Spatio-Temporal Action Instance Segmentation and Localisation
user-5d8054e8530c708f9920ccce(2020)
摘要
Current state-of-the-art human action recognition is focused on the classification of temporally trimmed videos in which only one action occurs per frame. In this work we address the problem of action localisation and instance segmentation in which multiple concurrent actions of the same class may be segmented out of an image sequence. We cast the action tube extraction as an energy maximisation problem in which configurations of region proposals in each frame are assigned a cost and the best action tubes are selected via two passes of dynamic programming. One pass associates region proposals in space and time for each action category, and another pass is used to solve for the tube’s temporal extent and to enforce a smooth label sequence through the video. In addition, by taking advantage of recent work on action foreground-background segmentation, we are able to associate each tube with class-specific segmentations. We demonstrate the performance of our algorithm on the challenging LIRIS-HARL dataset and achieve a new state-of-the-art result which is 14.3 times better than previous methods.
更多查看译文
关键词
Segmentation,Dynamic programming,Computer vision,Computer science,Spacetime,Action recognition,Artificial intelligence,Image sequence,One pass
AI 理解论文
溯源树
样例
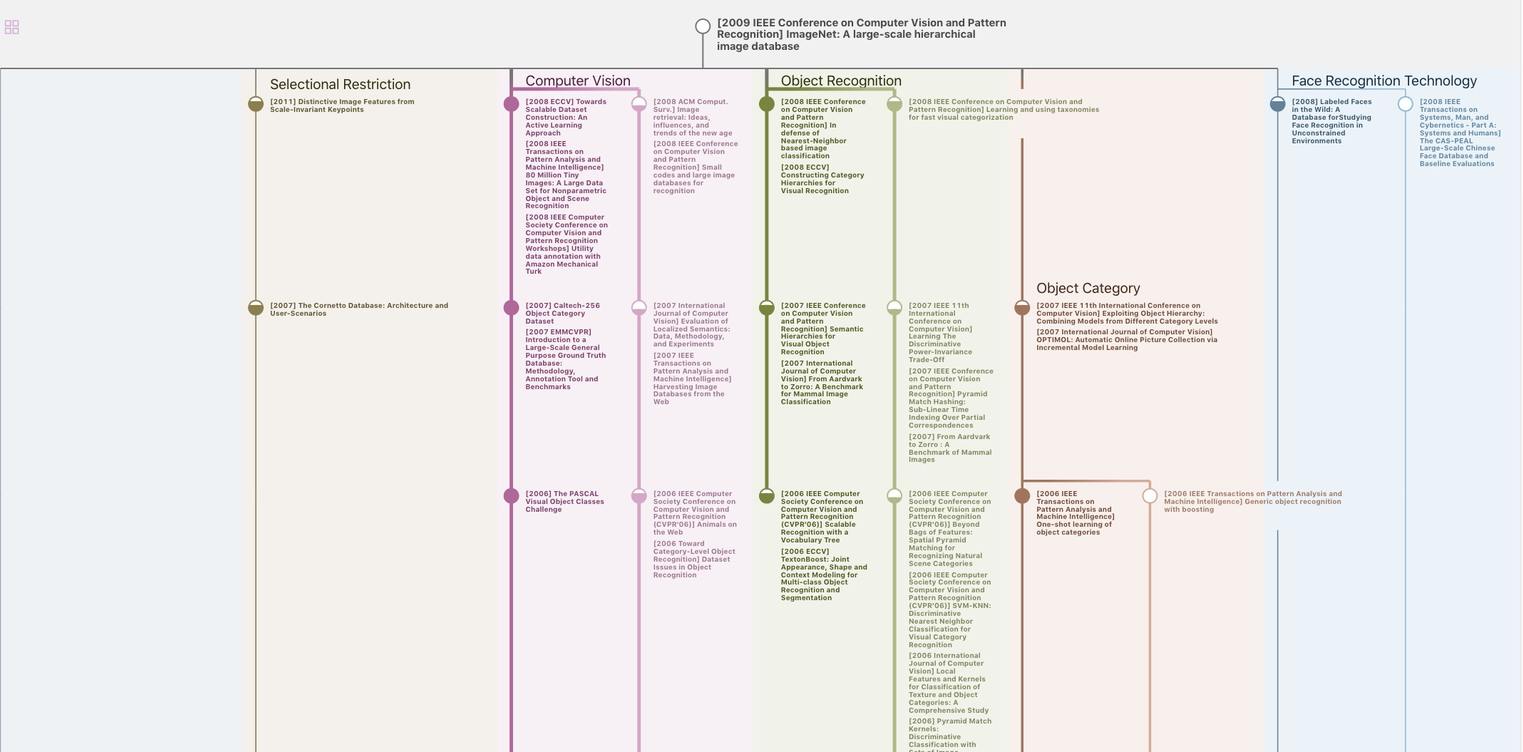
生成溯源树,研究论文发展脉络
Chat Paper
正在生成论文摘要