Pattern Recognition In Distributed Fiber-Optic Acoustic Sensor Using An Intensity And Phase Stacked Convolutional Neural Network With Data Augmentation
OPTICS EXPRESS(2021)
摘要
Distributed acoustic sensors (DASs) have the capability of registering faint vibrations with high spatial resolution along the sensing fiber. Advanced algorithms are important for DAS in many applications since they can help extract and classify the unique signatures of different types of vibration events. Deep convolutional neural networks (CNNs), which have powerful spectro-temporal feature learning capability, are well suited for event classification in DAS. Generally, these data-driven methods are highly dependent on the availability of large quantities of training data for learning a mapping from input to output. In this work, to fully utilize the collected information and maximize the power of CNNs, we propose a method to enlarge the useful dataset for CNNs from two aspects. First, we propose an intensity and phase stacked CNN (IP-CNN) to utilize both the intensity and phase information from a DAS with coherent detection. Second, we propose to use data augmentation to further increase the training dataset size. The influence of different data augmentation methods on the performance of the proposed CNN architecture is thoroughly investigated. The experimental results show that the proposed 1P-CNN with data augmentation produces a classification accuracy of 88.2% on our DAS dataset with lkm sensing length. This indicates that the usage of both intensity and phase information together with the enlarged training dataset after data augmentation can greatly improve the classification accuracy, which is useful for DAS pattern recognition in real applications. (C) 2021 Optical Society of America under the terms of the OSA Open Access Publishing Agreement
更多查看译文
AI 理解论文
溯源树
样例
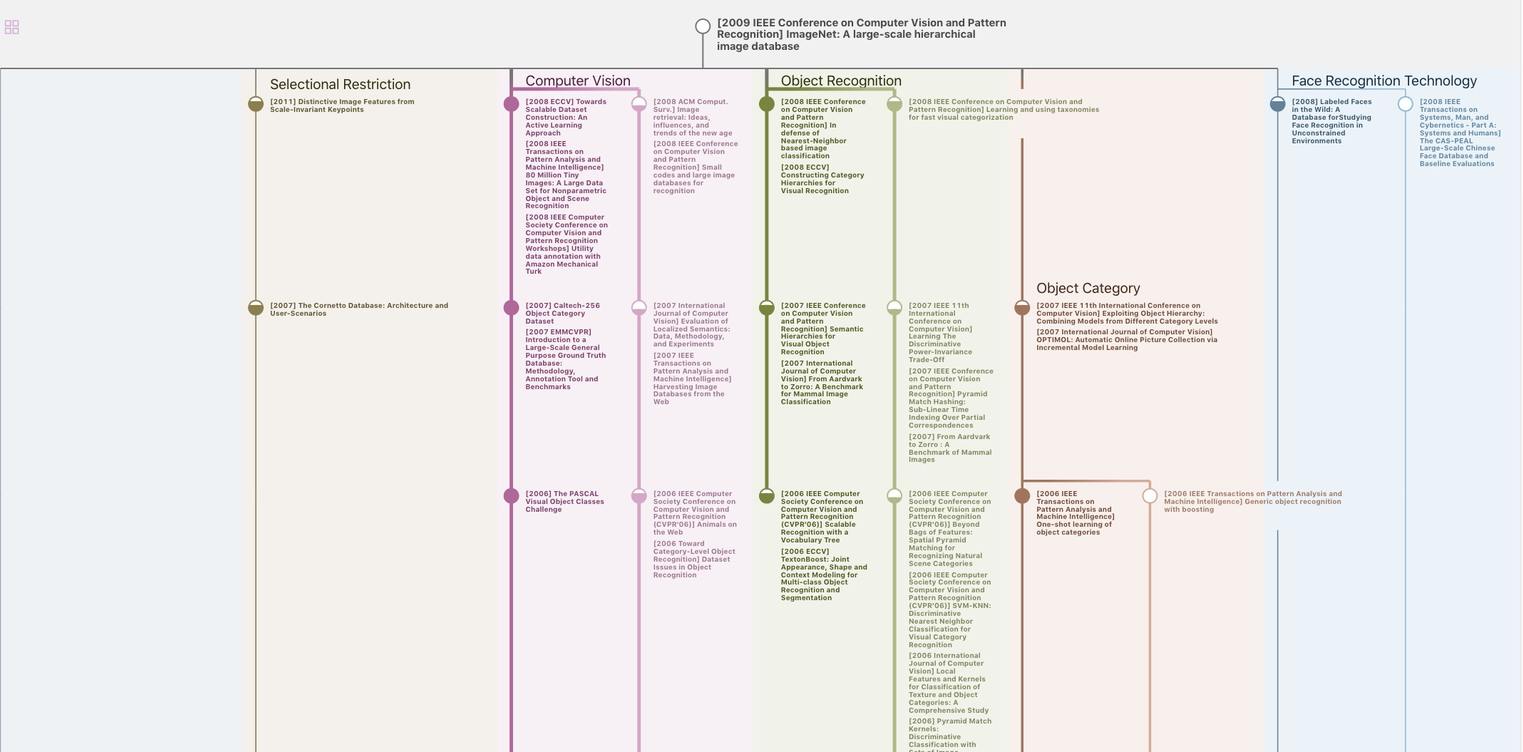
生成溯源树,研究论文发展脉络
Chat Paper
正在生成论文摘要