Masks and distancing during COVID-19: a causal framework for imputing value to public-health interventions.
Scientific reports(2021)
摘要
During the COVID-19 pandemic, the scientific community developed predictive models to evaluate potential governmental interventions. However, the analysis of the effects these interventions had is less advanced. Here, we propose a data-driven framework to assess these effects retrospectively. We use a regularized regression to find a parsimonious model that fits the data with the least changes in the [Formula: see text] parameter. Then, we postulate each jump in [Formula: see text] as the effect of an intervention. Following the do-operator prescriptions, we simulate the counterfactual case by forcing [Formula: see text] to stay at the pre-jump value. We then attribute a value to the intervention from the difference between true evolution and simulated counterfactual. We show that the recommendation to use facemasks for all activities would reduce the number of cases by 200,000 ([Formula: see text] CI 190,000-210,000) in Connecticut, Massachusetts, and New York State. The framework presented here might be used in any case where cause and effects are sparse in time.
更多查看译文
AI 理解论文
溯源树
样例
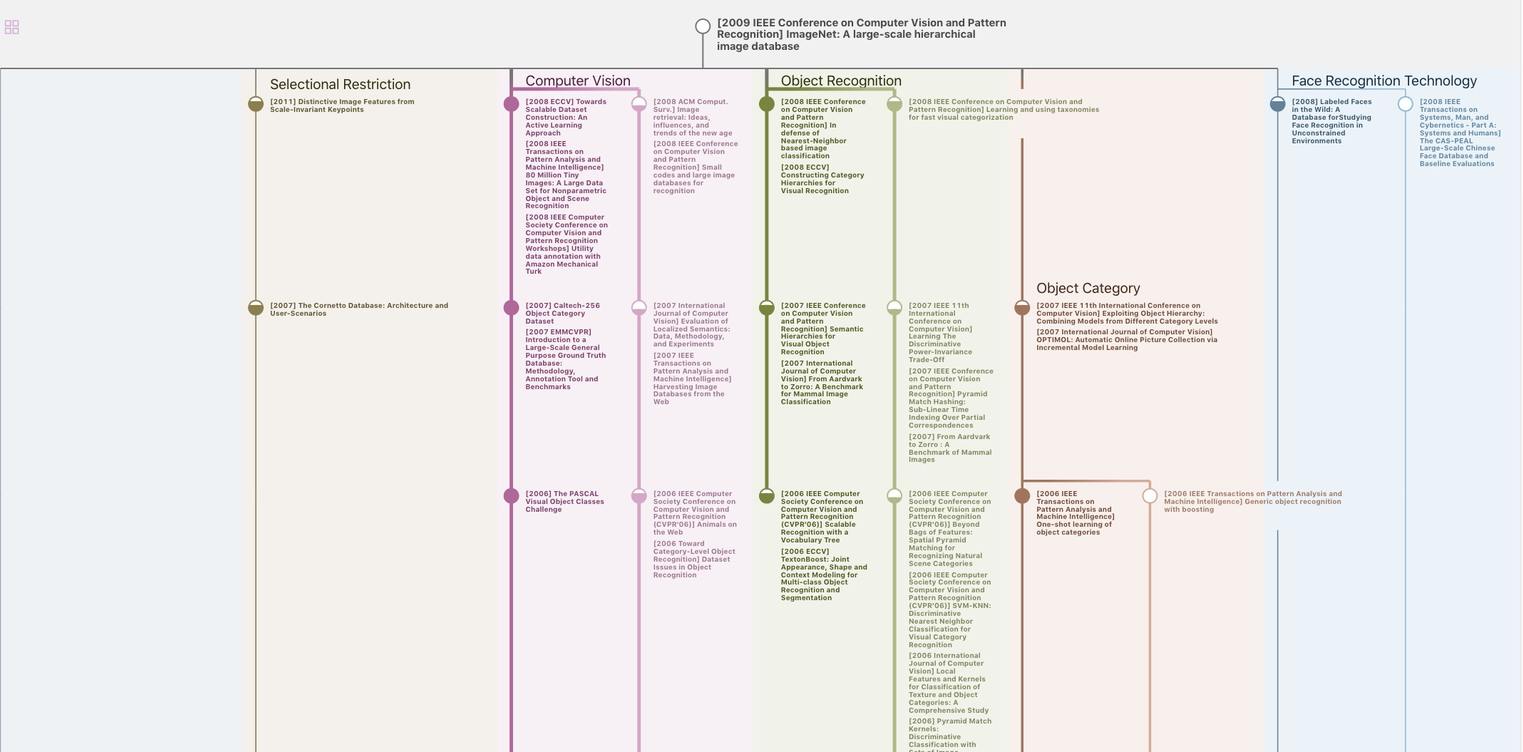
生成溯源树,研究论文发展脉络
Chat Paper
正在生成论文摘要