Indirect Estimation Of Vertical Ground Reaction Force From A Body-Mounted Ins/Gps Using Machine Learning
SENSORS(2021)
摘要
Vertical ground reaction force (vGRF) can be measured by force plates or instrumented treadmills, but their application is limited to indoor environments. Insoles remove this restriction but suffer from low durability (several hundred hours). Therefore, interest in the indirect estimation of vGRF using inertial measurement units and machine learning techniques has increased. This paper presents a methodology for indirectly estimating vGRF and other features used in gait analysis from measurements of a wearable GPS-aided inertial navigation system (INS/GPS) device. A set of 27 features was extracted from the INS/GPS data. Feature analysis showed that six of these features suffice to provide precise estimates of 11 different gait parameters. Bagged ensembles of regression trees were then trained and used for predicting gait parameters for a dataset from the test subject from whom the training data were collected and for a dataset from a subject for whom no training data were available. The prediction accuracies for the latter were significantly worse than for the first subject but still sufficiently good. K-nearest neighbor (KNN) and long short-term memory (LSTM) neural networks were then used for predicting vGRF and ground contact times. The KNN yielded a lower normalized root mean square error than the neural network for vGRF predictions but cannot detect new patterns in force curves.
更多查看译文
关键词
gait analysis, ground reaction force, ground contact time, INS, GPS, machine learning, deep neural network
AI 理解论文
溯源树
样例
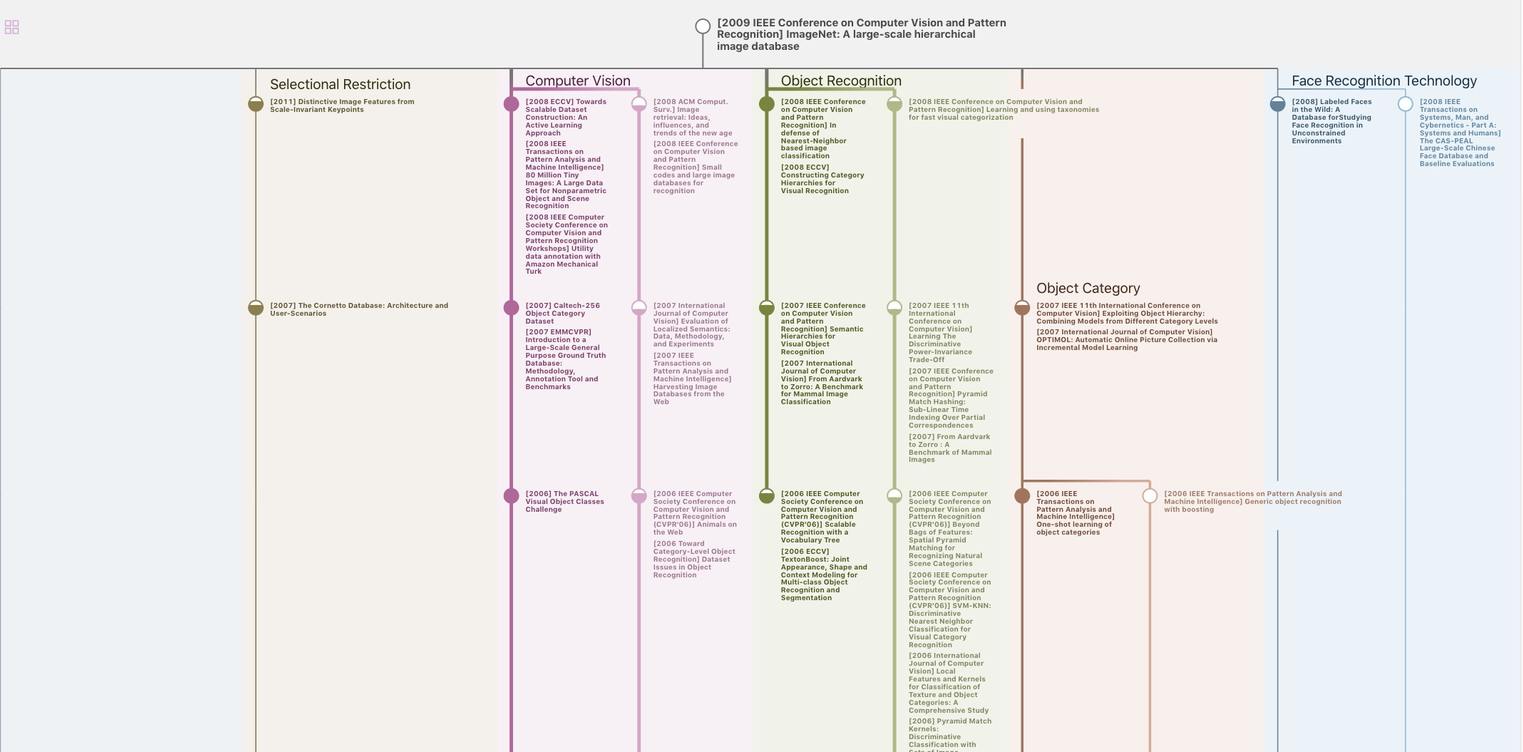
生成溯源树,研究论文发展脉络
Chat Paper
正在生成论文摘要