Standardised training system for specialists in China: a new opportunity and challenge for doctors.
Postgraduate medical journal(2022)
摘要
It is very important to train high-quality doctors for any country’s medical service system. As we can see, China is entering an ageing population era, facing the demand for health services of more than 1.3 billion people, which has brought a great challenge to the Chinese government and doctors. Following international practice, China is gradually improving the key steps to establish a national doctor training system. As reported, the standardised residency training (SRT) has been widely promoted in China, and has become a compulsory training for all medical graduates.1\n\nAlthough there is still room for improvement in SRT, it has already played a positive role. Therefore, it is time to think about where the future medical education of young doctors in China will go after they have completed SRT. The Chinese government has also realised that the ultimate success of their ambitious health reform depends on the well-educated and constantly trained health professionals. So a brand-new training model named 5 + 3 + X has been introduced to produce doctors with clinical, professional, humanistic and ethical abilities, which are crucial to the quality of clinical services.2 In detail, the 5 + 3 + X model requires a doctor to receive 5 years of undergraduate medical education and 3 years of SRT, and then receive 2–4 (X) years of standardised specialist training (SST) according to the requirements of different specialties. The plan of the Chinese government is to initially implement SST nationwide in 2020.2 Based on the SRT, SST aims at training clinicians who can independently engage in specialised diagnosis and treatment. Trainees applying for SST are required to be doctors who have obtained the certificate of SRT and intend to engage in clinical work of a certain specialty, or postgraduate students with a medical doctorate. In order to …
更多查看译文
关键词
medical education & training
AI 理解论文
溯源树
样例
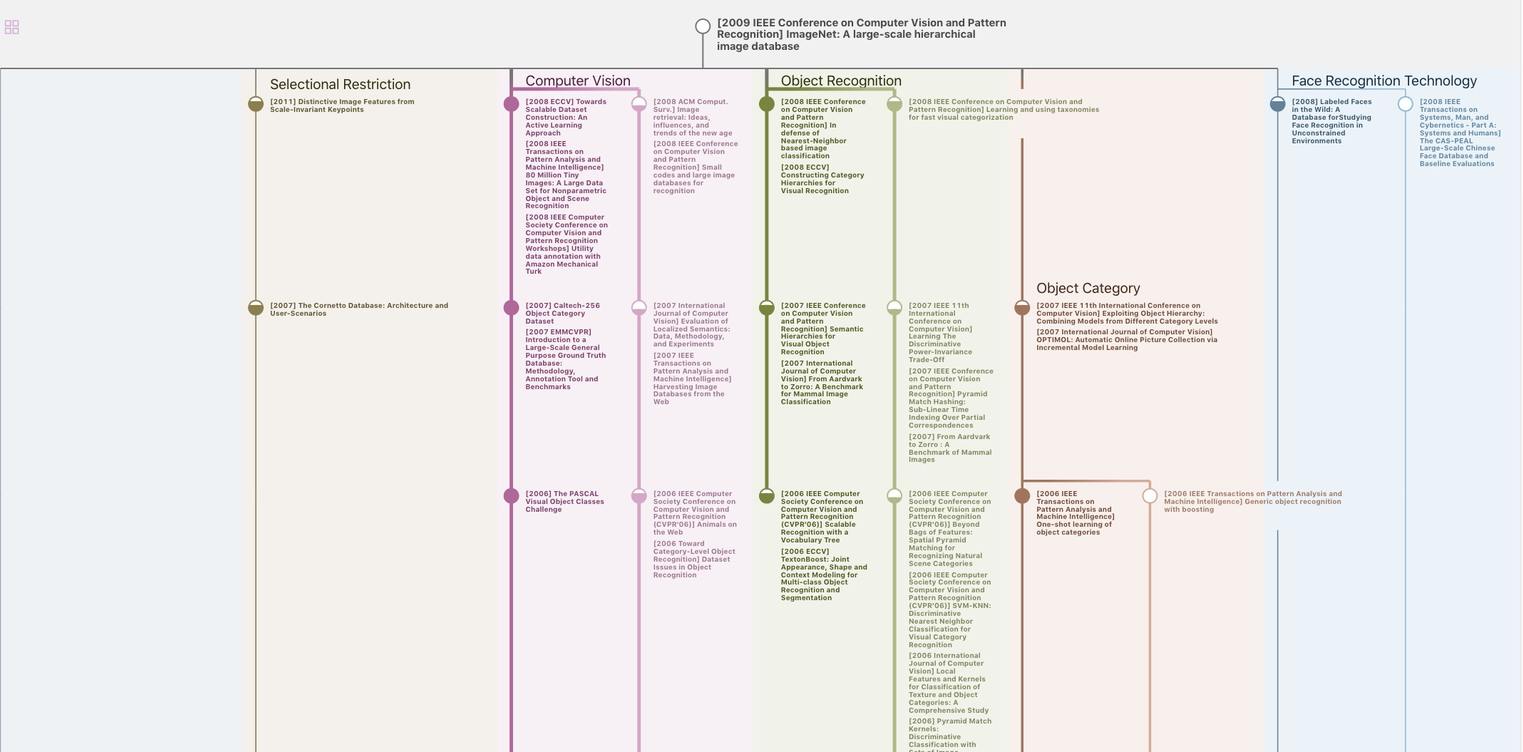
生成溯源树,研究论文发展脉络
Chat Paper
正在生成论文摘要