Prediction of Treatment Medicines With Dual Adaptive Sequential Networks
IEEE Transactions on Knowledge and Data Engineering(2022)
摘要
Predicting treatment medicines is a key task in many intelligent healthcare systems. Prediction of treatment medicines can assist doctors in making informed prescription decisions for patients according to their Electronic Health Records (EHRs). However, predicting treatment medicines is a challenging task due to the following reasons: (1) heterogeneous nature of EHR data that typically includes laboratory results, treatment records, disease conditions, and demographic information; (2) complex correlations among EHR sequences, including inter-correlations between sequences and temporal intra-correlations within each sequence; (3) temporal dynamics of these correlations changing with disease progression. In this paper, we predict treatment medicines for patients with dual adaptive sequential networks (DASNet). Specifically, DASNet is designed with three components. First, a decomposed adaptive long short-term memory network (DA-LSTM) is designed to capture the intra- and inter-correlations in multiple heterogeneous temporal sequences. Then, we develop an attentive meta learning network (AT-MetaNet) to learn dynamic weight parameters for DA-LSTM, thus enabling it to model various correlation structures. Finally, we employ an attentive fusion network (AT-FuNet) to incorporate historical information and collectively fuse representation embeddings of heterogeneous data to predict treatment medicines. Our results on the public MIMIC-III dataset covering 11 medical conditions demonstrate that the proposed end-to-end model can achieve the state-of-the-art prediction performance while providing clinically useful insights.
更多查看译文
关键词
Treatment prediction,sequential network,temporal correlations,electronic health records
AI 理解论文
溯源树
样例
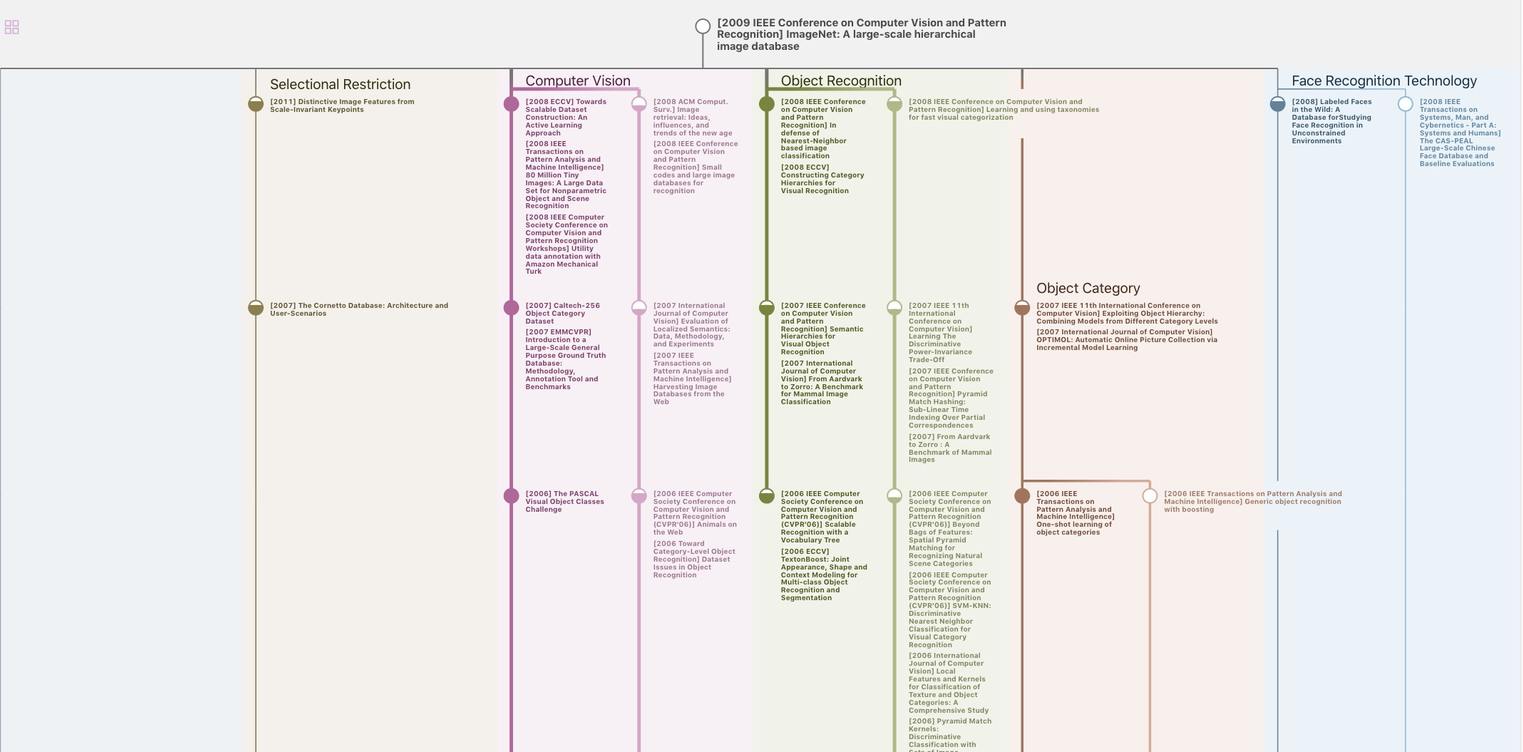
生成溯源树,研究论文发展脉络
Chat Paper
正在生成论文摘要