Sample Balancing for Improving Generalization under Distribution Shifts
user-5edf3a5a4c775e09d87cc848(2021)
摘要
Deep neural networks have achieved striking performance when evaluated on testing data which share the same distribution with training ones, but can significantly fail otherwise. Therefore, eliminating the impact of distribution shifts between training and testing data is of paramount importance for building performance-promising deep models. Conventional methods (e.g. domain adaptation/generalization) assume either the availability of testing data or the known heterogeneity of training data (e.g. domain labels). In this paper, we consider a more challenging case where neither of the above information is available during the training phase. We propose to address this problem by removing the dependencies between features via reweighting training samples, which results in a more balanced distribution and helps deep models get rid of spurious correlations and, in turn, concentrate more on the true connection between features and labels. We conduct extensive experiments on object recognition benchmarks including PACS, VLCS, MNIST-M, and NICO which support the evaluation of generalization ability. The experimental results clearly demonstrate the effectiveness of the proposed method compared with state-of-the-art counterparts.
更多查看译文
关键词
Test data,Spurious relationship,Contextual image classification,Cognitive neuroscience of visual object recognition,Machine learning,Computer science,Artificial intelligence,Deep neural networks,Domain adaptation,Training phase,Training set
AI 理解论文
溯源树
样例
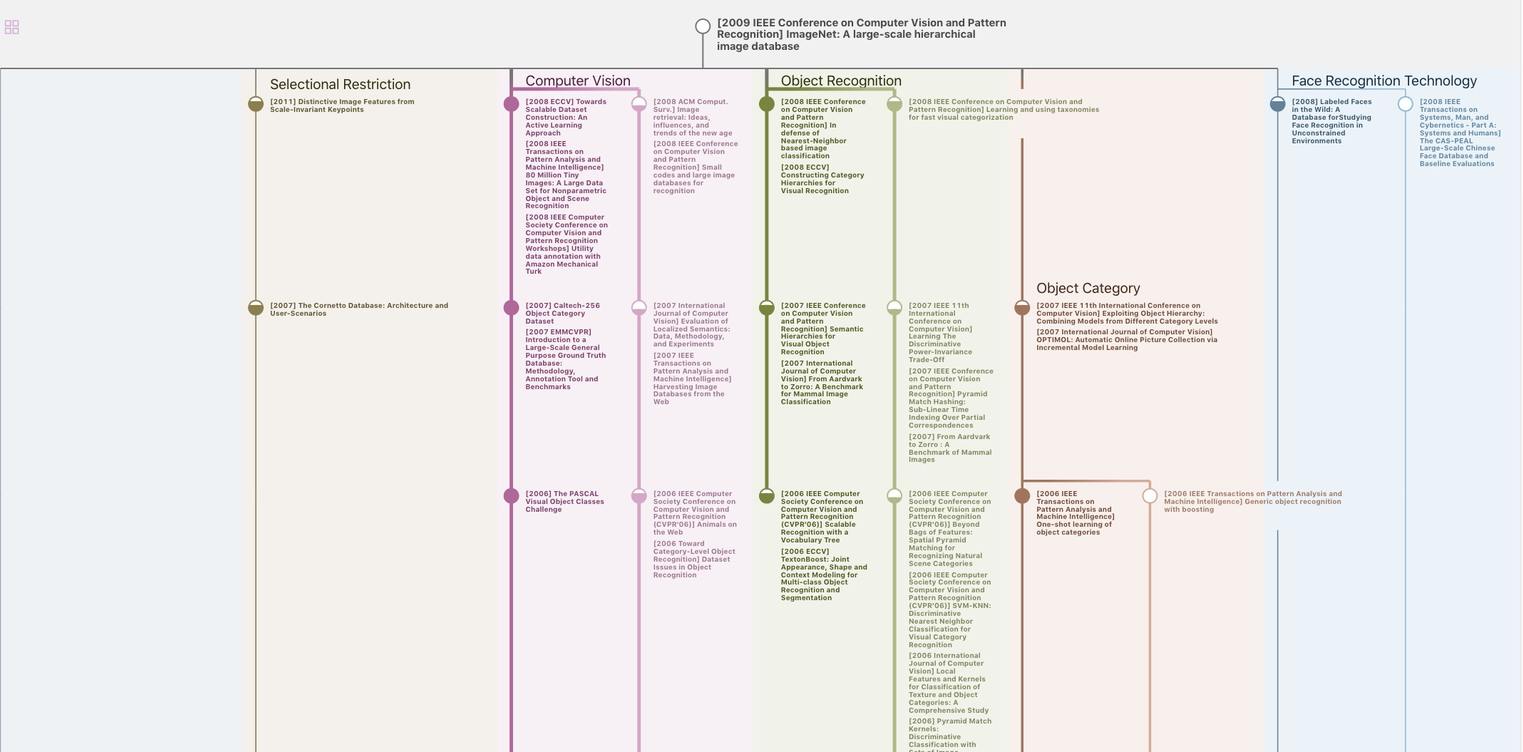
生成溯源树,研究论文发展脉络
Chat Paper
正在生成论文摘要