ERROR-DRIVEN FIXED-BUDGET ASR PERSONALIZATION FOR ACCENTED SPEAKERS
2021 IEEE INTERNATIONAL CONFERENCE ON ACOUSTICS, SPEECH AND SIGNAL PROCESSING (ICASSP 2021)(2021)
Abstract
We consider the task of personalizing ASR models while being constrained by a fixed budget on recording speaker specific utterances. Given a speaker and an ASR model, we propose a method of identifying sentences for which the speaker's utterances are likely to be harder for the given ASR model to recognize. We assume a tiny amount of speaker-specific data to learn phoneme-level error models which help us select such sentences. We show that speaker's utterances on the sentences selected using our error model indeed have larger error rates when compared to speaker's utterances on randomly selected sentences. We find that fine-tuning the ASR model on the sentence utterances selected with the help of error models yield higher WER improvements in comparison to fine-tuning on an equal number of randomly selected sentence utterances. Thus, our method provides an efficient way of collecting speaker utterances under budget constraints for personalizing ASR models.
MoreTranslated text
Key words
Data selection, Personalization, Accent-adaptation, Error detection, Speaker-adaptation
AI Read Science
Must-Reading Tree
Example
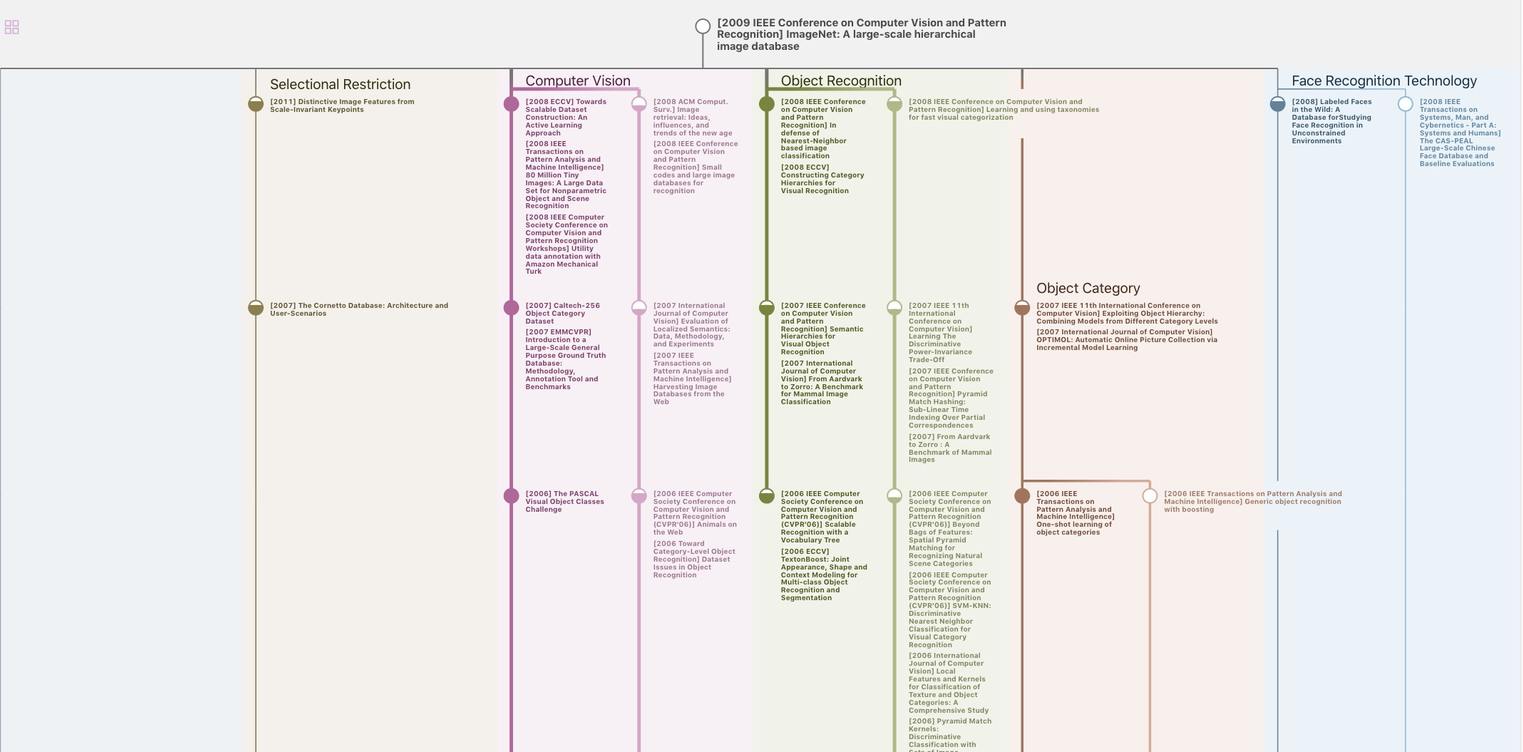
Generate MRT to find the research sequence of this paper
Chat Paper
Summary is being generated by the instructions you defined