Machine Learning for Predictive Deployment of UAVs with Rate Splitting Multiple Access
2020 IEEE Globecom Workshops (GC Wkshps(2020)
摘要
In this paper, an unmanned aerial vehicles (UAVs) deployment framework based on machine learning is studied. UAVs are deployed as flying base stations (BSs) to offload heavy traffic from ground BSs. A method of backpropagation neuron network (BP) algorithm is used to predict the future cellular traffic. According to the cellular traffic spatial distribution, a KEG algorithm, which is a joint K-means and expectation maximization (EM) algorithm based on Gaussian mixture model (GMM), is proposed for determining each UAV's service area. The UAV locations are optimized to minimize transmit power in their service area. Three multi-access techniques are compared to minimize the total uplink transmit power. Simulation results show that the proposed method can reduce up to 24% of the total power consumption compared to the conventional method without traffic prediction. Besides, rate splitting multiple access (RSMA) has the lower required transmit power compared to frequency domain multiple access (FDMA) and time domain multiple access (TDMA).
更多查看译文
关键词
UAV Deployment,BP,K-means,EM,GMM,RSMA
AI 理解论文
溯源树
样例
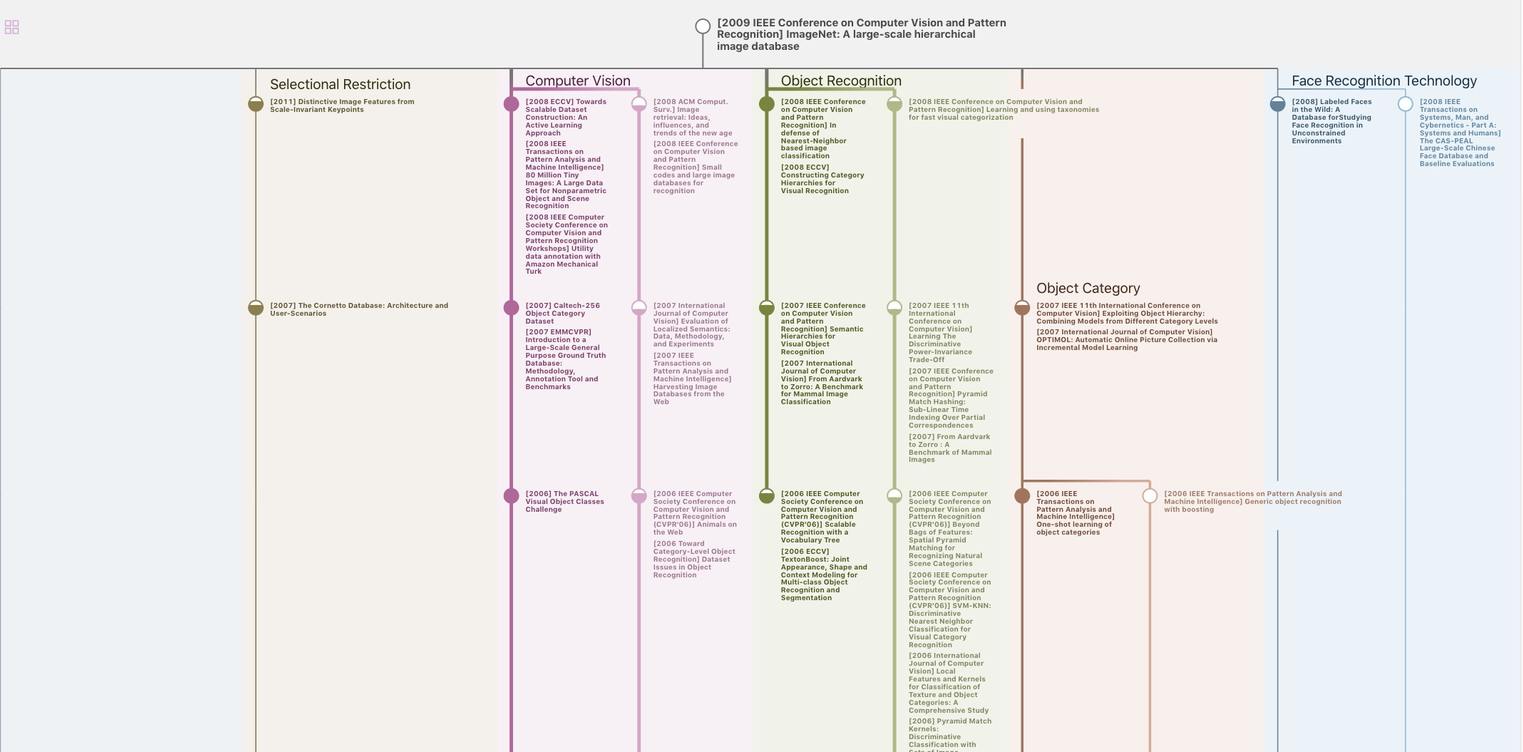
生成溯源树,研究论文发展脉络
Chat Paper
正在生成论文摘要