RMIX: Risk-Sensitive Multi-Agent Reinforcement Learning
user-5f8cf7e04c775ec6fa691c92(2021)
摘要
Centralized training with decentralized execution (CTDE) has become an important paradigm in multi-agent reinforcement learning (MARL). Current CTDE-based methods rely on restrictive decompositions of the centralized value function across agents, which decomposes the global Q-value into individual Q values to guide individuals' behaviours. However, such expected, i.e., risk-neutral, Q value decomposition is not sufficient even with CTDE due to the randomness of rewards and the uncertainty in environments, which causes the failure of these methods to train coordinating agents in complex environments. To address these issues, we propose RMIX, a novel cooperative MARL method with the Conditional Value at Risk (CVaR) measure over the learned distributions of individuals' Q values. Our main contributions are in three folds: (i) We first learn the return distributions of individuals to analytically calculate CVaR for decentralized execution; (ii) We then propose a dynamic risk level predictor for CVaR calculation to handle the temporal nature of the stochastic outcomes during executions; (iii) We finally propose risk-sensitive Bellman equation along with Individual-Global-MAX (IGM) for MARL training. Empirically, we show that our method significantly outperforms state-of-the-art methods on many challenging StarCraft II tasks, demonstrating significantly enhanced coordination and high sample efficiency.
更多查看译文
关键词
CVAR,Expected shortfall,Reinforcement learning,Bellman equation,Randomness,Mathematical optimization,Computer science,Risk level,Risk sensitive
AI 理解论文
溯源树
样例
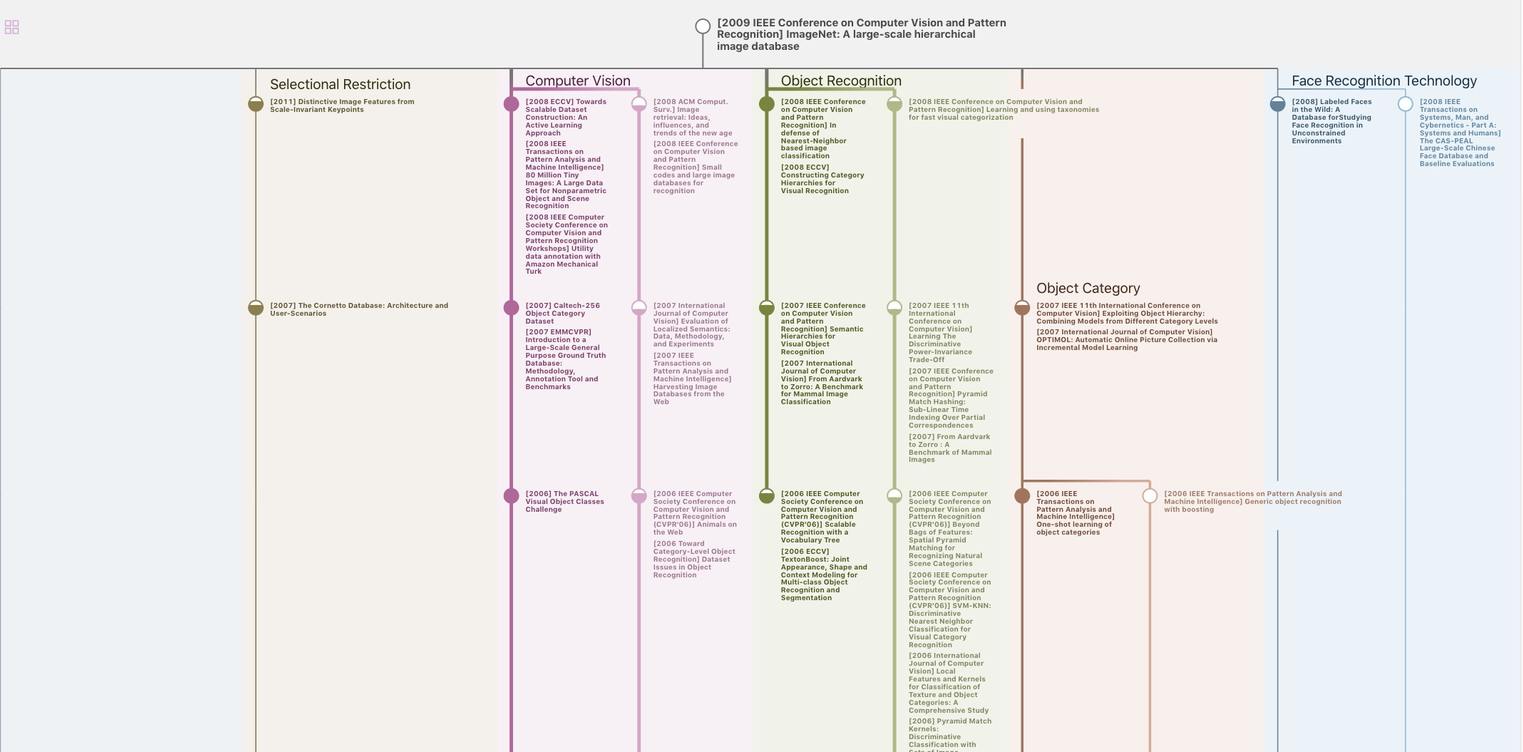
生成溯源树,研究论文发展脉络
Chat Paper
正在生成论文摘要