Factorized Binary Search: change point detection in the network structure of multivariate high-dimensional time series
arxiv(2021)
摘要
Functional magnetic resonance imaging (fMRI) time series data presents a
unique opportunity to understand the behavior of temporal brain connectivity,
and models that uncover the complex dynamic workings of this organ are of keen
interest in neuroscience. We are motivated to develop accurate change point
detection and network estimation techniques for high-dimensional whole-brain
fMRI data. To this end, we introduce factorized binary search (FaBiSearch), a
novel change point detection method in the network structure of multivariate
high-dimensional time series in order to understand the large-scale
characterizations and dynamics of the brain. FaBiSearch employs non-negative
matrix factorization, an unsupervised dimension reduction technique, and a new
binary search algorithm to identify multiple change points. In addition, we
propose a new method for network estimation for data between change points. We
seek to understand the dynamic mechanism of the brain, particularly for two
fMRI data sets. The first is a resting-state fMRI experiment, where subjects
are scanned over three visits. The second is a task-based fMRI experiment,
where subjects read Chapter 9 of Harry Potter and the Sorcerer's Stone. For the
resting-state data set, we examine the test-retest behavior of dynamic
functional connectivity, while for the task-based data set, we explore network
dynamics during the reading and whether change points across subjects coincide
with key plot twists in the story. Further, we identify hub nodes in the brain
network and examine their dynamic behavior. Finally, we make all the methods
discussed available in the R package fabisearch on CRAN.
更多查看译文
AI 理解论文
溯源树
样例
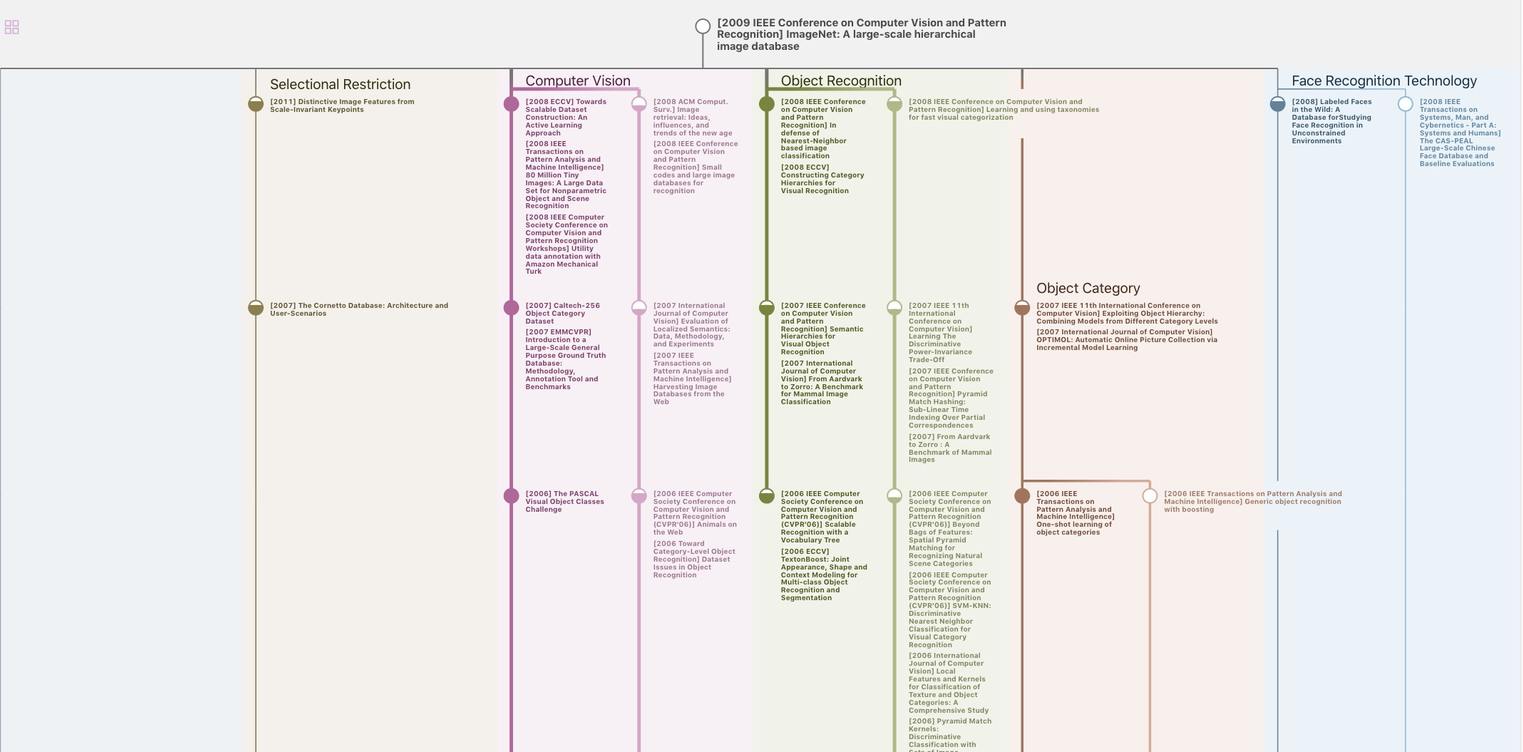
生成溯源树,研究论文发展脉络
Chat Paper
正在生成论文摘要