Cancer miRNA biomarkers classification using a new representation algorithm and evolutionary deep learning
SOFT COMPUTING(2020)
摘要
The diagnosis of cancer is presently undergoing a change of paradigm for the diagnostic panel using molecular biomarkers. MicroRNA (miRNA) is one of the most important genomic datasets presenting the genome sequences. Since several studies have shown the relationship between miRNAs and cancers, data mining and machine learning methods can be incorporated to extract a large amount of knowledge from cancer genomic datasets. However, previous research works on the identification of cancers from miRNAs have made it possible to diagnose cancer, and the accuracy of some classes is not quite satisfactory. Therefore, this research is aimed at promoting a super-class (meta-label) approach and deep learning in a three-phase method to diagnose cancers from miRNAs. The steps in the first phase of the proposed method, named Representation learning, are partitioning data into super-classes, meta-data creation and super-classes classification. This phase helps data to be split into some subsets to improve classification accuracy. In other words, the first phase groups labels based on the separability of classes into a meta-label, and then a multi-label learner is built to predict these meta-labels. In the second phase, a feature selection to reduce the dimensions of the problem is applied to each super-class to help to focus the attention of an induction algorithm in those features that are more important to predict the target concept. In the third phase of the proposed method, an evolutionary deep neural network for the classification of labels in each super-class is performed. The last two phases are done separately for each subset in which five super-classes and subsequently five deep neural networks are trained. The experimental results reveal that the proposed method achieved more efficient results than 19 recent machine learning methods. Despite the fact that evaluating the dataset which consists of 29 types of cancers provides a more complicated situation for the convolutional neural network to be learned, the performance of the method is noticeably better than other existing methods. The other success which can be considered here is a significant reduction in running time comparing to other methods.
更多查看译文
关键词
MicroRNA,Meta-label,Super-class,Intelligent reasoning,Feature selection,Optimized convolutional neural network
AI 理解论文
溯源树
样例
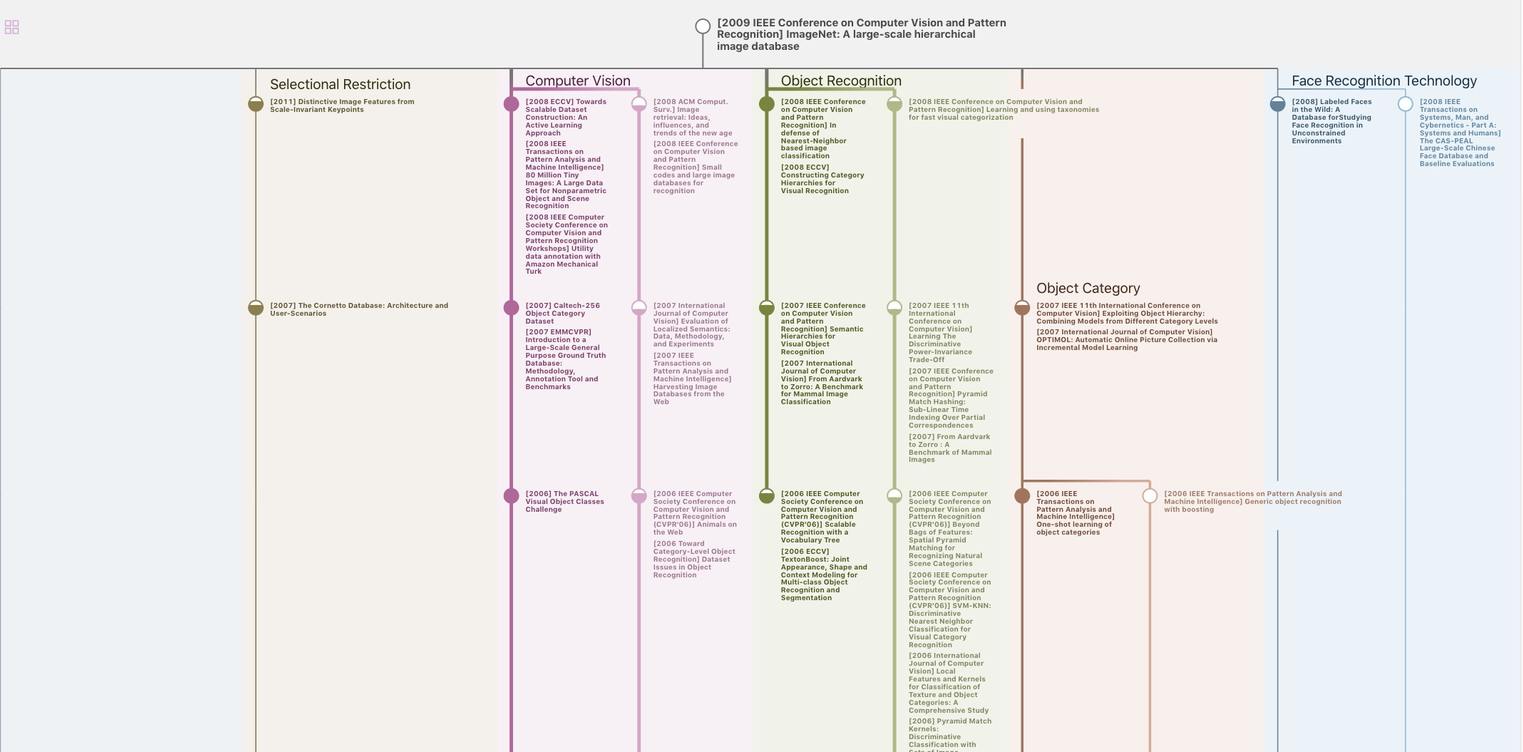
生成溯源树,研究论文发展脉络
Chat Paper
正在生成论文摘要