Machine Learning Identifies Girls With Central Precocious Puberty Based On Multisource Data
JAMIA OPEN(2020)
摘要
Objective: The study aimed to develop simplified diagnostic models for identifying girls with central precocious puberty (CPP), without the expensive and cumbersome gonadotropin-releasing hormone (GnRH) stimulation test, which is the gold standard for CPP diagnosis.Materials and methods: Female patients who had secondary sexual characteristics before 8 years old and had taken a GnRH analog (GnRHa) stimulation test at a medical center in Guangzhou, China were enrolled. Data from clinical visiting, laboratory tests, and medical image examinations were collected. We first extracted features from unstructured data such as clinical reports and medical images. Then, models based on each singlesource data or multisource data were developed with Extreme Gradient Boosting (XGBoost) classifier to classify patients as CPP or non-CPP.Results: The best performance achieved an area under the curve (AUC) of 0.88 and Youden index of 0.64 in the model based on multisource data. The performance of single-source models based on data from basal laboratory tests and the feature importance of each variable showed that the basal hormone test had the highest diagnostic value for a CPP diagnosis.Conclusion: We developed three simplified models that use easily accessed clinical data before the GnRH stimulation test to identify girls who are at high risk of CPP. These models are tailored to the needs of patients in different clinical settings. Machine learning technologies and multisource data fusion can help to make a better diagnosis than traditional methods.
更多查看译文
关键词
central precocious puberty, GnRH stimulation test, machine learning, multisource data
AI 理解论文
溯源树
样例
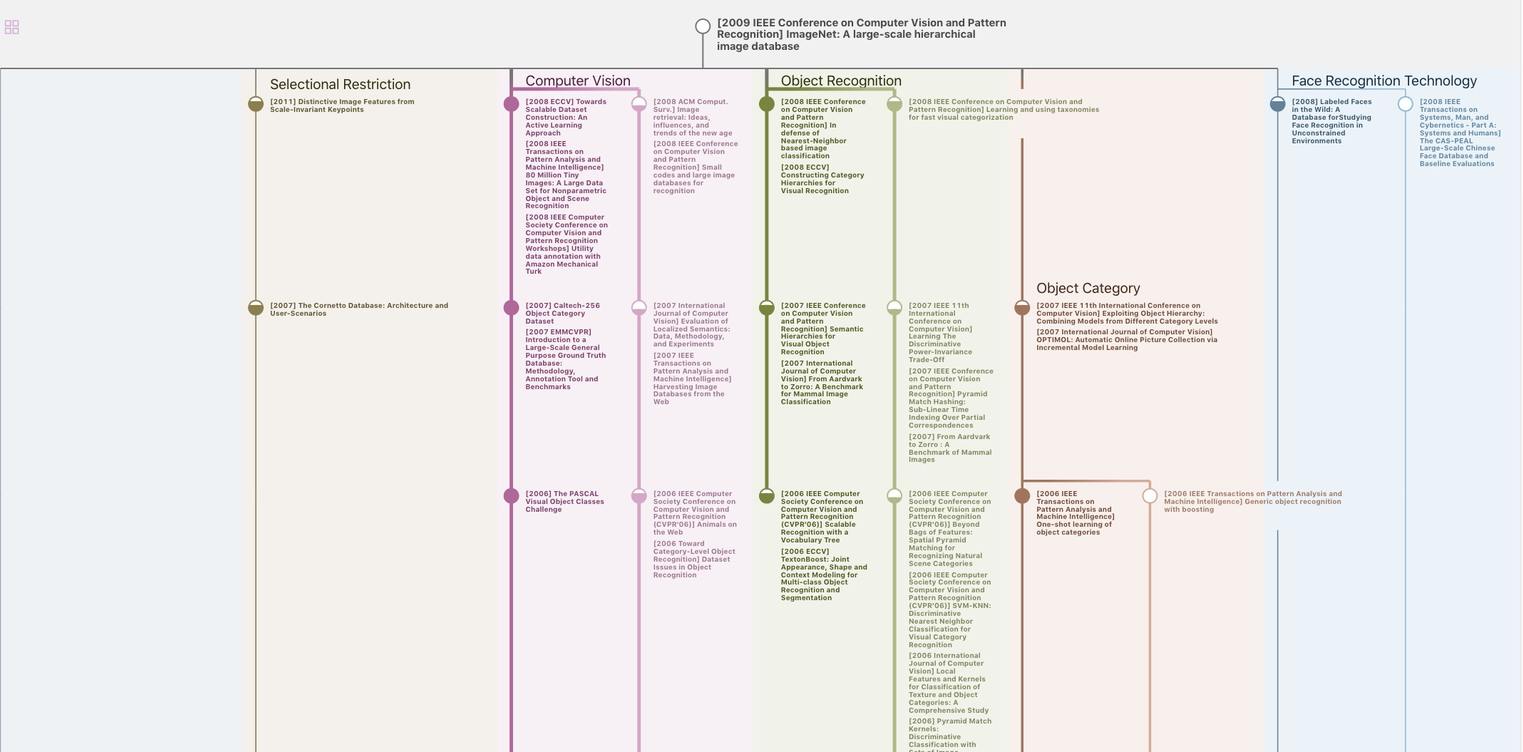
生成溯源树,研究论文发展脉络
Chat Paper
正在生成论文摘要