LevelUp: A thin-cloud approach to game livestreaming
user-5f8cf7e04c775ec6fa691c92(2020)
摘要
Game livestreaming is hugely popular and growing. Each month, Twitch hosts over two million unique broadcasters with a collective audience of 140 million unique viewers. Despite its success, livestreaming services are costly to run. AWS and Azure both charge hundreds of dollars to encode 100 hours of multi-bitrate video, and potentially thousands each month to transfer the video data of one gamer to a relatively small audience.In this work, we demonstrate that mobile edge devices are ready to play a more central role in multi-bitrate livestreaming. In particular, we explore a new strategy for game livestreaming that we call a thin-cloud approach. Under a thin-cloud approach, livestreaming services rely on commodity web infrastructure to store and distribute video content and leverage hardware acceleration on edge devices to transcode video and boost the video quality of low-bitrate streams. We have built a prototype system called LevelUp that embodies the thin-cloud approach, and using our prototype we demonstrate that mobile hardware acceleration can support real-time video transcoding and significantly boost the quality of low-bitrate video through a machine-learning technique called super resolution. We show that super-resolution can improve the visual quality of low-resolution game streams by up to 88% while requiring approximately half the bandwidth of higher-bitrate streams. Finally, energy experiments show that LevelUp clients consume only 5% of their battery capacity watching 30 minutes of video.
更多查看译文
关键词
LevelUp,thin-cloud approach,game livestreaming,million unique broadcasters,collective audience,140 million unique viewers,multibitrate video,video data,relatively small audience,mobile edge devices,multibitrate livestreaming,leverage hardware acceleration,video quality,low-bitrate streams,mobile hardware acceleration,real-time video transcoding,low-bitrate video,low-resolution game streams,higher-bitrate streams,time 100.0 hour,time 30.0 min
AI 理解论文
溯源树
样例
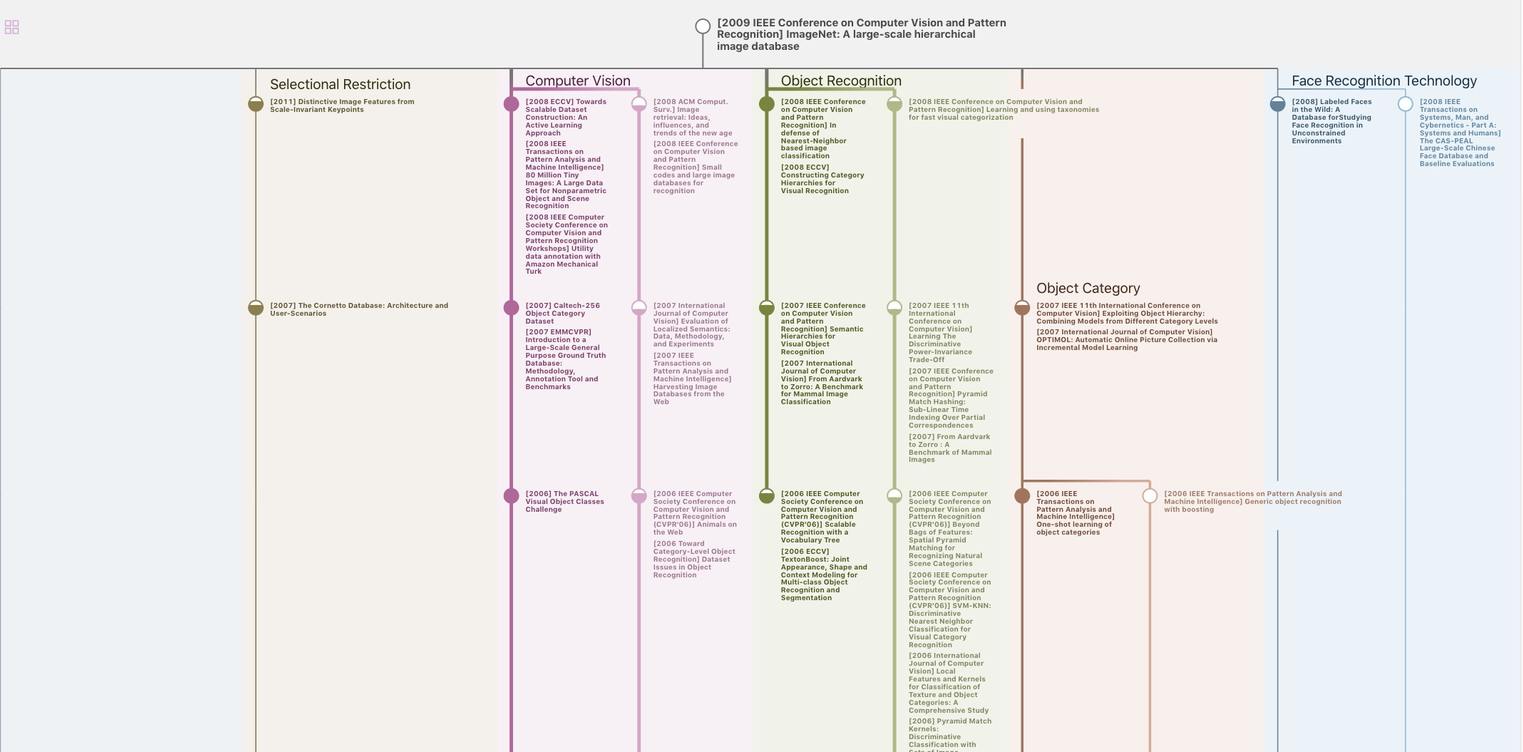
生成溯源树,研究论文发展脉络
Chat Paper
正在生成论文摘要