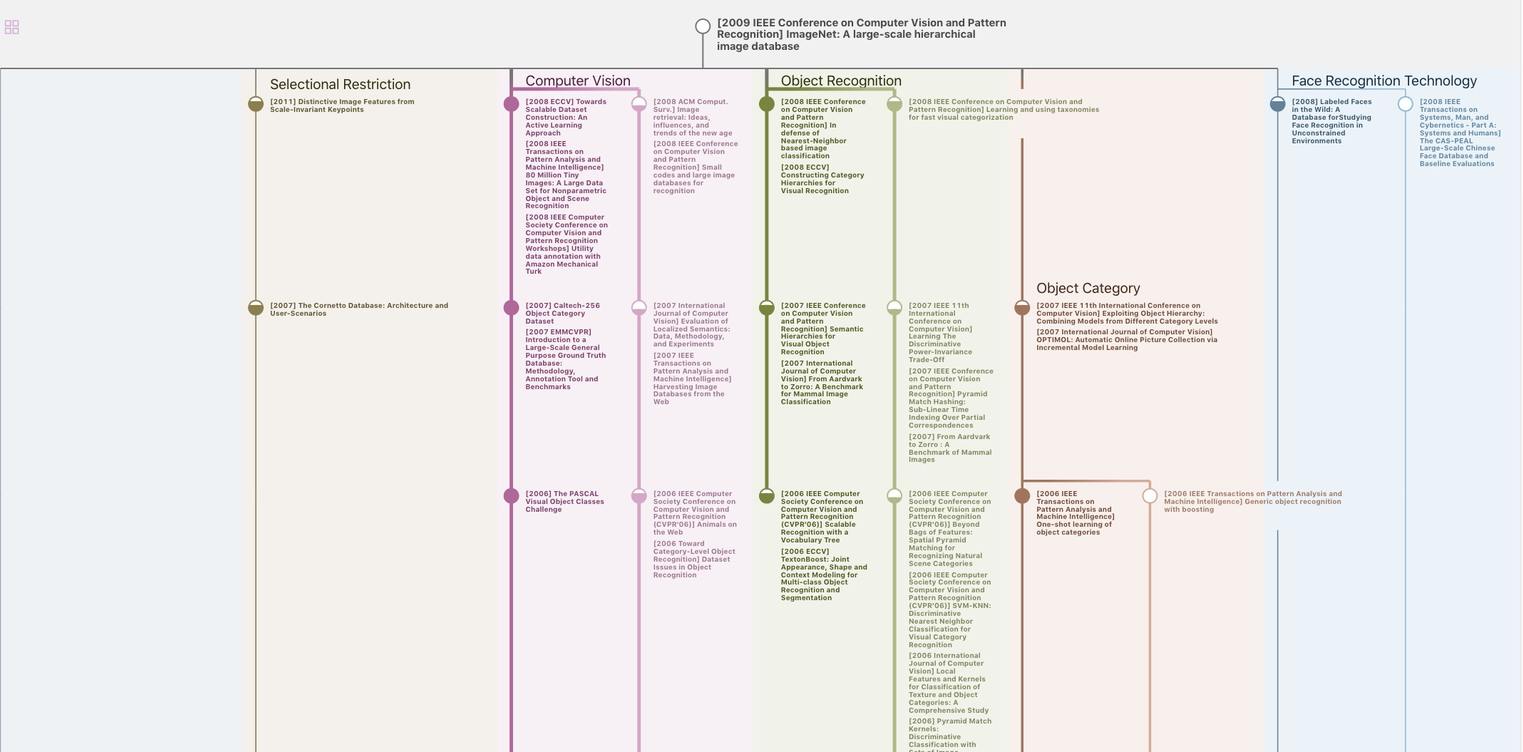
生成溯源树,研究论文发展脉络
Chat Paper
正在生成论文摘要